A future focus: Science, technology and innovation
Drawing on the experience of our panel, in this section we highlight select examples that could shift how we fish commercially to reduce the stress it causes within the marine environment. We recognise that research and innovation relating to fisheries and the marine environment is expensive – the feasibility and prioritisation regarding implementation falls beyond this report. Not all of these ideas are will be implemented at scale by 2040, but we take a deliberate future focus to highlight a raft of changes.
Contents
- Introduction
- How we respond to changing fisheries
- How we fish
- Knowing how fish behave is essential for gear design of the future
- Future nets, trawls and dredges could be more selective with reduced impacts
- Innovations could increase efficiencies and reduce harm from line fishing
- Traps and pots could be redesigned to eliminate entanglements and gear loss
- Gear add-ons will be essential to deter non-target species
- Technological advances in fish detection could reduce fuel costs and time at sea
- Challenges and opportunities with gear innovation
- How much we fish
- Computers, cameras and AI could revolutionise catch monitoring
- Technical and analytical advancements will help stock assessments
- Genetic technologies
- Biochemical technologies
- Acoustic technologies
- Innovations that expand ecosystem knowledge will add an extra dimension to fisheries management
- Underwater and surface cameras give a wider and sharper view of the ocean
- Environmental DNA (eDNA) can grow ecosystem knowledge
- Models can support ecosystem approaches to fisheries management
- Where and when we fish
- New tools can refine spatial and temporal knowledge or marine life to inform fisheries management
- Comprehensive models can inform predictions about populations and protected species
- Dynamic ocean management will help protect non-target species in real time
- Innovative tools can also be used to detect illegal fishing
- How we ensure a healthy ocean
- Using the whole fish to develop high-value by-products
- References and footnotes
Introduction
Over the past three decades, Aotearoa New Zealand’s fisheries management system has evolved somewhat to support more sustainable fishing and reduce some of the adverse effects of fishing. Data and science has helped to shine a light on parts of our fisheries to inform these improvements. However, our knowledge of ocean dynamics and ecosystems and how these influence our fish stocks remains poor. Without this knowledge, we will remain largely in the dark about what, where, when and how we can fish in order for the fishing to be sustainable.
With such a complex and inaccessible system as the ocean, action cannot wait for the perfect dataset, which is why we have recommended changes to the regulatory framework and the research system suggested in the section ‘Commercial fisheries in 2020’. In parallel, science and technological innovation will enable improvements in the sustainability of our commercial fishing sector on a longer timeframe. Innovation is not yet part of the Aotearoa New Zealand global fisheries brand, but it could be. There are many innovative ideas and scientific solutions to help address sustainability issues in the commercial fishing sector – some old and some new. In a challenging environment characterised by poor information, high infrastructure costs, and divergent and sometimes conflicting priorities, these ideas face many barriers to implementation. A policy and regulatory environment in which innovation can thrive and information can rapidly feed into more responsive management decisions on shorter timescales will be fundamental to achieving better outcomes for our fisheries and the marine environment more broadly in the long term.
There are many innovative ideas and scientific solutions to help address sustainability issues in the commercial fishing sector – some old and some new. In a challenging environment characterised by poor information, high infrastructure costs, and divergent and sometimes conflicting priorities, these ideas face many barriers to implementation.
The marine environment faces significant challenges in 2020 – it is under considerable stress from fishing, sedimentation, climate change, pollution and more (as discussed in ‘Challenges for the marine environment’). These challenges cannot be solved with science alone. A systems change is required that brings together a raft of solutions, some scientific but many relating to a culture change to improve relationships within, and management of, the marine environment. Despite science not providing all of the short-term answers, the application of a raft of scientific innovations may collectively provide improvements in the health of our fisheries and the marine environment. In this part of the report we focus on the tools that may help to achieve these desired improved outcomes. Some of these tools have been available for some time, but not widely adopted. Others are new and not yet ready for implementation. All have something to offer a vision of future fisheries management if prioritised within an overarching strategy for the ocean.
How we respond to changing fisheries
A big challenge associated with making commercial fishing more sustainable is managing fisheries that are ever-changing. Both the regulator and industry need extensive data and information about fisheries changes to inform faster and more effective decision making.
Some biological variations that occur are known and expected, while others are not. Variations in stock numbers may be evident through fluctuations in recruitment that occur years to decades apart, but the phenomena that causes the fluctuation may remain unknown. An example is hoki[1, 2] where western science has not explained a phenomenon acknowledged through mātauranga Māori.[3] Recognising biological variations in the natural world is essential to accommodate them in fisheries strategies and management and further basic research in this area would be beneficial.
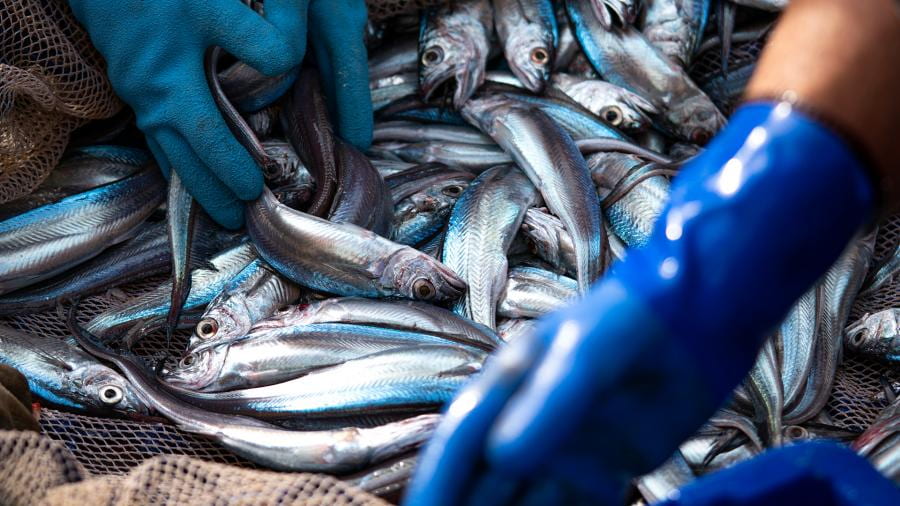
Hoki harvested during a research trawl. Image credit: Stu Mackay/NIWA.
Climate change, ocean acidity, marine heatwaves and other anthropogenic stressors amplify the need to be able to respond to changing fisheries. As discussed in the section ‘Climate change is a huge threat to our oceans’, climate change will drive many changes across fisheries by both disrupting ecosystems and moving species into different regions in response to changing temperatures and ocean circulation. Though a changing climate is already upon us, far more significant changes are expected to come. The climate changes to date have already highlighted the need for greater data collection and analysis to feed into more rapid and responsive decision making. The management system will need to be able to urgently respond to challenges such as shifting stocks, disrupted food webs, changes in fish productivity and recruitment patterns, and ocean acidification.
Climate changes to date have already highlighted the need for greater data collection and analysis to feed into more rapid and responsive decision making.
The marine environment and fisheries will continue to be further impacted by the other stressors outlined in ‘Challenges for the marine environment’, including sedimentation, plastic pollution and invasive species, with their cumulative effects contributing to ongoing changes in fisheries that will be felt by the commercial sector.
Changes in our fisheries require management that is flexible and adaptable to changing situations, at pace. It is essential that Aotearoa New Zealand’s fisheries management system accelerates rather than encumbers adaptation to a changing environment.
The resulting changes in fisheries require management that is flexible and adaptable to changing situations, at pace. It is essential that Aotearoa New Zealand’s fisheries management system accelerates rather than encumbers adaptation to a changing environment. To make our fisheries management system responsive to these inevitable changes we need to draw on innovative ways of collecting and managing data to make it more widespread and efficient. This data needs to be rapidly turned into useful information and be acted on without delay. Keeping pace with the increasing volumes of data and extracting knowledge from it will be a challenge, but we can draw on the emerging fields of artificial intelligence (AI) and machine learning to do so.
While data is fundamental to being able to make informed decisions, a lack of data should not be a roadblock to making decisions. There will still be times where there is a need to make decisions without a complete evidence and the system should be able to account for uncertainty while we endeavour to fill in knowledge gaps.
There will still be times where there is a need to make decisions without a complete evidence base and the system should be able to account for uncertainty while we endeavour to fill in knowledge gaps.
Changing fisheries demand nimble and responsive decision making
In a changing environment, fisheries management needs to be able to respond to variations so that an accurate picture of stock and ecosystem health forms the basis of decisions. Many stakeholders we engaged with during this project emphasised the need for a nimbler fisheries management system, highlighting that the TAC system (described in ‘Setting catch limits and allocating catch allowance’) is not sufficiently responsive.
Detecting and responding to changes in the distribution and productivity of species requires both good monitoring and a responsive fisheries management system. The system needs to be able to respond quickly to changes in abundance, as well as situations where there is new need for catch entitlement (e.g. if the bycatch of a species increases significantly due to shifts in distribution or a population boom) or where catch entitlement is ineffectual (e.g. because the species is no longer present). Enabling early signals of changing stock structure or presence to be rapidly investigated to quickly inform decisions around TAC in that region or management area would support this, though the success partly relies on having a solid foundation of species knowledge.
Information and knowledge from a range of different sources could also be drawn on to trigger a stock assessment, including locally held knowledge from fishers and the mātauranga held by local iwi (mostly relating to our inshore fisheries). The effort to evaluate the information and develop responsive management is likely of most benefit to short-lived species, whose abundance fluctuates largely year-to-year.
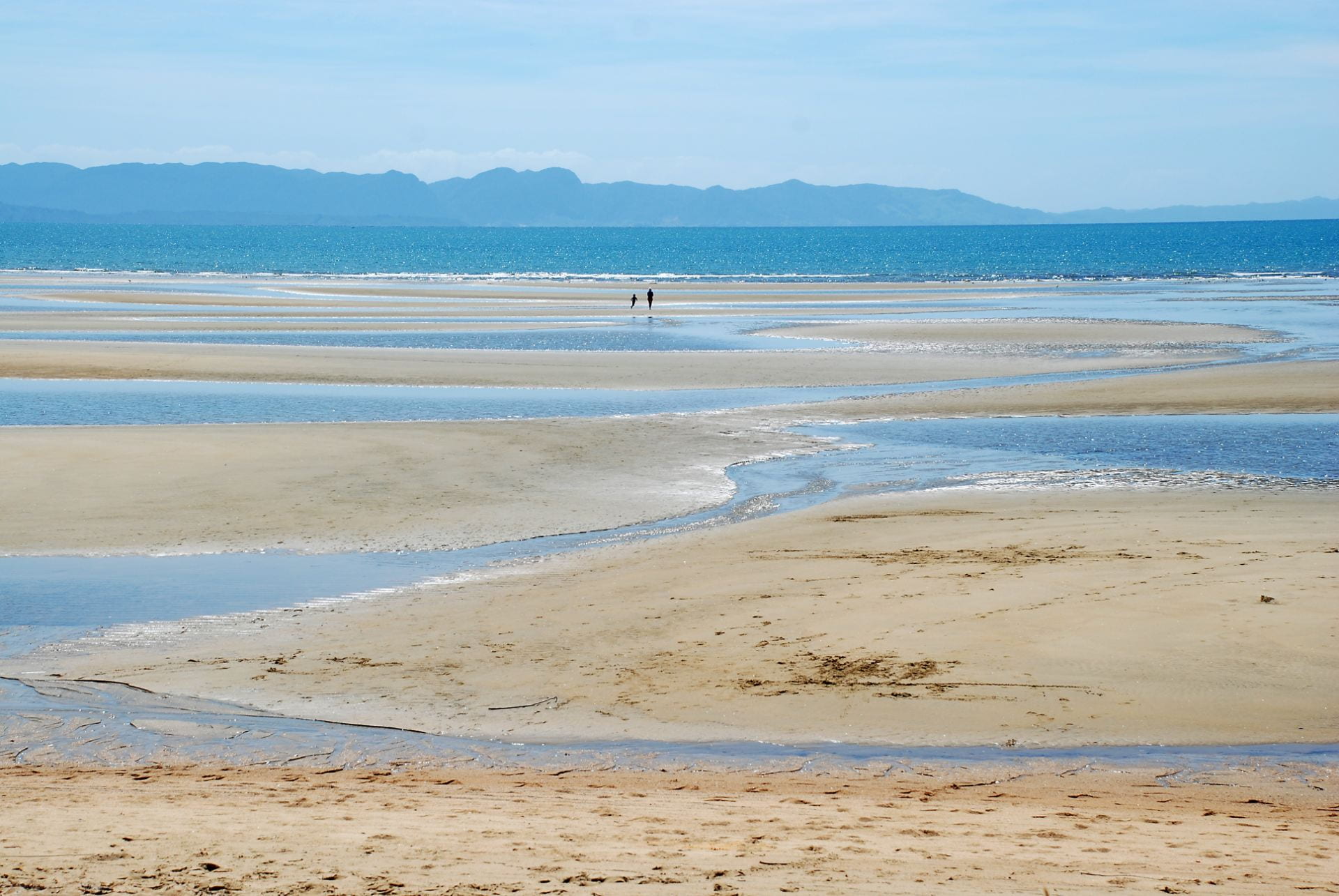
The once highly productive scallop fisheries of Golden Bay have diminished substantially. Image credit: Tata Bay, Geof Wilson/Flickr (CC BY-NC-ND 2.0).
Information and knowledge from a range of different sources could be drawn on to trigger a stock assessment, including locally held knowledge from fishers and the mātauranga held by local iwi.
Taking a regional approach to management at an appropriate scale for the fishery will also enable greater flexibility in decision making and more responsive management. The issues that need to be addressed may be unique to an area and require a holistic local approach to tackle a range of stressors that are causing changes to fisheries. Regional approaches to fisheries management are more resource intensive but can provide greater input and satisfaction for local communities (within the context of broader fisheries management). These are already showing promise throughout Aotearoa New Zealand (see case study: Fiordland created a novel model for managing the marine area and case study: Te Korowai o te tai ō Marokura in Kaikōura shows how regional responsibility can streamline fisheries management), and could be built on by making sure the process is able to respond to new data and knowledge in a rapid fashion. Locally held knowledge will play a critical role in more flexible decision making, specifically for inshore fisheries. Once time has been spent establishing local involvement in marine management, combining place-based evidence at an appropriate scale with a management framework that is fast and responsive will help to provide optimum management for each unique scenario.
Combining place-based evidence at an appropriate scale with a management framework that is agile and responsive will help to provide optimum management for each unique scenario.
Existing management processes could be modified to sharpen the response capacity. The recent shift within the fisheries management system to use digital technology for EM provides a strong foundation on which to build a more responsive system. Information about harvested catch gleaned from these processes can be built into models and ongoing monitoring efforts to observe changes in near real time to support ‘moving management options’. The innovations highlighted in the sections ‘How much we fish’ and ‘Where and when we fish’ will also be critical components of a more responsive system. Faster decision making will also support consistency in the application of new software and technologies in the industry, with guidance from the regulator being received prior to purchasing decisions.
The recent shift within the fisheries management system to use digital technology for electronic monitoring provides a strong foundation on which to build a more responsive system.
Regulatory swiftness underpinned by a stronger evidence base to inform decisions will be the foundation of a responsive management system. Such a framework would enable researchers to more efficiently provide input into the decision-making process and identified risk indicators could ensure rapid action to protect ecosystem health. A far greater understanding of basic fisheries science and ecosystem dynamics is a prerequisite to enable this more responsive system, along with ecosystem indicators. This in turn will enable the ongoing and sustainable use of Aotearoa New Zealand’s fisheries for generations to come.
These considerations underpin our recommendations in Theme 4.
Data-driven knowledge is the cornerstone of effective and sustainable fisheries management
Improving how we collect, curate, use and share fisheries and marine science data is crucial to advance Aotearoa New Zealand’s fisheries management system and enable research to answer critical questions in the marine environment. In the sections ‘Commercial fishing has impacts on target species sustainability’ and ‘Fishing effort has wider ecosystem impacts’, we highlighted what we know and the gaps in our knowledge for Aotearoa New Zealand’s fish stocks and ecosystems.
As discussed in ‘Data transformation strategy’, Fisheries New Zealand is working to improve data collection, handling and storage; however, the marine data landscape in Aotearoa New Zealand is currently fragmented and often inaccessible. A useful step forward would be to aggregate existing datasets from within and outside government, determine data and knowledge gaps, and prioritise efforts to fill these gaps. Some data gaps urgently need to be filled using the methods widely used in fisheries science (i.e. stock assessments for priority stocks that are currently not assessed (see section ‘Data collection on target stocks and accessibility of this information’). Some other decisions rely on out-of-date data or would benefit from research to better understand the basic biology of the commercial fish species. Systems should be in place so that data is strategically collected to fill knowledge gaps, data is collected regularly, decisions are based on the present not the past, and full datasets are available for extrapolations to predict outcomes. Transparency of the data used in fisheries management is vital to the scientific scrutiny of the management decisions.
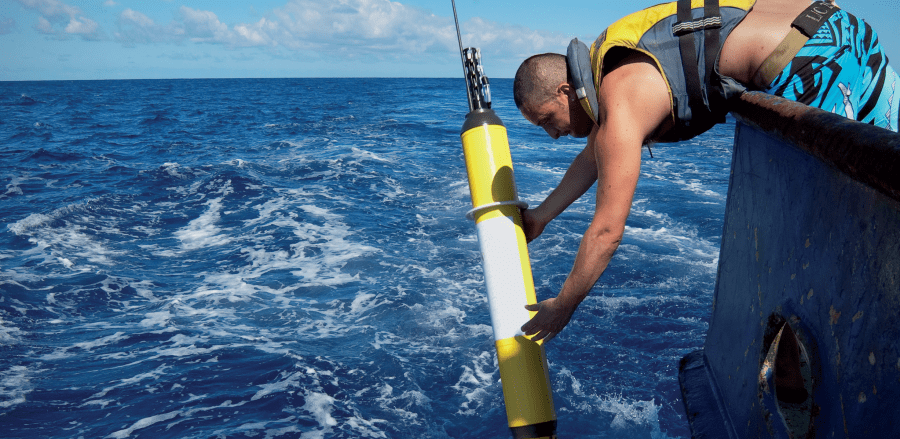
Deploying an Argo float, part of a worldwide network of ocean sensors collecting data. Image credit: Simon Wadsworth/NIWA.
Systems should be in place so that data is strategically collected to fill knowledge gaps, data is collected regularly, decisions are based on the present not the past, and full datasets are available for extrapolations to predict outcomes.
There are also areas where we are data rich and information poor – that is, the data is not being used to its full potential to extract knowledge. The use of electronic reporting primarily for compliance purposes is an example of this, where we have rich data that could be used more widely (see section ‘Electronic catch and position reporting is live’). Best practice examples where we have robust long-term data that informs decision making, such as in the Chatham Rise[4] (see case study: Chatham Rise is a unique fishery with consistent, long-term data), are what we should strive for in all other priority fisheries management regions throughout the country.
Data needs to be integrated in a way that it is easily accessible for decision makers, commercial fisheries, researchers and other stakeholders. Improving how we integrate and manage data, and opening up access so data is widely utilised, will help to turn data into information. The real value of the (long-term, time-stamped) data will then come from the interpretation and analyses of it, and the risk assessments and decision support tools that are driven by the data. The suggestions below can feed into the data transformation strategy that is currently underway within Fisheries New Zealand (introduced in ‘Regulator initiatives and data transformation’).
There are opportunities to collect data for fisheries in new ways
There are innovative approaches that can be applied to broaden how and when we collect data for management, research and monitoring. Collecting fisheries independent data is particularly important for validation and building trust in industry data. These data collection approaches can build on and complement the existing systems that mostly rely on fisheries scientists and catch monitoring.
- Draw on mātauranga. As is demonstrated in the ‘Te ao Māori’ section, Māori knowledge and ways of knowing have an essential role to play in fisheries management. Connecting with local iwi for region specific co-management of marine resources, especially inshore fisheries, will support this knowledge feeding into management decisions.
- Engage more fishers in data collection and innovation. Data collected by fishers on their catch and effort are already fundamental to the system. Some fishers would like to contribute more to science and have their observations used to support environmental monitoring. Fishers can also lead data collection, with the Moana Project illustrating the efficacy of fishers taking on this role (see case study). There is also a need to bring qualitative information into formal systems and the (sometimes intergenerational) knowledge held by fishers is invaluable in this regard. There are perceptions that data collected by fishers may be of lower quality, and evidence of an observer effect discussed in the section ‘On-board cameras are being introduced‘, highlights the need for verification. EM systems will go some way to reducing these issues and lessons from citizen science can also be drawn on to support high-quality data collection and ensure data is robust and validated.[5, 6] Similarly, experienced fishers have deep knowledge of fishing practices that can be vital to key innovations, if they are enabled to innovate.
- Opportunistic data collection. Vessels that are in the ocean for non-fishing purposes can be used to collect data to inform marine science and fisheries management. Many of the costs of conducting marine monitoring could be reduced by using these vessels and resources already operating in the marine environment. For example, acoustic information (described in ‘Acoustic technologies’) that was collected on merchant ships over seven years was used in conjunction with information on species distribution from research trawls to make the first estimates of mesopelagic[7] fish density in the Southern Ocean,[8] demonstrating the utility of opportunistic data collection to inform biomass estimates, even if what is able to be surveyed is more limited than a research vessel.[9] Plankton monitoring can also take place on a range of vessels (see section: ‘How we ensure a healthy ocean’).
- Involve citizen scientists. People from the community who want to engage in a particular issue may collect data as part of a citizen science project. Some local examples already exist, such as the use of citizen sightings to independently validate the spatial predictions that are made from habitat modelling (see case study: Supporting the community to engage in science to protect Māui dolphins). There are ongoing perceptions that data or samples collected by citizen scientists may be unusable because of lower quality. However, a great local exemplar of how to standardise data collected by citizen scientists so that it meets the requirements of government reporting shows how collection of high value data is possible. Sustainable Coastlines’ Litter Intelligence programme is a citizen science‐based initiative to measure litter on Aotearoa New Zealand’s coastlines and collate data in a national coastal litter database. The initiative follows a standardised method based on international best practice (UNEP/IOC guidelines on surveying and monitoring of marine litter), with citizen scientists surveying coastal sites and recording the type and weight of each piece of litter found. The data meets Statistics NZ Tier 1 data standards, meaning it can feed into government environmental reporting, as highlighted in Rethinking Plastics. A similar approach could be replicated in the fisheries sector.
Screengrab from the Litter Intelligence website: a citizen science effort to measure litter on Aotearoa New Zealand’s coastlines and waterways.
- Automated data collection. AI and machine learning present new ways to process larger volumes of data with less resource-intensive methods (expanded on below).
- Innovative use of observers. As aspects of the role of observers become automated, there is opportunity to employ observers in more innovative research tasks on-board the vessel or to extend the types of data they capture using new tools and technology.
- Monitoring surveys. Better coordination and structuring of routine monitoring of inshore fisheries would ensure better coverage across the inshore fisheries around the country and fill data gaps of significant benefit to fisheries management.
As technology use increases and cost decreases, and it becomes easier to automate methods or build in transparency and verification processes, it should become increasingly viable to use data collected from a range of sources in marine science and fisheries management, with confidence that it is robust and high-quality. For the data collected from a range of sources to be useful, it will need to be well organised and integrated.
An improved data system can help us move from data to information
Gathering more data and collating and integrating existing datasets is essential to guide sustainable management of Aotearoa New Zealand’s fisheries. But doing so is only going to be beneficial to fisheries management if there is a robust data management system which facilitates analytical processes to turn the data into knowledge through ongoing monitoring and scientific research. The full benefits of a rich dataset will be realised when a wider range of stakeholders is able to access the data, test theories, and build up scientific knowledge and a clear understanding of its associated uncertainties.
Aotearoa New Zealand needs a robust data management system for the marine environment
Data management techniques have improved over the years and present opportunities for greater accessibility and analysis of data. The figure below shows what data collection used to be like in fisheries on top, and below a scenario of a high-tech fishery-dependent data collection system.[10] In the old scenario, data is collected through the use of logbooks and reports, through fisheries observers, and other records and surveys. Processing this data is resource intensive and slow, and is then generally assessed by the regulator or a scientific institute to inform management decisions. It can take a year or longer to implement changes such as area closures.
In the high-tech fishery-dependent system, in near real time, data is collected from fishers (this would include recreational fishing, although this is out of scope for this report), synthesised with quality control measures and integrated with environmental data, and accessed by the management agency and scientific bodies who integrate and share their data as well. Data is fed back to the fishers who input, and management decisions can be made on a much shorter timeframe – within days, weeks or months.
A conceptual diagram from [10] (CC BY 4.0) showing one example of a status quo (top) and possible high-tech (bottom) example of a fishery-dependent collection system for data such as stock and bycatch data, whereby decision making could happen on a faster time scale. Aotearoa New Zealand is already on the path to the high-tech system, though, in practice, decisions may be limited by regulatory processes such as ministerial decision making. Note that recreational fishing is out of scope for this report but would need to form part of a comprehensive fisheries data platform.
Aotearoa New Zealand’s fisheries management system is currently on a journey towards a more high-tech system, having started to collect a lot of data electronically, but there are still obstacles (discussed in the section ‘Regulator initiatives and data transformation’). The high-tech science-driven system needs to have a bridge to the observational system embedded in mātauranga so that both types of information can be integrated into decision making. Additional challenges to moving towards a high-tech system include[10]:
- High upfront costs. Switching to a new system can be very expensive compared to continuing with the status quo. Adopting new software and hardware for data collection, processing, analysis and storage has purchase, installation and training costs. The New Zealand Government has already invested in some of the EM equipment needed to move to a more high-tech system.
- Regulatory barriers. The types of data collected in a high-tech system may not align with government requirements for reporting. For example, requirements for paper-based reporting may hinder uptake of electronic reporting. Aotearoa New Zealand is already past this particular hurdle but regulation needs to continue to keep up with new ways of capturing and reporting data so as to not hold back uptake of improved systems.
- Implementation of data collection standards. Some data collection efforts may be redundant because the data is not viewed as legitimate or it does not meet the needs or technical and performance standards required by the user. Agreeing on guidelines and standards for fisheries data would enable better utilisation of externally collected data in fisheries management.
- The need for trust and buy-in from fishers. If fishers cannot access and do not benefit directly from data, they may resist being involved in data collection efforts, especially where requirements are burdensome. Concerns around privacy and commercial sensitivities may also come into play.
Aotearoa New Zealand’s fisheries management system is currently on a journey towards a more high-tech system, having started to collect a lot of data electronically, but there are still obstacles.
Getting new data systems set up correctly from the beginning requires having the data experts and end users involved and informing what questions need to be answered by the system. For example, having end-users input early would have helped to ensure fishers are reporting how they use seabird mitigations in a way that answers vital science questions. Similarly, it will be important to ensure video data (from cameras on boats) is reviewed in ways that will meet particular statistical requirements of risk assessment modelling. In some instances, fishers need data to validate new fishing approaches and there needs to be a simple connection into the system to enable this (see case study: The importance of connecting fishers to researchers).
As we streamline, link up, and set up new data systems, it is important to effectively involve stakeholders or end-users in data system design. While establishing new data systems will always be a somewhat iterative process, where there is a lack of early stakeholder or end-user input, this can result in lost opportunities for setting up effective systems for data analysis from the start, and results in unnecessary amendments. This provides opportunities for win-wins between all participants in a data system.[10]
Efforts to improve the fisheries and marine science data systems could align with the recommendations of the Parliamentary Commissioner for the Environment relating to environmental reporting and the coordinated and long-term funding of environmental research.
This underpins recommendations in Theme 5.
Need for large and secure data storage
Aotearoa New Zealand has secure data storage for current fisheries data, but if collection and access is to expand the current system may need to change. Large amounts of data (often in terabytes) must be stored – this requires either physical hard-drives that are manually exchanged, or cloud storage/wireless transmission that may be difficult for fisheries operating in remote areas.[11] Costs of data transfer and storage are also non-trivial. Data storage must be secure and access to data must be clearly defined. The format of data will also need to be considered, for example, increases in video imagery and multibeam echosounder.
Need for improved analytical processes
A broader and more automated data collection and management approach will generate a wealth of information. Ongoing monitoring efforts will see these datasets continue to grow. Some new or complementary data collection methods may also collect more data than previous approaches. For example, acoustic data collection generates much greater volumes of data than trawl samples.
New analytical processes will be required to get through this sheer volume of data. Software that is able to automate or streamline analyses in a reproducible fashion will be an essential tool to utilise data to inform fisheries management, with a quick turnaround being critical to support fast and responsive decision making (see case study: Software to streamline acoustic data analysis). These efficiencies will potentially be further improved by the use of AI and machine learning in analytical processes (discussed below).
Need for data to be widely accessible
Transparency and access are essential to enable the quality and integrity of science and analysis and good peer review. Data transparency is also beneficial for traceability and market access (see ‘Improving traceability to add a premium to products’). The move to proactive anonymised data release, including metadata and context alongside the raw data, is long overdue. There are multiple benefits from making aggregated data more widely accessible within a shorter timeframe. Doing so can facilitate greater responsiveness in fisheries management actions by both industry and regulators, support researchers to develop new insights about the marine environment, and enable greater transparency and accountability for all stakeholders. Greater transparency can help those in fisheries who want to more actively manage their impacts on the environment.
There is also opportunity to make some data even more widely available, such as in public online databases, so that it can be accessed for different research and management needs. Requiring publicly funded research to have open access datasets may be a first step to achieving this goal.
Providing access to datasets could allow for novel analysis and new insights. Reciprocated data sharing between different groups could provide a fuller picture of the state of the marine environment and fisheries and could prevent duplicating data collection efforts. There is also opportunity to make some data even more widely available, such as in public online databases, so that it can be accessed for different research and management needs. Requiring publicly funded research to have open access datasets may be a first step to achieving this goal. It also would allow the public access to information that provides a more complete picture on the state of our fisheries and environment, which may build trust.
There would be great benefits in aligning with Te Mana o Te Taiao – Aotearoa New Zealand Biodiversity Strategy 2020 goal that ‘national, agreed common data standards and open data agreements are ensuring that everyone has access to a federated repository of biodiversity information’.
Data privacy and intellectual property is a major concern that must be addressed through consultation with fishers, iwi and other stakeholders. Commercial sensitivities may be managed through data anonymisation and data aggregation. However, some concerns around data privacy relate more to surveillance and the risk of sensationalisation of imagery. For example, camera footage from boats currently depicts people in a workplace. There may also be privacy concerns related to identifying locations of protected or threatened species. Unrepresentative imagery of a dead protected species could also be taken and used out of context which influences industry buy-in.
Future developments for camera technology might help to reduce these concerns. The Datalink system being built into Tiaki to develop a release-at-depth strategy may provide a first step to remove some intrusion in the workplace by capturing footage underwater (see case study: Precision Seafood Harvesting – Tiaki). The specific concerns of iwi relating to data rights, data privacy and intellectual property must be factored in to any processes that make data more widely accessible. Establishing trust and appropriate conditions for sharing of data is important and it may not be appropriate for that to be wholly accessible. A data trust is a possible way to provide stewardship over data while enabling wider access.
There would be great benefits in aligning with Te Mana o Te Taiao – Aotearoa New Zealand Biodiversity Strategy 2020 goal that “national, agreed common data standards and open data agreements are ensuring that everyone has access to a federated repository of biodiversity information”.
Improving access to data could build on the existing systems. See the section ‘Who collects data?’ for a list of some existing databases.
Need for effective data visualisation tools
A significant volume of data is available from four standardised time series of research trawl surveys conducted for the regulator over the last 30 years. This data can be used to develop indicators of fisheries ecosystem health and change, but access to, and communication of, this data has mostly been via individual lengthy survey reports, making it challenging to determine trends over time. Data visualisation tools that are able to be used across common platforms will be more accessible than specialist programmes.
Access to, and communication of, this data has mostly been via individual lengthy survey reports, making it challenging to determine trends over time.
Some visualisation tools already exist, such as Sea Sketch – a publicly accessible tool for visual marine planning that was used in Sea Change Tai – Timu Tai Pari. The web-based platform, Fishecoviz, in the early stages of development[12] is another step towards making data more visually accessible. The prototype includes Chatham Rise trawl survey data but could be expanded to include other ecosystem components (e.g. oceanographic indices) and other areas. The portal currently includes several interactive pages where the user will be able to explore and compare species and ecosystem indicators, biomass trends and feeding relationships and view species distribution on a map. In order to be effective, the portal needs to be publicly accessible and user friendly.
A web-based delivery platform, DOC2.0,[13] is being developed to provide data that is currently only accessible as pdf or hard copy versions of New Zealand Fisheries Assessment Reports to Fisheries New Zealand and other users. The concept is for searchable delivery (and download) of peer-reviewed published outputs (rather than of raw data). A beta testing version has been developed and is currently being reviewed by users with further implementation planned in 2021.
The need to improve Aotearoa New Zealand’s fisheries data system is addressed in recommendations in Theme 5.
AI and machine learning have the potential to increase efficiencies
Increasing computer power combined with improved technology means we have the potential to gather more data about our oceans than ever before. But this data is only useful if we can analyse and extract meaning and knowledge from it. Our ability to analyse data has not kept pace with the data collection explosion, resulting in an ‘analysis bottleneck’.[14] Besides the sheer volume, the data collected is increasingly complex and can vary substantially in quality. This poses further challenges for data analysis efforts.
Most AI-related fisheries projects – both in Aotearoa New Zealand and overseas – are still at the proof-of-concept stage.
AI and machine learning can widen the analysis bottleneck and accelerate the shift to responsive data-driven fisheries management. AI technology has the potential to make fishing more precise, cost-effective, transparent and sustainable by improving the efficiency of manual work. It can also enhance marine and fisheries science by providing new approaches to analyse complex datasets.
However, most AI-related fisheries projects – both in Aotearoa New Zealand and overseas – are still at the proof-of-concept stage. Examples of pilot and early-stage applications are discussed throughout this section. Several hurdles must be overcome in order to progress this emerging technology beyond small pilot projects. Progress in this area will be critical to being able to fully utilise the wealth of data that will come from projects in the pipeline, such as cameras on boats.
What is AI and how can it help us fish sustainably?
The AI Forum of New Zealand defines AI as, “Advanced digital technologies that enable machines to reproduce or surpass abilities that would require intelligence if humans were to perform them”.[15] Currently, AI can perform specific, narrow tasks very well, like playing chess, working out a protein structure[16] or operating a self-driving car. However, we are still a long way off generalised, creative AI that can perform any task, like a human brain.[17]
AI is an umbrella term that encompasses a range of different approaches. Some of the approaches relevant to fisheries are defined below.
Machine learning
Machine learning is an application of AI where algorithms learn and improve from experience, rather than being explicitly programmed.[18] These computational methods sift through vast amounts of data, identify patterns in the data, and then apply these patterns.
Most machine learning is supervised. This is where the algorithm is ‘trained’ on human-labelled input and output data – effectively telling the algorithm what patterns to look for. When the input data does not have labels, the machine learning is unsupervised. In this case, the algorithm looks for whatever hidden patterns it can find. Reinforcement learning sits somewhere between supervised and unsupervised learning, building on both exploration of the unknown and exploitation of current knowledge.[19] Through trial and error, the algorithm attempts to achieve a goal. Its actions are either rewarded or penalised and by striving to maximise the reward, the machine ‘learns’.

Schematic of the different approaches to machine learning.
Deep learning and artificial neural networks
A neural network is a type of machine learning system inspired by the structure of a human brain. It consists of computational nodes that are interconnected.[20] Deep learning is a method that employs nodes arranged in layers, where the outputs of one layer feed into the next layer.[21] For example, the first layer of a deep neural network might identify corners and edges in an image, which then feeds into another layer that identifies higher-level shapes, and so on. A convolutional neural network (CNN) is a type of deep neural network inspired specifically by the human vision system.[22] Most deep learning systems for image analysis use CNNs.
These methods could help us fish more sustainably by expanding opportunities for data collection and automating processing of fish data, greatly increasing the amount of data and information that can be drawn on to inform fisheries management decision making. For example, US company, CVisionAI, is working on AI solutions to automate trawl survey video review, detect scallops and recognise activities on-board fishing vessels.
US company, CVisionAI, is working on AI solutions to automate trawl survey video review, detect scallops and recognise activities on-board fishing vessels.
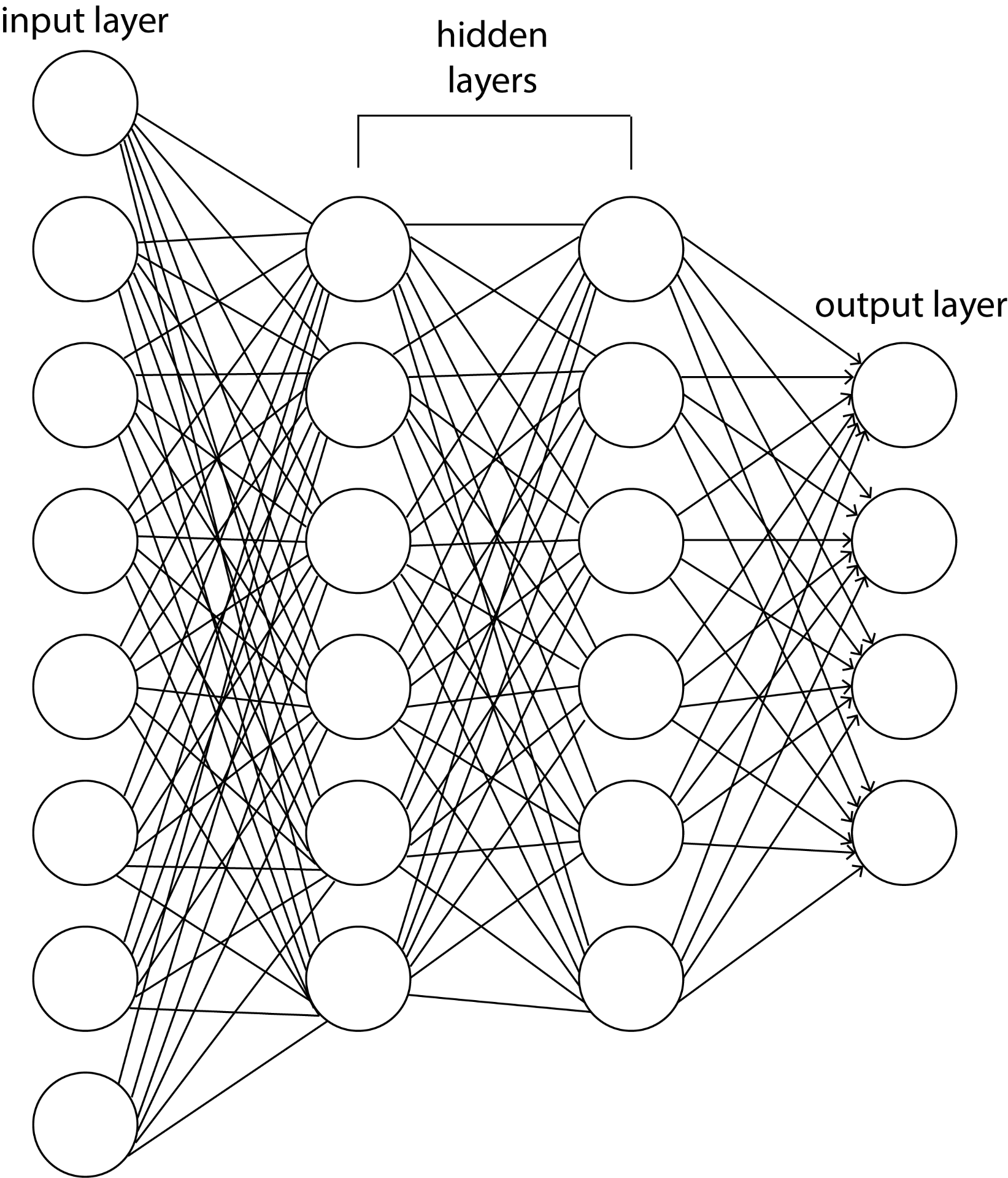
Visual representation of a simple neural network.
Differences in annuli counts (top) and age bias (bottom) between annuli estimations via machine learning and human age estimations in the machine learning trial of Moore et al.[24]
AI opens up opportunities to automate processing of fish data
Measuring fish length is one data collection aspect that lends itself to a computer vision solution and may present a suitable first step towards widespread AI implementation in fisheries.
In Aotearoa New Zealand, Pisces Research Ltd is developing a fish measurement app (“Snappy”). The app could be used in onshore processing facilities, which have less variable photography conditions than on vessels at sea. The length data collected can inform both stock assessment and add useful product information to benefit companies.
Fish age is an important parameter in stock assessments (see section ‘Data collection on target stocks and accessibility of this information’). The age of a fish is determined by inspecting its otolith – similar to counting rings in a tree trunk. Otolith interpretation is time- and labour-intensive, requiring specialist knowledge. But computer vision can now be deployed to measure fish otoliths (a structure in the inner ear).
A study from 2018 used deep learning to estimate age from Greenland halibut otolith images.[23] The resulting CNN performed at level comparable to human accuracy.
In Aotearoa New Zealand, tens of thousands of otoliths are collected from commercial fisheries and research surveys every year (at least 27,000 in 2019/20). A feasibility study on automatic image processing for hoki, ling and snapper otoliths has been carried out[24] and concluded that researchers will still need to be involved in the process prior to age estimation and the technology required to estimate age from CT scanned otoliths needs further refinement, but there is significant potential for automating age estimation from otolith images using machine learning. This could speed up the age estimation process overall.
While the technology required to estimate age from CT scanned otoliths needs further refinement, there is significant potential for automating age estimation from otolith images using machine learning.
There are limitations and challenges in applying AI in the commercial fisheries sector
Successful scaling up of AI technology across the fisheries sector will require collaboration and dialogue between fishers, academic researchers, NGOs and the private sector. While AI has the potential to effect change in fisheries, in practice there are several hurdles that are currently limiting implementation to small research projects and pilots.
Transparency and privacy
- Data privacy and intellectual property (as discussed above) is important in considering how AI is used to capture and interpret data.
- Neural networks are essentially ‘black-box’ systems and it is often unclear how such models make decisions, or what information they are capturing.[14, 22]
- Transparency in the use of algorithms and how decisions are made are important. Government agencies that are signatories to the Algorithm Charter for Aotearoa New Zealand have committed to the transparent use of decisions made informed by algorithms.
Retaining the human touchpoints and building trust
- The Nature Conservancy reported in 2018 that some experts “are sceptical of the near-term impact of AI”.[25] They believe that human involvement in certain technologies – such as the implementation of EM – is necessary to build trust and achieve buy-in from fishers.
- As with many other industries, AI and automation may make manual human labour obsolete. This is of concern for small, coastal communities that rely on fisheries for their livelihood,[26] although potentially people could then be involved in more meaningful work.
- Development in the AI and fisheries space should focus on collaboration between human and machine – a partnership that takes the best parts of both – rather than replacing humans with AI.
Cost and funding
- Although there may be mid- to long-term financial benefits to AI, there is an up-front cost associated with hardware, and ongoing costs associated with data transfer and data storage that may be prohibitive.[11] This may be an area where government support makes a significant difference to improve sustainability of fisheries management.
- Sustained investment is required to progress the technology from small-scale to wide rollout and stay up-to-date as the technology evolves. The current cost-recovery model of fisheries research funding in Aotearoa New Zealand is not effective for advancing blue-skies innovation into practice.
- There is a limited market size, especially in Aotearoa New Zealand, but we could sell our technology to other jurisdictions.
The right data must be available and useable
- The data we need to make decisions needs to be collected in the first place.
- Machine learning requires training datasets, often with annotations. Datasets with adequately labelled metadata are few and far between.[14] Some research using CNNs is aiming to develop solutions for sparsely labelled data.[22]
- Different fisheries will require custom training datasets depending on the species they target.
- Image recognition in a fisheries context is particularly challenging, with environmental conditions that may include rough seas, changeable weather and moving objects.
Image libraries are critical for the development of AI
- Image libraries are a crucial part of the application of AI and machine learning in computer vision solutions and developing these is a critical step to being able to apply these technologies widely in the industry.
- One example of a dataset with labelled fish images designed to train AI systems is Fishnet, a library of over 100,000 tagged EM images.
- All government-mandated EM programmes could require provision of source-anonymised labelled images to an appropriate publicly accessible dataset. Organisations operating non-government EM systems could also be encouraged to provide suitable labelled images and images may also be obtained from fisheries trawl surveys.
Data must be stored
- The issues around data storage (discussed above) are particularly relevant to AI.
Development of AI expertise
- To date, AI and machine learning expertise has largely been concentrated in big data companies such as Google. Fisheries and marine scientists must build and enhance connections with experts in the AI space, in order to attract talent and interest in fisheries-specific issues.
Biases
- Depending on the code, inherent biases may be programmed into the application and perpetuate biases.
How we fish
There is a fine balancing act between fishing at scale economically and fishing to minimise social, environmental and ecosystem harm and maintain a sustainable harvest for future generations. Gear innovation is a key way to help strike this balance and ensure that fishing activities do as little damage to the marine environment and ecosystems as possible.
Responsible sourcing of seafood is fundamental to a sustainable fisheries sector because it means that there will be healthy ecosystems and habitats and strong juvenile populations of fish, with the added benefit of high quality catch due to less damage during harvest. It will also help to industry maintain social and cultural licence to operate. The key issues that relate to fishing gear that need to be addressed by innovation are:
- Selectivity. Being able to catch target species of a particular size is critical to maintain healthy ecosystems because it selects for the size of fish and avoids unintended species. Selective fishing approaches can be designed based on conventional approaches of targeting specific fish above a certain size, or alternatively be designed for balanced harvesting (applying a moderate fishing intensity across as much of the ecosystem as feasible[27]) thus expanding the range of sizes and species considered as the target. As discussed in ‘Fisheries management involves the use of many different tools’, selectivity also has economic implications due to the deemed value associated with fish that are caught without ACE. Mesh sizes, hook sizes and configurations, net configuration, and jigging technology all have the potential to improve selectivity.
- Bycatch. As well as not wanting to catch non-target fish species, there is huge effort to reduce the bycatch of protected species and vulnerable benthic habitats, in line with the bycatch reduction goals of Te Mana o te Taiao – Aotearoa New Zealand Biodiversity Strategy 2020. The degree to which bycatch is an issue is species- and location-specific (see ‘Bycatch of non-target and protected species’) demanding bespoke management and innovation solutions. Innovations relating to how gear is used and adding on equipment that deters non-target species or reduces benthic impacts can help to reduce bycatch, which can sometimes be mandated for certain protected species and habitats. Trawls can capture bycatch while ropes and lines from other methods may entangle species underwater or at the surface. Innovations that are effective at avoiding, detecting or deterring protected species and benthic habitats vulnerable to fishing gear have the potential to allow fishers to continue to fish where these species and habitats are present or be better informed about where not to fish.
- Damage to environment. Fishing gear such as trawls, dredges and seines can damage the environment by coming into physical contact with habitats. One innovation challenge is to be able to harvest bottom-dwelling fish with minimal damage to the seafloor. Loss of fishing gear in the ocean can also damage the environment, particularly gear made of plastic which gradually degrades into microplastics (see section in this report ‘Plastic pollution is building in the ocean’, or section 2.4.4 of Rethinking Plastics in Aotearoa New Zealand).
- Survivability. Whether fish are alive when landed on deck or damaged or killed from the catch process has significant bearing on whether it is viable to return unwanted catch (for discussion see section ‘Discards’) and also the quality of the fish, which can dictate price-point (see case study: How a commitment to transparency and traceability has generated a premium product). Again, this must be approached species by species as some species cannot be brought to the surface alive. There are significant economic benefits to be realised from landing fish in better condition and returning live bycatch – gear innovations in all fishing methods are working to address this issue.
Innovations can also address other needs to change how we fish.
- Reducing harm from ‘ghost gear’. Lost or abandoned fishing equipment is a significant threat to marine animals and can also damage the environment. Gear innovations that improve identification to retrieve gear if lost can help to address this issue. The choice of materials used in gear can also make a difference to the harms caused (see section 2.4.4 of Rethinking Plastics in Aotearoa New Zealand).
- Locating fish to reduce fuel use. Technologies that locate fish can increase efficiencies of fishing trips and therefore have positive climate change implications. This can also reduce fishing effort and therefore impacts on associated and dependent species. Uptake might be increased if government support were available. Notus Electronics (Canada) have developed a wireless microphone which can hear shrimps ‘ping’ off a grid as they enter the trawl, enabling detection of hotspots along a tow. This technology could potentially be applied to Aotearoa New Zealand’s scampi fisheries.
- Moving away from fossil fuels. Shifting to lower fuel use methods and incorporating alternate energy sources where possible is beyond the scope of this report, but an important climate change consideration for the sector.
- Understanding fish behaviour. A better understanding of how target and non-target species respond to fishing can help the industry design gear that is more selective.
Fostering more gear innovation will help to make fishing fit for the future (see case study: Gear innovation pathway). Gear innovation doesn’t always rely on inventing completely new ways of fishing, it can also involve incremental improvements in the traditional ways we have fished, using nets, lines, hooks and traps. A range of innovations in the existing fishing equipment and methods, as well as some additional new features, can change their environmental, economic and social impacts.
In this section we outline the current innovations and potential for improving the commonly used commercial fishing methods to make these more sustainable.
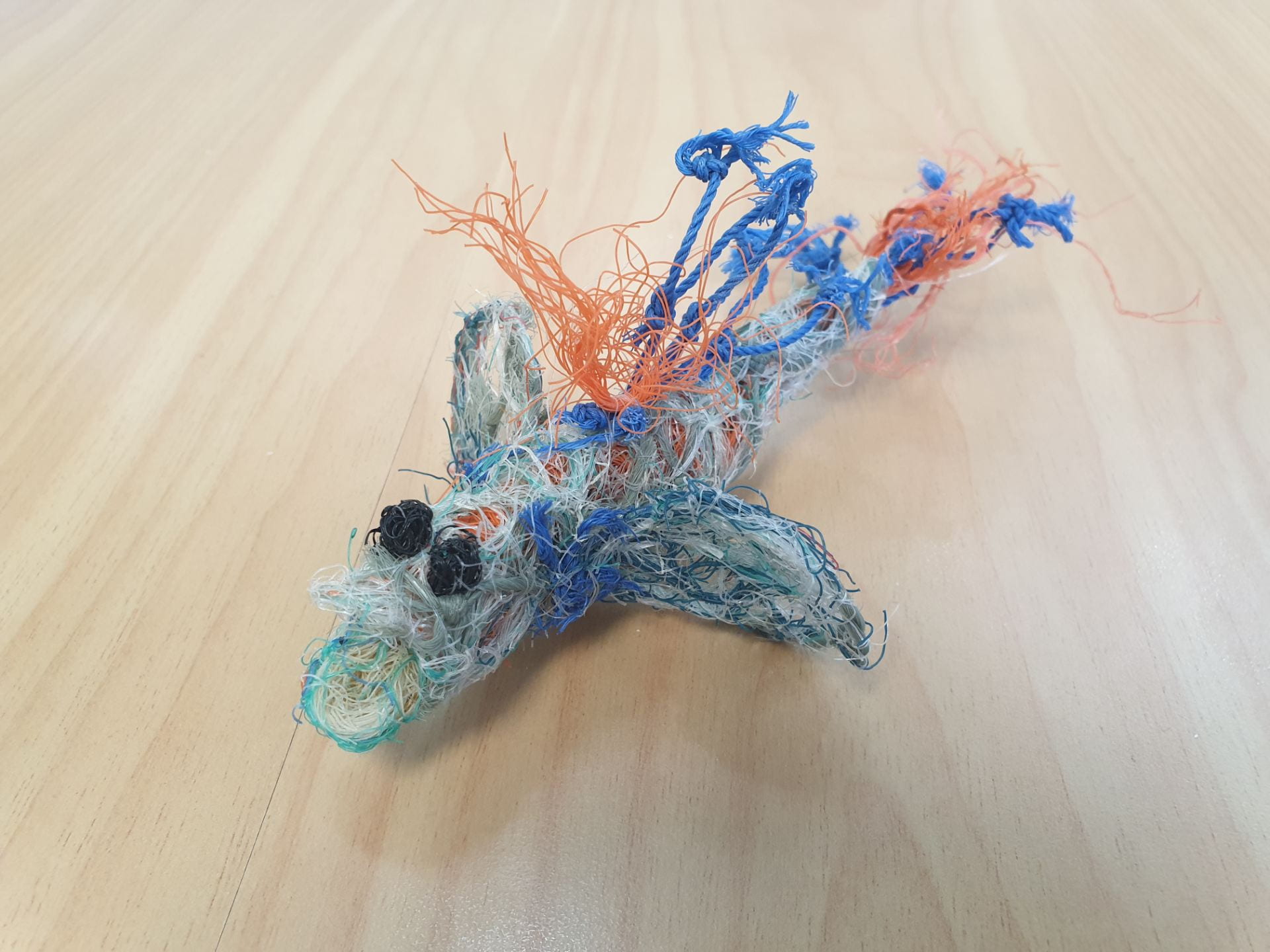
A fish made from abandoned fishing gear (‘ghost gear’) by artist Lynette Griffiths.
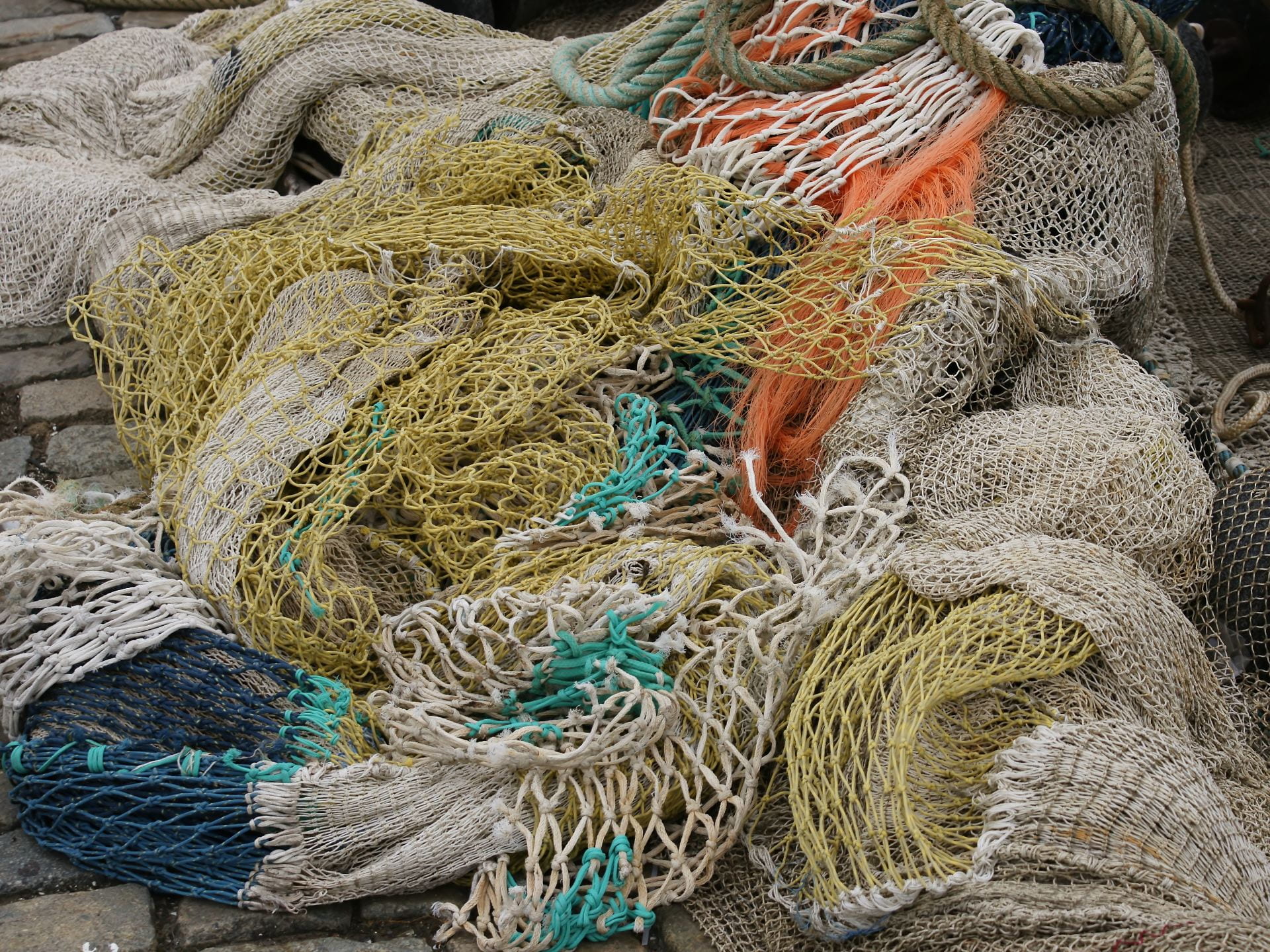
Fishing nets. Image credit: Hans Hillewaert/Wikimedia (CC BY-SA 3.0).
The technologies surveyed in this section inform our recommendations in Themes 4, 5 and 7.

Understanding fish movement and behaviour is important for gear design. Image credit: Koheru (Decapterus koheru) school, Sarah Milicich/iNaturalist (CC BY-NC 4.0).
Knowing how fish behave is essential for gear design of the future
Fishers have long analysed fish behaviour and response to different gear to develop more efficient approaches to fishing.[28, 29] However, new challenges facing fishers today demand gear that is highly selective and maintains conditions that support fish welfare before the catch is landed. Knowledge of fish behaviour in relation to fishing gear is a prerequisite to design, construct and operate new responsible fishing gears.
Historically, observations and lab-based studies have been relied on to understand fish behaviour in the context of capture methods because of the difficult and costly nature of studying this in the field. However, technological developments with underwater video cameras (see section ‘Underwater and surface cameras give a wider and sharper view of the ocean’) are opening up new opportunities to ascertain how fish behave in the natural setting because it is now cheaper and more accessible to study (as demonstrated in the case study: Fish behaviour and catchability in fishing gear).
With a better understanding of how both target and non-target species respond to fishing gear and methods, gear can be designed to be less harmful and more selective for size and species. This information can also feed into improved trawl survey design so that these methods are non-lethal.
Aspects of fish behaviour that need to be understood to inform gear design include:
- Swimming speed and movement patterns. The speed and movements of fish need to be understood in order to design non-lethal trawl methods so that the fish harvested are top quality and the bycatch can be returned unharmed.
- School movements. Japanese researchers developed a simulation model of fish-schooling behaviour to determine the most effective set net design to be selective and reduce bycatch.[30]
- How fish respond to visual cues. Understanding more about what fish see and how they react to gear will help to attract certain species.
- How fish respond to sounds. Innovations to improve the use of sound to selectively attract fish could be beneficial, but only if developed with consideration of the need to minimise anthropogenic ocean noise as it can impact marine life.[31, 32] The use of sound to guide fish to another location could possibly be applied in a way that shifts schools of fish away from a protected habitat before fishing effort takes place. Sounds can also be used to deter non-target species (as discussed in ‘Gear add-ons will be essential to deter non-target species’).
- How fish respond to olfactory stimuli. Understanding how olfactory queues could be used to select for some species and deter others may also open avenues to use these methods in new gear. Bait analysis tests for scampi that fed into potting methods are an example of this in action.[33]
- Diet. Knowledge of fish diets can inform approaches to fishing such as identifying effective baits for trap fishing. A local study that used DNA analysis (specifically metabarcoding) identified the diet of deep sea scampi and found that scampi have a varied diet, with a high reliance on scavenging a diverse range of species from the seafloor.[34] Analysis of diet also enables a way to detect if diet is changing in response to environmental shifts.
Fish behaviour has already informed some design aspects of innovative gear in use in Aotearoa New Zealand’s commercial fisheries. The net design and trawl speed of the Precision Seafood Harvester – Tiaki, were designed such that fish swam and survived until landed on deck (see case study: Precision Seafood Harvesting – Tiaki). The potting technique profiled in the case study ‘Potting as an alternative to trawling’, also based its design on prior behaviour studies.[35] Some challenges still need to be overcome so that fish behaviour can inform gear design, with multi-species fisheries posing a particular issue.
Future nets, trawls and dredges could be more selective with reduced impacts
Full contact bottom trawling can cause damage to the marine environment when it drags along the seafloor, as discussed in the ‘Habitat’ section. The impacts on the physical habitat depend in part on the weight and structure of the ‘trawl doors’ and the use of other things such as chain and rigging, as well as the specific nature of seafloor ecosystem.[36] Midwater trawling has less impact on the benthic environment but cannot catch bottom dwellers (such as blue cod, tarakihi and ling) and can still damage and kill unwanted species, including those that are bycatch. There is also some evidence that midwater trawls can sometimes come into contact with the seafloor depending on the target species, though contact is not extended.[37]
Midwater trawling has less impact on the benthic environment but cannot catch bottom dwellers.
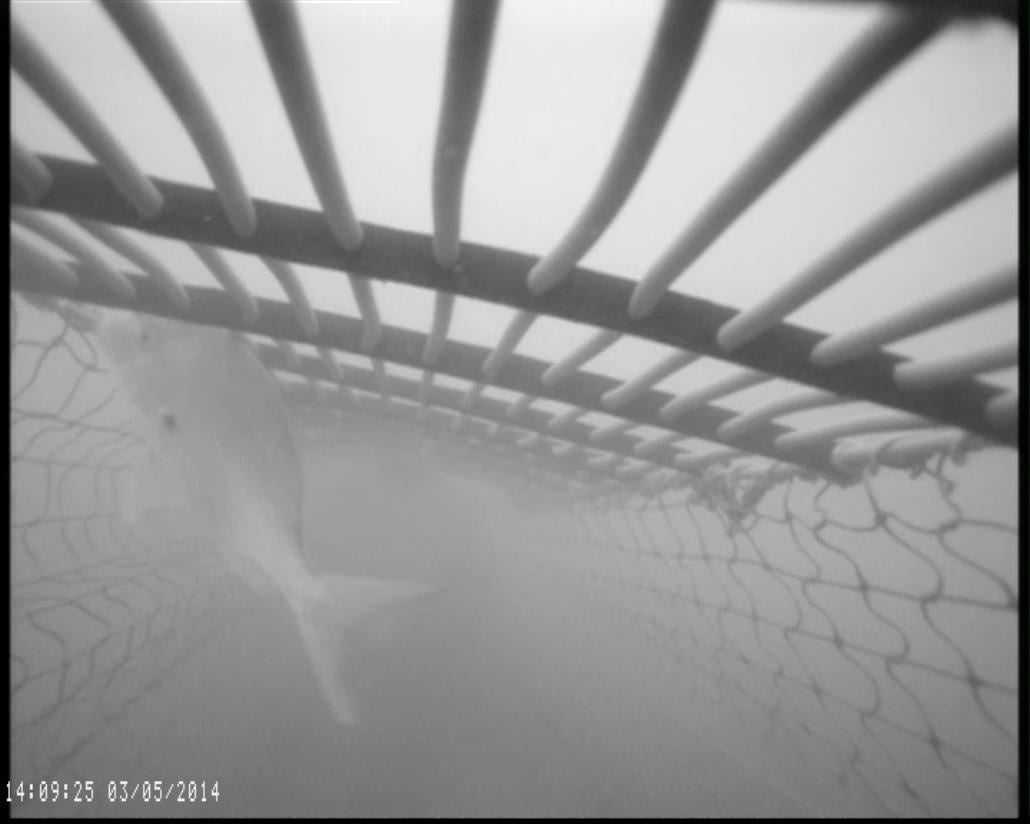
Underwater screen shot of a snapper attempting escape in a trawl fitted with a novel escape panel. Image credit: NIWA.
Selectivity
Much of the focus in trawling innovation relates to selectivity by selecting for fish that are a marketable size. For nets and trawling, this is largely determined by the size and shape of mesh openings in the net, particularly at the end of the net where the fish accumulate (the ‘cod-end’), and fish behaviour during the capture process (see section above ‘Knowing how fish behave is essential for gear design of the future’). The simplest way to reduce the catch of undersized fish is to increase the size of meshes in the cod-end or use selectivity grids where appropriate and allow the fish to escape. Some fish that pass through the nets die during the process.[38] In the future, greater selectivity could also be achieved if bycatch is caught alive and healthy and released back to the ocean unharmed.
In the future, greater selectivity could also be achieved if bycatch is caught alive and healthy and released back to the ocean unharmed.
After many years fishing with traditional gear, in recent years some in the fishing industry have shown leadership and taken steps to increase mesh size above the minimum legal mesh size in some fisheries or adopt the use of different mesh types such as square or ‘T90’ that allow mesh to remain more open during fishing than traditional diamond mesh. Napier-based skipper Rick Burch uses T90 mesh and has demonstrated the effectiveness of this simple change to reduce catches of undersized gurnard by 61%.[39] The different mesh sizes and configurations that work will depend on the target species and size of fish targeted.
Even with larger or more open apertures in a trawl, the selection process is relatively passive, and is currently only effective if the target species is larger than the unwanted catch, or a shape that prevents it escaping as easily. For example, square or T90 openings are very effective for species that are round in cross section such as red gurnard but may be less selective for more elliptical or compressed body shapes such as flatfish, snapper or tarakihi. Fisheries generally encounter multiple species of different sizes and shapes, which can make selective, targeted fishing particularly difficult in our ocean, where there are often a large number of species in the same area compared to some fisheries overseas.
In recent years, some in the fishing industry have shown leadership and taken steps to increase mesh size above the minimum legal mesh size in some fisheries or adopt the use of different mesh types.
Other tools such as sorting grids and exclusion devices also provide a way to select for the target species and reduce bycatch by allowing for the escape of larger animals when they come into contact with grid bars at the top or bottom of the trawl net, but not ejecting the smaller target species. An example is the sea lion exclusion devices (SLEDs) used in the squid fishery near Maungahuka the Auckland Islands (SQU6T) and the southern blue whiting fishery near Motu Ihupuku Campbell Island. A review concluded SLEDs have contributed to reduced rates of observed sea lion mortality[40], though these findings are contested.[41] Separator panels with multiple cod-ends of different mesh sizes have achieved successes in some fisheries in Aotearoa New Zealand. However, these approaches are not applicable to smaller bycatch species. There is room for improvement in bycatch reduction innovations in Aotearoa New Zealand’s commercial fisheries industry.
Combining gear innovations with other technologies such as acoustic and video technology could in future facilitate more selective methods and reduce the trawling impact as the trawling effort needed is reduced.[42] Advances in computer vision (discussed above, in ‘AI and machine learning have the potential to increase efficiencies’) coupled with more selective design will allow for selection to take place at depth and allow for underwater release to reduce discard mortality. Video imaging and acoustic technologies could be coupled for pre-catch identification and catch monitoring to potentially increase catch rates of target species and reduce the trawling footprint.[42] Among a range of international examples is a local project that aims to develop a video-guided active sorting device (see case study: The importance of connecting fishers to researchers). On a larger scale, a collaborative, multimillion dollar project led to the development of a modular harvesting system that offers significant advantages over traditional nets but does not address seabed impacts (see case study: Precision Seafood Harvesting – Tiaki). Development of underwater computer vision to add to the harvesting system is currently underway.
Reducing bottom impact
There are also opportunities to innovate in trawl design and materials to lighten the weight of equipment so that it doesn’t drag along the seafloor and damage habitat (the impacts of this are discussed in ‘Habitat’).[36] Innovations to change the extent to which these gears contact the seabed have dual conservation and production gains in that as well as avoiding habitat and species disturbance, they reduce fuel consumption and expensive damage to trawling gear.
A number of techniques have been developed overseas to reduce the impact of trawl fishing on benthic habitats, including semi-pelagic trawl where trawl doors are lifted off the bottom rather than in contact with the seabed, and raised fishing line trawls. These are thought to be suited to application in some of Aotearoa New Zealand’s fisheries and could be tested in our setting,[36] building on early testing of semi-pelagic trawls.[43]
A precision robotic shellfish picker is under development by researchers at the University of Canterbury to address the issues related to dredging (as discussed in the case study: Scallop surveys and harvest).
Trialling gear
Understanding and quantifying the selective properties of trawl fishing gear and its impact on the seafloor is an important tool in achieving sustainable fisheries. Trialling different gear innovations at sea can be costly and time consuming. There are two ways to address this.
The first is to model and predict the selectivity outcomes of changes in mesh sizes and openings to refine the proposed method until predicted selectivity is optimised. Researchers at NIWA are working on a project to achieve this, combining conventionally collected experimental selectivity results with ‘artificial’ selectivity data for a range of different mesh sizes and opening angles created by performing ‘fall through’ experiments (see figure on right).[44] So far this project has developed predictive models for four species (snapper, red gurnard, New Zealand sole/pātikirori[45] and yellowbelly flounder/patiki-totara[46]), providing quantitative information on the changes in selectivity that could be expected from the inshore vessel shifting from the minimum legal mesh size (4” or around 100 mm) to larger mesh sizes of 5” (125 mm) and even 6” (150 mm) diamond mesh. Data on fish sizes and shapes has also been collected for sand flounder/pātiki[47] and tarakihi, as a basis for future trials. Once predictive selectivity models are finalised, the data can be summarised into an interactive app which shows how changes to mesh size and opening affect selectivity across different species.
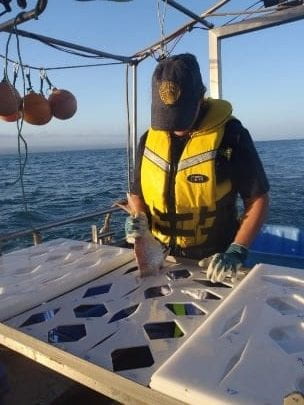
Collecting “artificial” selectivity data from “fall-through” experiments. Photo credit: Jure Brčić/NIWA.
The second approach is to use flume tanks to test systems. Flume tanks provide a physical environment to carry out performance evaluations of the new gear and simulate underwater and near-surface conditions. There are only a handful around the world for testing trawling gear and Aotearoa New Zealand’s researchers and industry collaborate with these institutes. Independent fishers who supply Talley’s have visited the flume tank at the Marine Institute at Memorial University in Newfoundland, which is the world’s largest flume tank, to test gear innovations. A flume tank is going to be built at Plant & Food Research which may be able to be used for testing gear locally.
There are remaining challenges in testing net and trawl innovations in the marine setting. In particular, it is challenging to capture data about survivability following escape from the net. Video footage is helpful in distinguishing between live and dead bycatch being released, but cannot capture subsequent events, such as predation. Tagging of fish may give some clues, but is extremely resource intensive. Beyond the benthic impacts, we also need to study the impacts of the gear on the ecosystem more broadly. A more permissive regulatory environment that maintains rigour is needed to enable controlled fishing trials of innovative gear with careful monitoring of the impact on stock numbers and the ecosystem over time. This could be achieved through special permits to support innovation.
A more permissive regulatory environment that maintains rigour is needed to enable controlled fishing trials of innovative gear with careful monitoring of the impact on stock numbers over time. This could be achieved through special permits to support innovation.
This underpins recommendations in Theme 6.
Looking to the future, towed capture methods will need to continually embrace technology that allows more active selection, identifies unwanted catch before or during the capture process, avoids or releases species at depth unharmed, and doesn’t come into contact with the seafloor. Alternative methods also need to be evaluated for differences in fuel use or emissions. Facilitating this gear innovation would be assisted by review and amendment of the Enabling Innovations in Trawl Technology regulations to better facilitate the development and use of innovative fishing technology. Funding and technological support for gear trials could also reduce barriers to gear innovation, as would a review of how the ‘third wire’ regulations can restrict innovative uses of technology.[48]
Not every new innovation will work first time and a permissive environment is needed to allow fishers to iterate and optimise new gear within their local context to give a fair comparison to established technology.
This underpins recommendations in Theme 7.
Innovations could increase efficiencies and reduce harm from line fishing
Innovations to improve the efficiency of non-trawl methods will be essential to make them more economically viable and enable wider use to minimise environmental harm from fishing. Compared to trawl and net methods, line fishing is less efficient for large commercial catches, but it can be more selective, land fish of higher quality and have less benthic impact. The ability to catch fish on a hook and line system is also dependent on the behaviour of the fish species in response to baited hooks as well as its physical ability to take a hook. Line fishing will not necessarily be effective for all species.
Compared to trawl and net methods, line fishing is less efficient for large commercial catches, but it can be more selective, land fish of higher quality and have less benthic impact.
Electronic jigging technology
Innovations in electronic jigging enable more efficient and precise hook and line fishing. A computer-controlled motor on the side of a boat drops a weighted line into the water, automatically finds the bottom, retracts enough to avoid seafloor contact, and then moves the line up and down in a jigging pattern to attract fish. The line is reeled back up when pressure sensors detect that a set weight of fish has been reached. Specific line movements, depth and lures can be used to attract a particular species of fish by mimicking prey movements, enabling selective fishing. For example, some fish will follow a lure to the surface while others won’t. This makes it a particularly useful innovation to support fishers to catch their quota and avoid non-target species. This method is used widely in the inshore fisheries in Iceland.[49] Murihiku Southland-based fisher Nate Smith uses computer-driven jigging technology (see case study: How a commitment to transparency and traceability has generated a premium product).
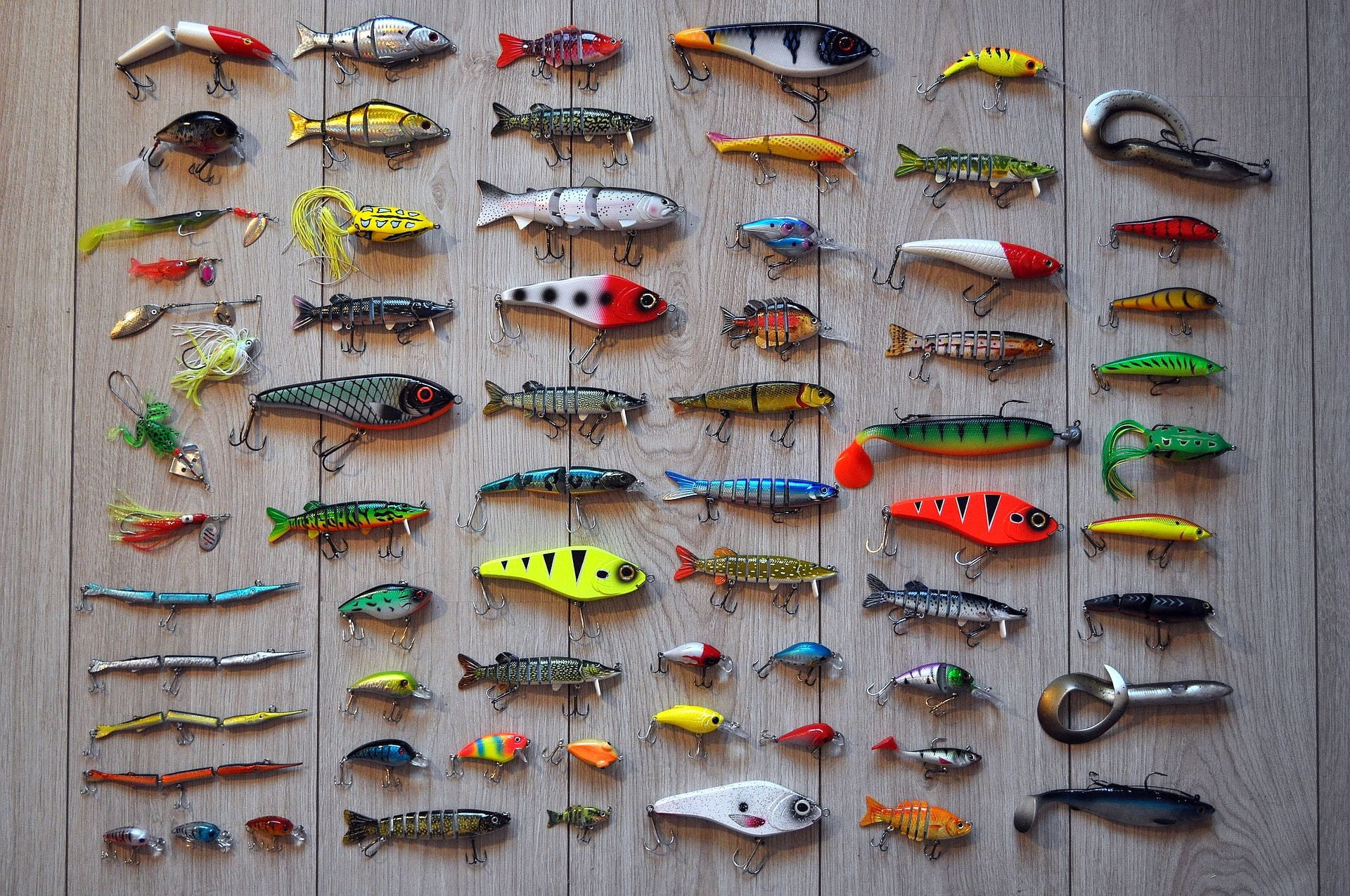
Fishing lures called ‘jigs’ come in a variety of shapes, sizes and colours.
A Nature Conservancy project supporting fishers to fish sustainably in the Gulf of Maine has equipped nine boats with this technology at no charge so that they can test it before purchasing at a discounted rate if they want to continue to use the method. Though one fisher could manage a number of electronic jiggers at once via an on-board computer, the current cost of the technology may hinder uptake at a scale that would make it economically viable to adopt this method over alternatives such as trawling. However, it does enable the harvest of selected quality fish for appropriate small, premium markets, and could be more widely adopted with investment to help operators find a route to these markets.
Underwater hook release
Gear innovations are required to address a key issue with line fishing – bycatch (see ‘Bycatch of non-target and protected species’). Hooks floating on the ocean’s surface are commonly mistaken for food by seabirds, which can get caught by the hook. This is a particular issue in longlining where hundreds or thousands of hooks are used at once. Changes in fishing practice, such as setting longlines at night, weighting lines, and bird-scaring decides go some way to reducing these impacts, but gear innovation has the potential to further reduce risks to seabirds. Innovations to address this issue focus on releasing the hook underwater out of accessible reach of seabirds. An underwater bait setter innovation developed by local fishermen is showcased in the case study: A collaborative effort to protect vulnerable seabirds. A separate but similar innovation developed in the UK, the Hookpod, encloses baited hooks in a case and then releases hooks at depth. These have been tested by commercial operators in Aotearoa New Zealand and are approved by the Ministry for Primary Industries as a standalone seabird bycatch mitigation measure. These innovations mitigate the risks of seabird bycatch on the sea surface during setting of the gear, but underwater risks of hooks or entanglement still exist for other seabirds and other marine animals.
Traps and pots could be redesigned to eliminate entanglements and gear loss
Trapping and potting methods are traditionally used to catch crustaceans such as rock lobster but innovations in how these methods are applied open them up to a wider range of fisheries.
Ropeless gear
Work is underway to develop and test ways to use pots and traps without the ropes that are traditionally used to bring the pot to the surface. These efforts aim to reduce the harm to marine life that ropes can cause through entanglement and gear loss in the marine environment, which is both costly and damaging. The pots sit on the seafloor and are released when an acoustic signal is sent (see ‘Acoustic technologies’). The acoustic modems can also record sounds to identify the locations of protected species such as whales, so that vessels can avoid these locations. The state of California has proposed new regulations for ropeless gear driven by the need to reduce entanglement of protected marine species, which will accelerate research and development of this technology. Several ropeless fishing solutions already exist but may need to be refined and improved for application in specific fisheries.[50] Pilot applications of ropeless gear are underway in North America and Australia and there are concerns around the many technical challenges to overcome and that the new technology may be cost prohibitive for the industry, though these issues will likely reduce over time.[51]

Pots on Rēkohu Wharekauri the Chatham Islands.
The cost of implementing these systems might be cost prohibitive and the types of fishing locations may limit viability in some areas. However, if further fisheries expand to using potting (such as in the case study: Potting as an alternative to trawling) there will be more ropes in the water and a higher chance of entanglement. It will therefore be even more pertinent to development ropeless gear to minimise the impacts on non-target species.
Gear add-ons will be essential to deter non-target species
In addition to the commonly used bycatch mitigation measures such as tori lines to prevent seabird bycatch, there are opportunities to innovate and develop new ways to deter non-target species from fishing activities. New devices can be added onto existing gear to deter species and protect non-target species. These include:
- Acoustic deterrents. Active sound emitters (‘pingers’) can be used to deter bycatch from regions where there are nets to avoid entanglement, but at a frequency that doesn’t deter the target fish.[52] Evidence is mixed for the effectiveness of pingers as it depends on the species, area and fishing methods.[52, 53] Though there have been limited trials, the available evidence indicates that this technology may be effective for dolphins.[54] Future developments in the area of acoustic deterrents may be beneficial but need to be precise and closely consider the benefits against the issues of acoustic pollution.
- Visual deterrents. Light-emitting devices such as Pisces can be used to deter non-target species. Nets illuminated by LED lights have been shown to be effective at deterring turtles and reducing bycatch in gillnet fisheries.[55, 56] However, these methods are not applicable to all bycatch situations. For example, high contrast panels and lights failed to reduce seabird bycatch.[57] There may be potential uses for such an approach in local fisheries and any future developments could draw on these experiences in using visual deterrents.
- Olfactory deterrents. A local study tested a bycatch reduction method suggested by a local fisher – sensory-based conservation of seabirds. Putting shark liver oil on the ocean surface behind fishing vessels was found to be effective in reducing the numbers of black petrel around the vessel and dives on baits compared to control treatments.[58] Caution is needed when considering deterrents of any kind so that the fisher is not impacted by the reduction in catch of commercially important species, as well as consideration of other unintended effects on the wider ecosystem.
- Olfactory stimulants. Olfactory baits could be developed to reduce non-target capture.[59, 60]
Uncertainty around the use of any new devices should be highlighted to decision makers.

A fisher holding a banana pinger. Image credit: Fishtek Marine.
Technological advances in fish detection could reduce fuel costs and time spent at sea
Acoustics
To reduce the burden of time and fuel spent searching for fish and associated environmental damage from unproductive activity, a high-definition omnidirectional sonar on an unmanned fishing vessel can be used to look for biomass and then send that information to land-based receivers or fishing vessels.
AI
Fish aggregate based on a range of seasonal and environmental factors. By collecting this data, advanced analytics such as machine learning (introduced in the section above, ‘AI and machine learning have the potential to increase efficiencies’) could be deployed to produce more accurate predictions for the distribution of target species.[61] In turn, this could reduce fuel costs and time at sea, as well as informing resource management. For example, the company Aker BioMarine Antarctic harvests krill from Antarctic waters. They have turned to digitalisation and machine learning to reduce their carbon footprint. Aker BioMarine have designed and deployed an unmanned, solar-powered ocean data drone called the Sailbuoy, kitted out with environmental sensors.[62] Data gathered by the Sailbuoy can be combined with historical and other information, and fed into machine learning models.
Matts Johansen, CEO of Aker BioMarine, told McKinsey & Company in December 2019, “We are only testing it [the model] now… So far, the model produces pretty good correlations. The model will learn as it gets more and more experience. We now spend about 10% of our time searching for krill. With this model, we expect that to be close to zero”.[61]
Similar projects are in development in Aotearoa New Zealand, including a proposed system that automatically integrates electronic catch and environmental reporting data and outputs continually updated maps, showing predicted locations of target and unwanted species in real time.
Advances in underwater image capture and analysis can also improve precision and targeting of desired species. For example, the Deep Vision system combines computer vision with image analysis in a robust, subsea enclosure to enable identification and measurement of fish species underwater. Developed in Norway, it is currently used for marine research trawls, but a version suitable for commercial trawl fisheries is under development.[22] Deep Vision could be combined with an in-trawl sorting mechanism so that only fish of the target species and correct size are captured, while non-target species and undersize fish are released.
Deep Vision could be combined with an in-trawl sorting mechanism so that only fish of the target species and correct size are captured, while non-target species and undersize fish are released.
There is similar research underway in Aotearoa New Zealand to develop technologies for species identification, trait measurement and individual fish identification, which may in future be able to be employed underwater to collect data and be integrated with trawl capture and release.
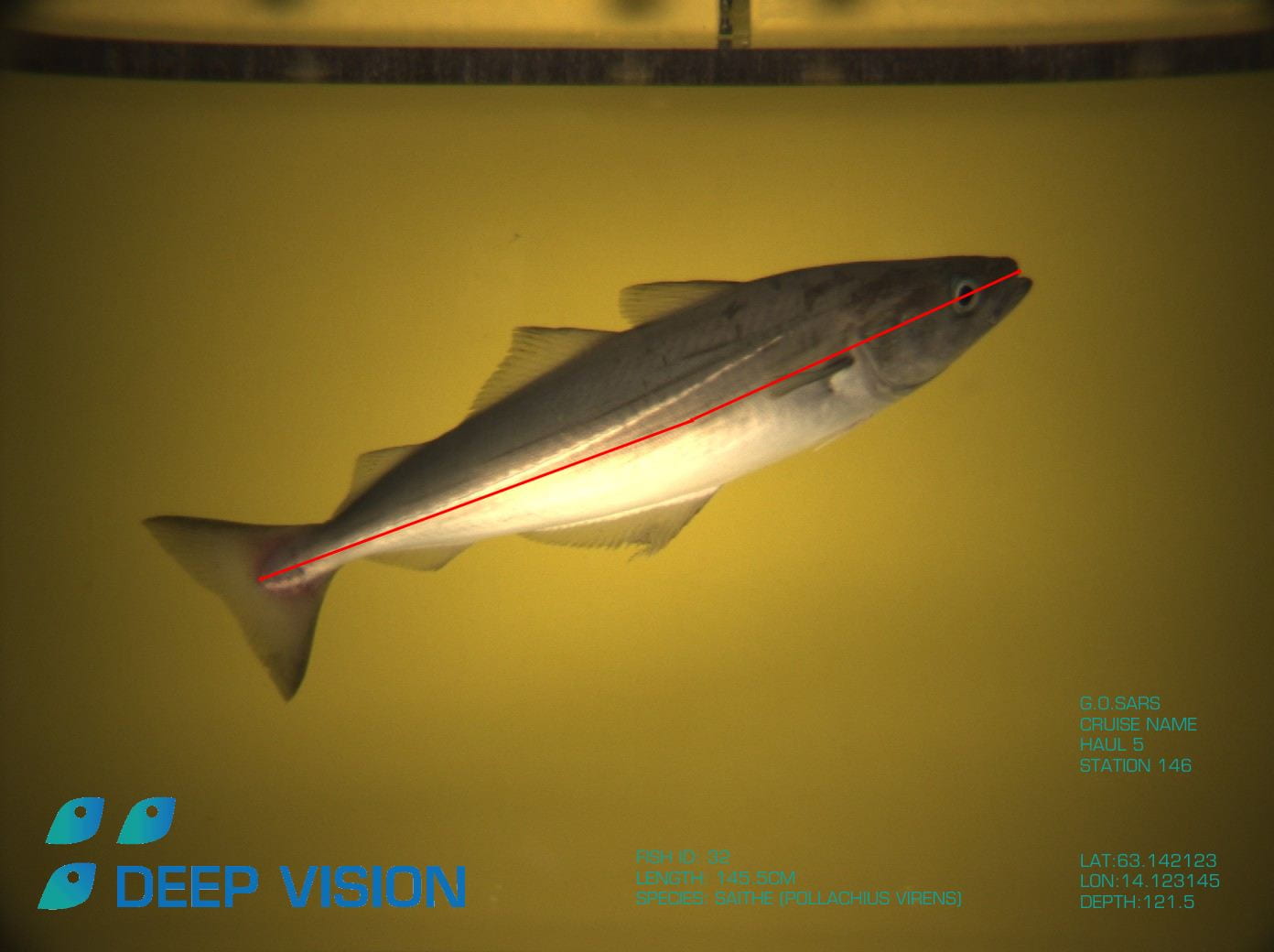
A fish with length measurement captured by the Deep Vision system. Image credit: Deep Vision.
Challenges and opportunities with gear innovation
Gear innovation has the potential to radically reduce the negative impacts of fishing through reducing bycatch, improving selectivity, enabling fishers to return unwanted catch that will survive, and eliminating the negative impact of gear on habitats.
New gear needs to be an economically viable alternative so that it is possible for large commercial fisheries companies and smaller independent fishers to adopt it. It can be costly to develop new gear and undertake studies to determine its effectiveness relative to the status quo. Further costs come from installing, optimising and using the new gear. Fisheries technological development has been found to have a positive influence on fisheries production, when guided by government investment[63] and this creates an opportunity for Aotearoa New Zealand to support our fisheries to operate in a way that is more environmentally sustainable.
New gear also needs to be practical and rooted in the needs of fishers and their practices. Part of the way to address these challenges is through enabling fishers themselves to lead the innovation, as demonstrated in the case study: A collaborative effort to protect vulnerable seabirds, and the case study: The importance of connecting fishers to researchers. The Gear Innovation Pathway (described in this case study) takes this idea and applies it to the Aotearoa New Zealand industry. While some technologies may not be affordable for smaller fishers, many invest in their own bycatch reduction technology such as using different mesh sizes, orientations and escape panels.[64] In addition to the local funding opportunities and initiatives such as the Gear Innovation Pathway, there are other programmes that Aotearoa New Zealand could take advantage of such as the WWF Smartgear Competition, which is to be revived.
True innovation needs to be assessed within a flexible monitoring framework that is not encumbered by monitoring methods inherited from old techniques
Policies exist to support innovative trawl technology, but in some instances the prescriptive requirements are actually hindering innovation rather than fuelling it. True innovation needs to be assessed within a flexible monitoring framework that is not encumbered by monitoring methods inherited from old techniques. Providing a permissive environment for innovation, coupled with a requirement for monitoring stock levels and bycatch, will encourage more industry players to adopt new techniques. Extra incentives could be provided by fast tracking the special permit process through the Enabling Innovations in Trawl Technology regulations to enable simpler trial of new gear through the special permit process, so as to reduce barriers to trialling new gear within quota. Well-designed regulation that signals the need to shift to less damaging gear could incentivise technological development in this area and expedite adoption of new technologies.
How much we fish
We could have a much deeper understanding of stock status, ecosystem health and exactly what is being taken from the marine environment to inform decisions around how much fish is allocated to commercial catch. That extra knowledge will enable an EAFM.
Here we discuss how different innovations – some already in play and others that may support fisheries management in the future – can help us to better understand the commercial fishing catch and inform management decisions around how much to catch going forward.
The technologies surveyed in this section inform our recommendations in Themes 4-7.
Computers, cameras and AI could revolutionise catch monitoring
It’s important to know precisely how much of the allocated catch is caught to ensure that future management decisions about catch allocation are sustainable. Shifting from manual to electronic methods to gather information about catch is a key way to improve accuracy. These electronic methods generate far more data than prior approaches and AI may provide a useful tool to streamline analysis so that catch monitoring can inform fisheries management in real time. In some cases, the inshore and deepwater contexts may require different technologies because of their inherent differences.
As discussed in the earlier section ‘On-board cameras are being introduced’, at-sea observer programmes tend to be accompanied by both deployment and observer effects that skew collected data.[64]
Electronic monitoring (EM) and reporting
Over the course of the 20th century, increasing recognition of impacts on marine resources prompted requirements for fishers to report their catch. This collected data could then inform management of marine resources. Now, fisheries around the world are in the next phase of modernisation: shifting away from old-school pen-and-paper logbooks towards digital solutions. As discussed earlier in ‘Electronic catch and position reporting is live’, an EM system is underway in Aotearoa New Zealand but is in its infancy.
EM with digital technology enhances the transparency, traceability and sustainability of commercial fisheries. The same data is required of commercial fishers, but a major benefit of EM is that the quality and reliability of commercially reported data is likely to improve and is verifiable, which will support better and more nimble decision making by the regulator. Though currently primarily used for compliance, it has the potential to enable more efficient and robust data collection to inform decision making and sustainable management of our ocean, as well as generating economic value for industry.
There are three basic elements of EM:
- An e-log book to report catch (replaces traditional paper-based logbooks).
- A GPS system to report location to keep track of where and when people are fishing.
- On-board video cameras to verify reported catch data and activity.
Different tools are used for different sized vessels. For example, the electronic reporting for the pāua vessel featured in the case study ‘Pāua fisheries and industry-led management’, was done on a mobile phone.
An EM system often also incorporates activity sensors that can detect when fishing gear is deployed. These sensors can be integrated with the camera system, such that the camera starts recording only when fishing is in progress. The resulting data and footage are often stored on hard drives that must be physically transferred to reviewers onshore, although some programmes are now moving to Wi-Fi, satellite or cellular network transfer and cloud storage. The footage is reviewed onshore by a trained observer – either in full, or a random portion is cross-checked for consistency with self-reported data.
It must be emphasised that simply putting cameras on boats is not the end goal for EM efforts. The promise of EM systems will be realised when the detailed data they generate is channelled into efficient and adaptive management decisions – including in real time – that enhance fisheries for all. This will include allowing these rich datasets to be mined for both commercial and environmental benefit. Some commercial fishing companies are already using their EM data to inform their own decisions, but there is potential for much wider benefit. In this way, the most successful EM programmes will have specific objectives and standards that invite collaboration and integrate with management and information systems.
The promise of EM systems will be realised when the detailed data they generate is channelled into efficient and adaptive management decisions – including in real time – that enhance fisheries for all.
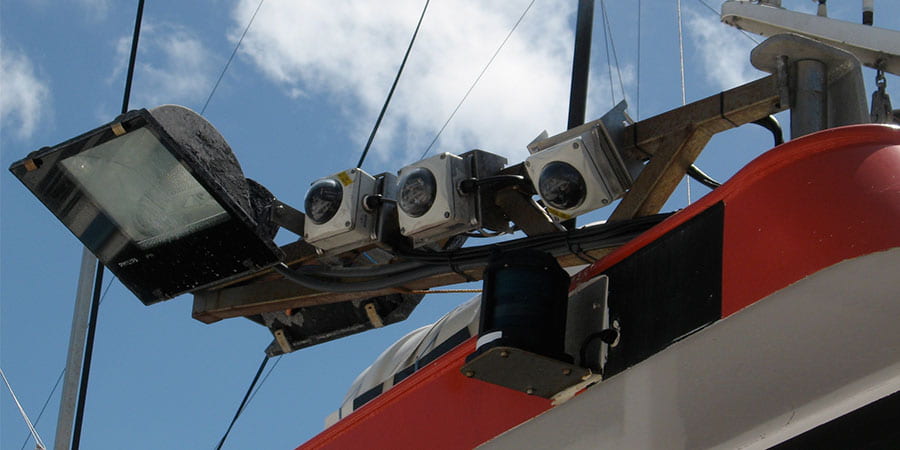
Electronic monitoring cameras onboard a fishing vessel. Image credit: AFMA.
Overseas EM programmes
Around the world, there are approximately 1,000 fishing vessels across 30 different fisheries outfitted with an EM system. This represents just 0.25% of all fishing vessels over 12 m in length.[25] The world’s first EM programme was trialled in British Columbia, Canada, in the late 1990s.[25] An industry-led initiative, EM was rolled out across the intensely competitive Dungeness crab[66] fishery to tackle catch and gear theft and to ensure compliance with trap limits.
North America continues to lead the world when it comes to EM, with the majority of comprehensive and fully implemented EM programmes based in the US and Canada.[11] In some fisheries, such as Canada’s British Columbia Groundfish Hook and Line Catch Monitoring programme, there is 100% EM coverage.[67] Others use a mix of EM and human observers. Funding also varies: some programmes initially operated under co-funding arrangements between industry and government, before transitioning to industry-only funding.
In the US, pre-existing at-sea observer coverage influences the receptiveness of a fishery to EM.[25] Fisheries with higher observer coverage see a cost benefit to switching to EM, whereas fisheries with little observer coverage may incur higher costs by implementing EM.
Across the Tasman, the AFMA runs an EM programme with coverage across four fisheries.[68] Coverage is expected to expand into other fisheries over the next 5-10 years.
Barriers and benefits
Video monitoring is the most challenging component of EM to implement – but for the most part, the challenges are not technical, but rather to do with people, relationships and communication. A collaborative, co-design approach is needed to develop EM programs that suit the needs of fishers and thus ensure buy-in by enabling commercial and environmental goals to be met as well as being simply a compliance tool.
A major contributor to the success of EM in Alaska was the switch from a top-down to an all-hands approach with all stakeholders at the table developing the details.”
– Dan Falvey, Alaska Longline Fishermen’s Association, National Electronic Monitoring Workshop 2016, NOAA Fisheries.[69]
Cost
There is a significant up-front cost associated with installing cameras and computer hardware and the set-up must be tailored to the specific characteristics of the vessel. This cost is especially problematic for small-scale or independent fishers. There are also ongoing costs associated with data storage and transfer that are non-trivial. Cost varies substantially depending on the goals of the EM programme, the number of cameras required, the level of review required, and the amount and duration of data storage.
A 2016 report found that in the US, EM costs range from 50% to 150% of human observer costs depending on specific aims and characteristics of the fishery.[70] Generally, as fishing effort increases, EM becomes more cost effective. Observers may be retained to provide other uses on-board so the costs may actually be even larger with implementation of EM.
A 2016 report found that in the US, EM costs range from 50% to 150% of human observer costs depending on specific aims and characteristics of the fishery. Generally, as fishing effort increases, EM becomes more cost effective.
Changes in practices
The presence of on-board cameras for EM may drive a change from the portion of the industry that is misreporting and illegal practices to more accurate reporting and changed fishing practices. EM may also require fishers to alter their workstream in ways that may add labour and costs. For example, fishers may need to present their catch to the camera view, slowing down the sorting process.
Privacy
Many fishers feel that video monitoring is intrusive and that such surveillance represents a distrust in them from government.[71, 72] Guidelines for the use and retention of EM data, especially imagery, must be clearly defined and communicated: who owns and has access to the data?
Numerical data is hard to manipulate but video images and pictures can be altered and misinterpreted… one image taken out of context could negatively impact a fisherman or the entire industry.”
– Mike Russo, New England Groundfish Fisherman, National Electronic Monitoring Workshop 2016, NOAA Fisheries.[69]
Suggested uses of drones to monitor fishing effort, including the two hypothetical case studies presented in a 2019 paper involving using drones to monitor recreational fishing effort and use of public lands,[73] also raise privacy and ethical issues that must be considered if unmanned autonomous vehicles are used for human surveillance purposes – whether of the general public or of commercial fishers.
Automated and AI solutions are being investigated to deal with this vast amount of data. Until these technological solutions eventuate, EM analysts should be trained to an assured standard to ensure reliability of and confidence in datasets produced.
Data review
Video monitoring also creates large volumes of data that must be reviewed – either in full, or a random selection to corroborate self-reported data. The amount of data to be reviewed, and the speed of review, depend on the objectives of monitoring – for example, if the aim is to monitor rare events such as seabird captures, it is likely 100% of the footage will need to be reviewed, but can be watched at higher speed.[74] Automated and AI solutions are being investigated to deal with this vast amount of data (see section ‘AI and machine learning have the potential to increase efficiencies’). Until these technological solutions eventuate, EM analysts (who may be former on-board observers) should be trained to an assured standard to ensure reliability of and confidence in datasets produced.
Demonstrating business benefits beyond compliance
Without the camera, my information is viewed as anecdotal to managers but a camera makes the observations more substantive. This gives fishermen power that they did not have before.”
– Mike Russo, New England Groundfish Fisherman, National Electronic Monitoring Workshop 2016, NOAA Fisheries.[69]
Some limitations compared to what observers can achieve
The additional scientific tasks that observers undertake on board a fishing vessel (e.g. tissue sampling or otolith extraction) cannot be replaced by EM. There may also be some limits to what EM can identify compared to an observer where features are not discernible with current technology, though this may improve over time.
Benefits
Although EM is an important tool for enhancing compliance with regulations, it has other less obvious benefits. EM can also:
- Ensure all fishers are operating on a level playing field.
- Empower fishers by providing concrete evidence that can back up their assertions in conversation with regulators and the public, building trust (see case study: Livestreaming commercial fishing catch).
- Improve quality of life at sea, by removing the necessity for an on-board observer who requires a berth, food etc. or, if observers are retained, expand monitoring of ecosystem health via tracking non-commercial index species.
- Verify claims about sustainability and traceability, allowing fishers to market an eco-certified, net/hook-to-plate product that is higher-value.
- Enable more flexible management of fisheries (discussed in section above: ‘Changing fisheries demand nimble and responsive decision making’). For example, identifying individual vessels with issues, meaning fishery-wide area closures can be avoided or scaled back. Fisheries managers can switch to more targeted management measures (e.g. fleet-wide, individual trip or vessel caps).
- Allow analysis of the product handling process, with a view to enhancing quality and value of the catch.
- Enable skippers to monitor activity on the vessel from multiple camera views, which has both operational and safety benefits.
- Monitor whether fishers are using the best practice mitigations (defined in regulations and the new National Plan of Action Mitigation Standards).
- Possible future benefits could be achieved through linking EM data with other real-time data to inform fishers about the environment to mitigate risks and/or enhance fishing effort.
Examples of these benefits in action are highlighted in the section ‘Dynamic ocean management will help protect non-target species in real time’, and the case study: EcoCast – an app that can help fishers decide where to fish.
Image processing
Improved computing can also enable rapid processing and analysis of images. This has a range of potential applications in fisheries and the information gleaned from it can inform fisheries management. Awalludin et al.[75] reviewed image processing techniques for fisheries, listing the following applications: fish classification, counting and abundance, fish weight and length, fish tracking, fish disease, fish tissue properties, and fish habitat identification.
The marine environment can be very challenging for computer hardware – with issues relating to power, access to the internet, and generally rough conditions. Sea-going hardware needs to be robust and resilient. Schoening[76] describes the development of a mobile sea-going high-performance computing cluster (ShiPCC), robustly designed to operate with electrically impure ship-based power supplies and based on off-the-shelf computer hardware. The SHiPCC units are envisioned to generally improve the relevance and importance of optical imagery for marine science.
AI has potential to streamline review of EM
There have been some small pilot projects related to AI and fisheries in Aotearoa New Zealand and overseas, with more currently in development.
EM can help generate reliable and transparent fisheries data at a lower long-term cost than on-board human observers. However, this information still needs to be sorted and analysed. Manual review of video footage is time-consuming and costly.[77]
Instead, we could use AI to review video with a combination of computer vision and machine learning to count and measure fish and identify species. This tech has the potential to make EM more efficient and cost-effective[25] and is being actively pursued by officials in Aotearoa New Zealand.[78]
The winning entry was able to identify five out of seven target species at 90% accuracy. Its counts were accurate to within one fish 83% of the time and average measurement errors were just below 2%.
In 2017, The Nature Conservancy collaborated with the Gulf of Maine Research Institute to crowdsource AI solutions to the fisheries video review problem.[79] They offered a first prize of US$50,000 for the best algorithm, attracting 620 entries. The winning entry was able to identify five out of seven target species at 90% accuracy. Its counts were accurate to within one fish 83% of the time and average measurement errors were just below 2%.
While this may seem promising, scaling up AI for video review remains problematic. It is difficult to design a single AI solution that works across different fisheries and vessels, each with a unique set of species and environmental conditions.
But even if a machine learning system does not match human performance, it can still expedite the review process.[14] Less accurate programmes can weed out irrelevant data or perform rudimentary sorting, making human review more efficient (and interesting).
Similar systems can be used for streamlining marine research. To address a backlog of imagery data collected by NOAA, the fisheries division of NOAA partnered with Kitware Computer Vision Inc. to develop the Video and Image Analytics for Marine Environments (VIAME) software.[80] VIAME has been used in underwater fisheries surveys, streamlining analysis of still and moving images and resulting in significant cost savings.[22] It can detect scallops on the seafloor (similar to the work profiled in the case study: Scallop surveys and harvest), track and classify reef fish, and count and classify seal and sea lion species from aerial surveys.
… scaling up AI for video review remains problematic. It is difficult to design a single AI solution that works across different fisheries and vessels, each with a unique set of species and environmental conditions.
Technical and analytical advances will help stock assessments
Innovations can improve understanding of stock status and structure to inform accurate catch limits. As discussed in ‘Commercial fishing has impacts on target species sustainability’, there are well-established methods to undertake stock assessments that are applied in Aotearoa New Zealand’s fisheries, to some but not all commercially fished stocks. There is significant room for improvement and new tools and technologies can augment the current approaches. Ensuring there is adequate data and information to achieve annual stock assessments of every commercially fished species by 2040 would go a long way to support the sustainable management of these fisheries. In order to meet this target, a broader suite of scientific approaches will need to be drawn on.
Ensuring there is adequate data and information to achieve annual stock assessments of every commercially fished species by 2040 would go a long way to support the sustainable management of these fisheries. In order to meet this target, a broader suite of scientific approaches will need to be drawn on.
Here we outline how three scientific approaches – genetic technologies, biochemical analyses and acoustic technologies – could add to our knowledge base on the health of fish stocks and support more informed fisheries management. Though the technologies themselves are not new, innovative applications, decreasing costs and improvements in analytical capabilities will render these tools invaluable for fisheries scientists to assess the sustainability of a fishery in the years to come.
There is a consensus that no single tool will address all of the questions on fisheries/ecosystem management, instead a toolkit approach will be needed whereby the right suite of tools are selected to address the specific fisheries question. The integration of new methods/tools into fisheries monitoring can disrupt long term data collection approaches so there needs to be a fine balance of if and when to integrate new approaches as the implementation of new methods often means stopping another form of data collection.
Genetic technologies
Although genetic technologies have been used in fisheries science since the 1980s,[81, 82] they have only recently started to be actively incorporated into the management of fisheries for some of the key commercial species.[83] Since the first use of population genetic characterisation for stock assessment, there have been remarkable advances in the field of genetics with the technological capabilities (notably, next-generation sequencing technologies or NGS) increasing at rapid pace and cost decreasing at a similar rate. The most common genetic technology that is used on wild fisheries is population genetics whereby the genetic diversity, population ‘health’ and interconnectedness of population can be determined with high precision. Historically, most applications use microsatellite markers that profile genetic information at less than 20 specific data points. More recently, NGS approaches have been used that can capture thousands of data points and capture much more nuanced data on population structure, sex ratios and migration.[82] Furthermore, recent epigenetic technologies are starting to use DNA methylation data to determine age of an animal – a technique that has been used successfully on whales using minimally invasive biopsies.
Genetic profiling, genetic mark/recapture, fish diets, epigenetic ageing, fish microbiomes and eDNA therefore have largely untapped potential that is not being capitalised on in the active management of fisheries across Aotearoa New Zealand. This was recently highlighted at a Fisheries New Zealand workshop on the utility of genetic analyses.[84] Even since this workshop fisheries-based genetic tools have advanced. In the case of land-based farming, genetics has been a key tool in active management of farms including stock selection – the industry has been at the leading edge of applying genomic technologies in practice. Wild fisheries could look to the application of genetic technology in aquaculture and agriculture for inspiration and learn from their experiences.[85, 86]
Genetic technologies may be able to replace conventional methods or augment other methods to improve outcomes in fisheries management (eDNA technology is covered below and appendix 12: Methods and applications of genetic technology in fisheries, has further detail). Several genomic applications can provide fundamental data to support the sustainable management of fisheries. They can be used to:
- Identify and assess fisheries stock structure and connectivity. Genetic data can be used to quantify the number of genetically discrete and thus reproductively isolated populations, and how related different populations are, and help us understand the geographical area over which a stock resides. The ability to genetically sex individuals is also useful in understanding population structure and sustainability. The data can also be used to measure the extent of movement and sharing of individuals between the populations. This information could inform decisions around whether genetically distinct populations need to be managed in separate stocks.[87]
- Resolve mixed-population fisheries. Genetic markers or whole-genome sequencing (WGS) can be used to delineate populations accurately, identify source populations, and determine the percentage of fish that breed separately but are exploited as one stock. This information can facilitate appropriate harvest strategies by season (see case study: Real-time genetic management of a marine fishery). This information could be used to assess, and where necessary redraw, boundaries of QMAs to ensure these align with biological stocks to enable more informed management.
- Better understand population demographics and dynamics. Genetic information can be used to estimate effective population size (as a ‘window’ to estimate of census population size), genetic diversity and mortality rates, and track changes in abundance through time, including prior to commercial fishing. This can be broken down into year classes if age data is available and thus can provide insights into recruitment dynamics. This information can help us better understand evolutionary responses to fishing,[88] which may inform management choices (see case study: What does ancient DNA tell us about the snapper population?). Genetic information can be gathered at a population level or an individual level. Data on genetic diversity, parent-offspring relationships, sibling relationships and sex ratios can provide population-level information.[84] Genetic tagging of individual fish (as an alternative to physical tagging) can provide information about abundance, population biomass, and growth as well as movement behaviour, and how these change through time (see case study: Genetic tagging to understand bluefin tuna population dynamics). Improvements in technology and analytic techniques mean these estimates are improving. Genetic data can also be used in tandem with other data on maturation, size-at-age and biomass to improve our understanding of and ability to monitor fisheries-induced evolution.[89]
Barriers to using genetic technologies in fisheries and possible solutions
While genetic technologies show a lot of promise for application in fisheries management, several barriers currently limit the ease of application in an industry setting. Some could be addressed in the present, while others may require further work to support uptake of these technologies. Collaboration and education between researchers, industry and communities to continue this trend of building up DNA databases and reference genomes will help to interweave genetic tools more seamlessly into the fisheries management toolkit.
Lack of genomic reference material
The success of many genetic applications in fisheries management can be streamlined and improved with appropriate reference and baseline data. In an ideal case, core resources of the species in question would be compiled to ensure optimal data capture and resolution (e.g. a reference genome).[90] However, progress can also be made without the key resources. For example, spatial sampling of a species could take place without such a priori Ideally, however, reference genome assemblies are compiled and single nucleotide polymorphism (SNP) chips developed, particularly when starting long-term research studies or large projects, as time and data quality benefits from these resources would then outweigh the initial costs of setting these up.
Doing so requires local efforts to support our local commercial fisheries sector. Some commercially significant fisheries species have had reference genomes developed, including snapper and trevally. Tarakihi and blue cod are underway. Some protected fish species and marine mammals have also been prioritised through conservation efforts, including great white shark, whale shark,[91] spine-tailed devil ray,[92] oceanic whitetip shark,[93] basking shark[94] and bottlenose dolphin/terehu.[84, 95] However, for the vast majority of New Zealand fisheries species no genomic resources exist at this stage. Collaboration and education between researchers, industry and communities to continue this trend of building up DNA databases and reference genomes will help to interweave genetic tools more seamlessly into the fisheries management toolkit.
Perception that genetic studies are expensive
Costs have been reducing rapidly for genetic sequencing, including newer genomics (NGS) approaches, but people often think these technologies are prohibitively expensive.[82] Depending on the genome size and depth of the genomic data required, one sequenced individual typically costs between NZ$20-40. For spatial analyses one typically aims to sample 30-50 individuals, and meaning that the costs per population sample are around NZ$2,000. It is more expensive to generate the reference genome (currently NZ$20-30,000 for the sequencing) and baseline work (e.g. developing a SNP chip panel) than to undertake the ongoing, routine sequencing.
For genetics studies designed to address many of the more important questions for fisheries management, this perception may often be correct; however as costs are expected to keep declining, this may change in the future. Improved communication of the costs and benefits of such approaches and sharing examples of successful implementation will be important to increase uptake for appropriate applications throughout industry.
Some commercially significant fisheries species have had reference genomes developed, including snapper and trevally. Tarakihi and blue cod are underway.
Lack of experience integrating genetic data into decision-making processes
Depending on the application and findings, genetic data may provide key information to inform practices or it may have smaller weight in the decision-making process. It has been reported that the industry has negative perceptions around genetics results not being important.[82] Pilot studies to address these issues may help to iron out how new information gleaned from genetics studies can feed into fisheries management decisions. Upskilling of staff in management positions is needed and a good dialogue between scientists and managers. There needs to be awareness of data that is available to inform decisions and ensure it gets to the decision makers and they know how to integrate it. The recent high-profile use of genomics in the COVID-19 response may have helped to normalise the use of genetic data in real-time decision making. Greater training and education surrounding the utility, cost and benefit of genetic tools is needed across the sector.
Genetic tagging can be invasive
The invasive nature of taking biopsies for genetic tagging can be risky depending on the methods needed, making it difficult to gain permits to use this approach. Using specific approaches for taking tissue biopsies for different species and refining these further to reduce risk can address this issue. For example, using biopsy hooks for samples collected at depth as this is associated with negligible mortality. This is particularly true for deepwater species that cannot be simply brought to the surface alive. Skin swabs have been demonstrated as a viable alternative to sample fish for genetic studies.[96, 97]
Need to consider genetic data rights
In Aotearoa New Zealand, genetic data rights and interests need to be factored into any genomic studies. Genomics and data sovereignty are of particular significance to Māori and in marine research the impact on taonga species should be considered.
The recent high-profile use of genomics in the COVID-19 response may have helped to normalise the use of genetic data in real-time decision making.
Using genetic technologies in Aotearoa New Zealand’s fisheries
The use of genomic technologies is not commonplace in Aotearoa New Zealand fisheries management, but several workstreams are currently underway that will pave the way for such applications (see appendix 13: Genetics in fisheries in Aotearoa New Zealand). Building on the efforts of the Fisheries New Zealand workshop,[84] researchers, industry, iwi and community should work together to address barriers, prioritise applications based on sustainability concerns and economic/cultural value. Feasibility studies which aim to determine where using genetics could be a cost-effective approach to inform sustainable management of our fisheries. We can also draw on the experiences of other countries who are beginning to integrate genetic technology in fisheries management, including Norway (see case study: Real-time genetic management of a marine fishery), Australia (see case study: Genetic tagging to understand bluefin tuna population dynamics) and the US (see case study: Managing great white shark conservation through eDNA). Because the application of genetic technologies in fisheries management is in its relative infancy, there is opportunity for local work to push the frontiers in this space, especially considering the strong international scientific footprint that Aotearoa New Zealand has in the areas of bioinformatics and phylodynamics.
To maximise on the potential of these applications, efforts need to be made to ensure that the various samples being collected now can be repurposed for genetic studies. For example, marine mammals that wash up on shore, become stranded, or are caught in trawls usually have small tissue samples taken. These are stored by various Aotearoa New Zealand institutions, often in an ad hoc way in university or museum freezers. However, some samples may be compromised due to poor handling and processing. The animals that strand may not be fully representative of the genetics of the population, which also needs to be factored in when using such samples. There is an emerging need for a more centralised repository of tissue samples and environmental samples (akin to a biobank). Such an initiative would have to play close attention to issues of data sovereignty and sample use especially in the case of taonga species.
Increasing demand for these technologies will also require more local capacity and capability to do high-throughput sequencing and store data. These efforts would need to be connected to other efforts within the fisheries sector to aggregate and use data to inform fisheries management decisions. There are also initiatives underway across Aotearoa New Zealand to familiarise communities and iwi with genetic technologies in environmental applications, these include the Wai Tūwhera o te Taiao – Open Waters Aotearoa initiative led by the EPA, and the Lakes380 and Marine Biosecurity Toolbox led by the Cawthron Institute.
Biochemical technologies
Analysing the chemical properties in fish is a valuable tool that fisheries scientists have used to inform fisheries management (as shown in this case study).[98] Used routinely since the 1980s, the approach traditionally relied on taking otoliths (a structure in the inner ear of the fish) and performing microchemical analyses on these. More recently, similar applications have been applied to other structures in the fish, including scales, fin spines, fin rays, eye lenses and the skeleton, and expanded to analyse a broader range of chemical elements.[98]
These methods can be used to measure concentrations of diagnostic molecules, which tell us about biological processes. For example, the concentrations of specific chemicals in bone relates to the age of the organism.[99] We can also infer details about an organism’s particular environment or diet based on the chemical properties present in a sample. For example, the ratio of nitrogen to carbon and sulphur can be a proxy for trophic level.[100]
Microchemical analyses are very powerful tools to use in fisheries science but they don’t necessarily work every time. The extent to which various questions about a fish’s environmental, ecological and life-history changes can be answered using microchemical analyses depends on the specific species, structure, and available technology.
In order to apply these techniques to a species we first need a thorough understanding of its species-specific biology (e.g. details of bone remodelling and collagen turnover) and to validate the method in that species. For some species, these knowledge gaps may need to be filled before microchemical techniques can be used to their full potential and premature use of the method can results in a lack of trust in the data.
Different structures within the fish have varying potential to address questions about ageing, migration and diet because of their chemical and physiological differences.[98] There are also different requirements for how to mechanically and chemically process each structure. Depending on the structure, the process may be lethal. This is a particularly important consideration for taonga or vulnerable species, and efforts to improve and expand techniques to focus on structures that are non-lethal and minimally invasive will increase the possible application of this approach.
Analytical capabilities of microchemical techniques are continually improving and researchers are now able to obtain high-resolution chemical profiles across diverse marine species, which could help to inform fisheries management decisions. As technology advances further, these techniques are likely to become more precise, the range of structures able to be analysed will expand, and new chemical signatures that document other changes in the life history of the fish may be uncovered. Future applications could also look at using multiple complementary structures and techniques to address limitations in interpretation and causes of chemical shifts that come with using only one method (e.g. linking with genetic markers, see ‘Genetic technologies’ section above).
Currently, microchemical analyses of fish are able to inform:
- Stock assessment and delineation. The chemical signatures of otoliths or other structures can identify and delineate fish originating from distinct geographical origins to classify fish stock units, but are currently best used as part of an integrated approach with other markers.[101]
- Migration patterns. Chemical signatures can show where the fish originated, where it moved and when, which has informed understanding about Atlantic salmon’s[102] migratory patterns.[103] These techniques have also been applied to connect larvae from aquaculture to restoration of bivalve reefs in the wild.[104]
- Age and growth rates. In addition to the traditional use of otolith structure to assess age, various chemical signatures can be used to determine the age of a fish and other details around age at maturity to inform management decisions, as demonstrated in studies on orange roughy.[105]
- Dietary patterns. Researchers can assess diets and determine trophic level based on chemical signatures by looking at amino acids to reconstruct dietary patterns and shifts over time.[106, 107]
Acoustic technologies
Since standard GPS signals do not work underwater due to radio waves breaking down rapidly in liquids, most underwater positioning systems rely on acoustic signals. Acoustic technologies have been used in fisheries and marine research for over 50 years and already play a role in informing fisheries management in Aotearoa New Zealand.[108] The approach is not new, but innovative acoustic technologies are under continuous development and have significant potential to support more sustainable management of our fisheries through existing and novel applications.[109]

An echogram of acoustic marks for krill swarms in the Ross Sea. Image credit: NIWA.
Acoustic technologies can be broadly divided into active and passive methods. Active methods rely on sending out sound underwater to gather information. A common approach is using a tool called an echosounder to send a pulse of sound down to the seafloor via an underwater transducer. If the pulse encounters something along the way, it is reflected back as an echo and converted to electrical energy via the transducer. The time it takes between sending out the pulse and receiving the echo tells us how far away the organism is. The echo is displayed as a 2D picture known as an echogram and the details can be used to infer the types of fish or other organisms in the area.
Originally, the technology was only able to operate at a single, discrete frequency. This limited resolution and range and therefore what could be detected. Advances led to the use of multiple, discrete frequencies at once to simultaneously measure different groups, where groups could be distinguished based on their biological or acoustic properties. A further development involved moving from a discrete burst to a continuous response around a central frequency which provides greater information and better resolution of the data, so it is easier to discriminate between species. These are known as broadband (‘chirp’) echosounders. Active devices can also send out acoustics that act as deterrents.
In contrast, passive acoustic methods are used to listen to and record sounds underwater.[110] These approaches use underwater microphones (hydrophones) to pick up sounds known to be associated with specific marine organisms, such as whales or sound-producing fish, but their application relies on prior work to identify and ‘sound-truth’ species-specific sounds through verification with other methods. Scientists can identify, record and study underwater animals using passive acoustic technologies, either in the absence of visual information or coupled with optical technologies such as underwater or surface cameras to make biomass estimates (see section ‘Underwater and surface cameras give a wider and sharper view of the ocean’). The technique provides a way to perform non-invasive, non-destructive surveys of marine life, generating information to locate fish to understand habitats, spawning times and other behaviours.
Acoustic technologies are used in a range of ways in fisheries:
- Abundance estimates. Acoustic technologies provide a non-destructive method of estimating fish densities and – with the right survey design – abundance, which can feed into stock assessments.[111. 112] Fisheries-independent acoustic surveys are currently used in Aotearoa New Zealand to estimate orange roughy, southern blue whiting, hoki, oreos, and mesopelagic fish abundance. Specialised towed acoustic systems, including deep-tow bodies and a net-mounted acoustic-optical system (AOS), have been developed by NIWA and CSIRO to improve sampling of these deepwater fish.[113]
- Species composition and distribution. Acoustic techniques can be used to locate individuals and concentrations of particular species, including during their vulnerable spawning stage.[114] This in turn allows spawning habitat to be identified, mapped and protected.[115] Researchers have applied a semi-automated process to better understand fisheries areas, such as assessing the micronekton community on the Chatham Rise,[116] using the open-source software ESP3 (see case study: Software to streamline acoustic data analysis).
In order to broaden the application of acoustics in fisheries management some key challenges need to be addressed. An initial hurdle is classifying the acoustic properties of a species before active acoustic technology can be used to monitor or study it. This relies on studies to identify the species and its target strength.[117] For some species or in particular habitats (e.g. deep water) this can be more difficult, but methods are constantly developing to improve in these two areas and validation steps are performed to ensure accurate data. Further research on the non-target effects (e.g. impacts on cetacean communication) should also be investigated before deploying the technology.
Innovations that expand ecosystem knowledge will add an extra dimension to fisheries management
In an ecosystem, nothing exists independently.[4] Fish are intimately and completely dependent on marine ecosystems for their prey and habitat. Consequently, changes in ecosystem structure and function will affect commercially important fish and fisheries.
Globally there is an increasingly shared view that steps towards EAFM are needed (see section ‘Ecosystem thinking’). Such an approach would rely on information about the health of an ecosystem to inform how the fisheries within it are managed. One of the key aims of EAFM is to move from a single-species management (i.e. deciding what the catch of species A should be without considering the status of or effect on species B, C and D) to a framework that recognises these as interacting components. Thus, increasing fishing pressure on one species may cause other species to decrease or increase and will therefore change the amount of fishing pressure these can sustainably withstand. Applying this requires a better understanding of the inter-species interactions via predation, competition and recruitment and that these are highly size-dependent (e.g. large individuals of species A may be predator of small individuals of species B and vice versa) so a food web type approach is not sufficient.
Innovative modelling approaches that draw on data from these and other techniques are changing the way that ecosystems are monitored and managed. Used alongside the catch limits, these tools offer the potential to monitor ecosystem health in a predictive and more holistic way, adding an extra dimension to fisheries management.
Use of cameras, both underwater and on the surface, can gather essential information about ecosystem health and dynamics. Other techniques introduced earlier, including genetic and acoustic technologies, can also be applied to fill gaps in our knowledge and inform EAFM. In addition, eDNA collected from the water can provide deep multi-trophic detail on the organisms that live in any given environment – from microbes to mammals,[118] as discussed below.
Innovative modelling approaches that draw on data from these and other techniques are changing the way that ecosystems are monitored and managed. Used alongside the catch limits, these tools offer the potential to monitor ecosystem health in a predictive and more holistic way, adding an extra dimension to fisheries management.
Underwater and surface cameras give a wider and sharper view of the ocean
As discussed for EM with on-board cameras, (section above: Computers, cameras and AI could revolutionise catch monitoring), camera imagery is a key, non-destructive tool for monitoring habitats and species, and improving management of the marine environment across different temporal and spatial scales. Over recent decades, our eye on the ocean has widened and sharpened, with marked improvements in camera technology.
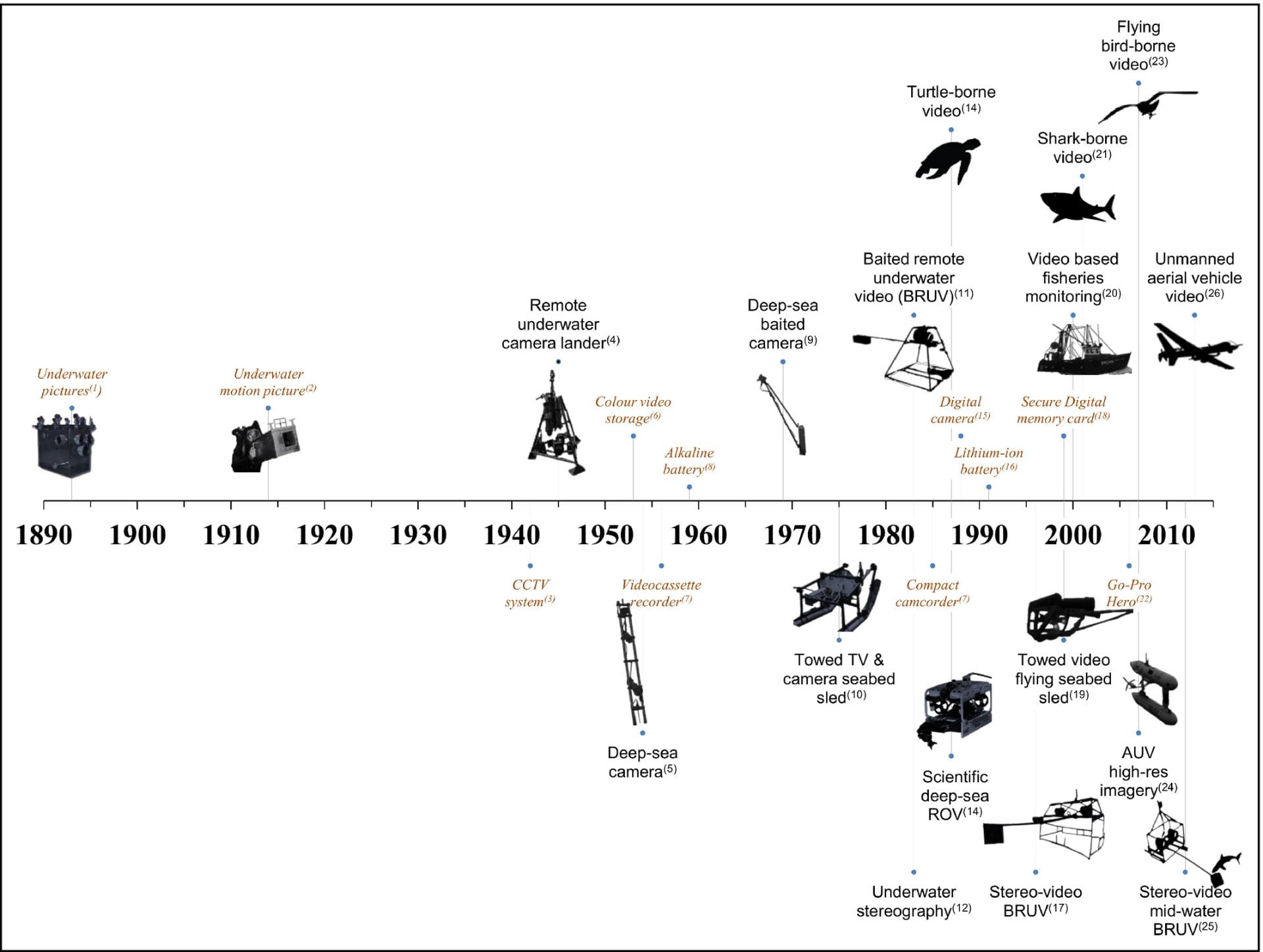
Timeline of development of cameras for studying marine biodiversity.[119]
Camera imagery is affected by water clarity and visibility issues, which are worsened by sedimentation. Converting imagery to data that can be used is often slow and laborious. Automation may be able to speed up this process, but the technology is, for the most part, still in the pilot stage. Current regulations that limit a third wire to protect seabirds are a barrier to using innovative underwater camera technology as an extra wire is needed to transmit imagery back to the boat, though special permits can be given to trial gear.[48]
This section discusses underwater and surface cameras. For discussion on satellite imagery, see the section ‘Satellite technology allows us to track marine species in detail’.
There are different types of cameras available for different applications.
Towed cameras and remotely operated vehicles (ROVs)
These allow us to collect data over large areas underwater. For example, a camera towed on a sledge on the seafloor can photograph the surrounding benthic environment, allowing scientists to estimate recovery rates and resilience of these habitats to bottom trawling and dredging.[120] The American research vessel EV Nautilus, owned by the Ocean Exploration Trust, has a number of submersible ROVs. These are outfitted with high-definition cameras and other sensors to perform biological, geological and archaeological exploration of the ocean. The expedition ROV footage is livestreamed via YouTube with commentary from on-board experts, beaming real-time discoveries into classrooms and onto smartphones around the world. We also have developed a deep towed imaging system in Aotearoa New Zealand (see case study: NIWA’s Deep Towed Imaging System (DTIS)). Some of the challenges associated with these operations, such as the need for vessels to be present to deploy them and the associated costs of that, can be addressed through the use of robotics and unmanned autonomous vehicles (see section below).
Camera traps
Camera traps can reveal the different species living in a habitat, with some designs triggering only when an animal moves into the camera’s field of view.[121] Some cameras may be baited to attract fish or other species, these are known as Baited Remote Underwater Video Stations (BRUVS).[122] These can tell us what species are present at a site and allows estimation of the length of individuals when stereo video is employed – which in turn enables understanding of biomass, population dynamics and fecundity. Some species may avoid baited camera systems, while choice of bait can affect the species that approach a camera.[119] There are challenges in scaling up the use of stereo-BRUVS because of the amount of boat space taken up by the BRUV itself and its retrieval gear. This may limit the pace of a study and increase field costs.[122]
Animal-borne cameras
Animal-borne cameras are attached directly to animals and can tell us about the behaviour, distribution and ecology of the species, especially when combined with other sensors such as GPS.[119] Animal-borne cameras can also provide a way to monitor the status and health of important fisheries habitats, such as seagrass.[123] Animal welfare must be considered as well as the potential for littering and marine pollution.
In-trawl cameras
In-trawl cameras are attached to nets or gear underwater. These can help to identify and quantify catch and bycatch (including threatened and protected species) and estimate seafloor impacts of bottom contacting fishing gear.[124, 125] They can also reveal fish behaviour, which in turn informs stock assessment and gear design. For example, flatfish in the Northeast Pacific were observed to ‘herd’ in response to a bottom trawl, and if this behaviour had not been accounted for, their stock could have been overestimated.[126] The local research underway which aims to achieve species identification, trait measurement and individual fish identification using in-trawl cameras (described in section above) highlights the potential utility of this technology in fisheries. AI developments may allow for automated recognition of species to allow for release of non-target catch.
Non-research camera imagery
Non-research camera imagery is also a source of information. Hundreds of hours of raw footage filmed in the waters around Rangitāhua the Kermadec Islands for Natural History New Zealand’s Our Big Blue Backyard series was analysed by researchers.[127] Three species new to the area were discovered. There have also been suggestions that documentary out-takes can help researchers determine how an environment might be changing over time. Imagery collected by recreational divers can also give insights, like the Ocean Sunfish Research initiative that catalogues the sunfish on the Nusa Penida reefs near Bali, Indonesia. This project determined that the sunfish present on the reefs were not Mola mola but were in fact Mola alexandrini.[128]
Right: A bump-head sunfish (Mola alexandrini) on the Nusa Penida reef near Bali, Indonesia. Imagery collected by recreational divers has advanced our understanding of these enigmatic creatures. Image credit: Albert Kang/iNaturalist (CC BY-NC 4.0).
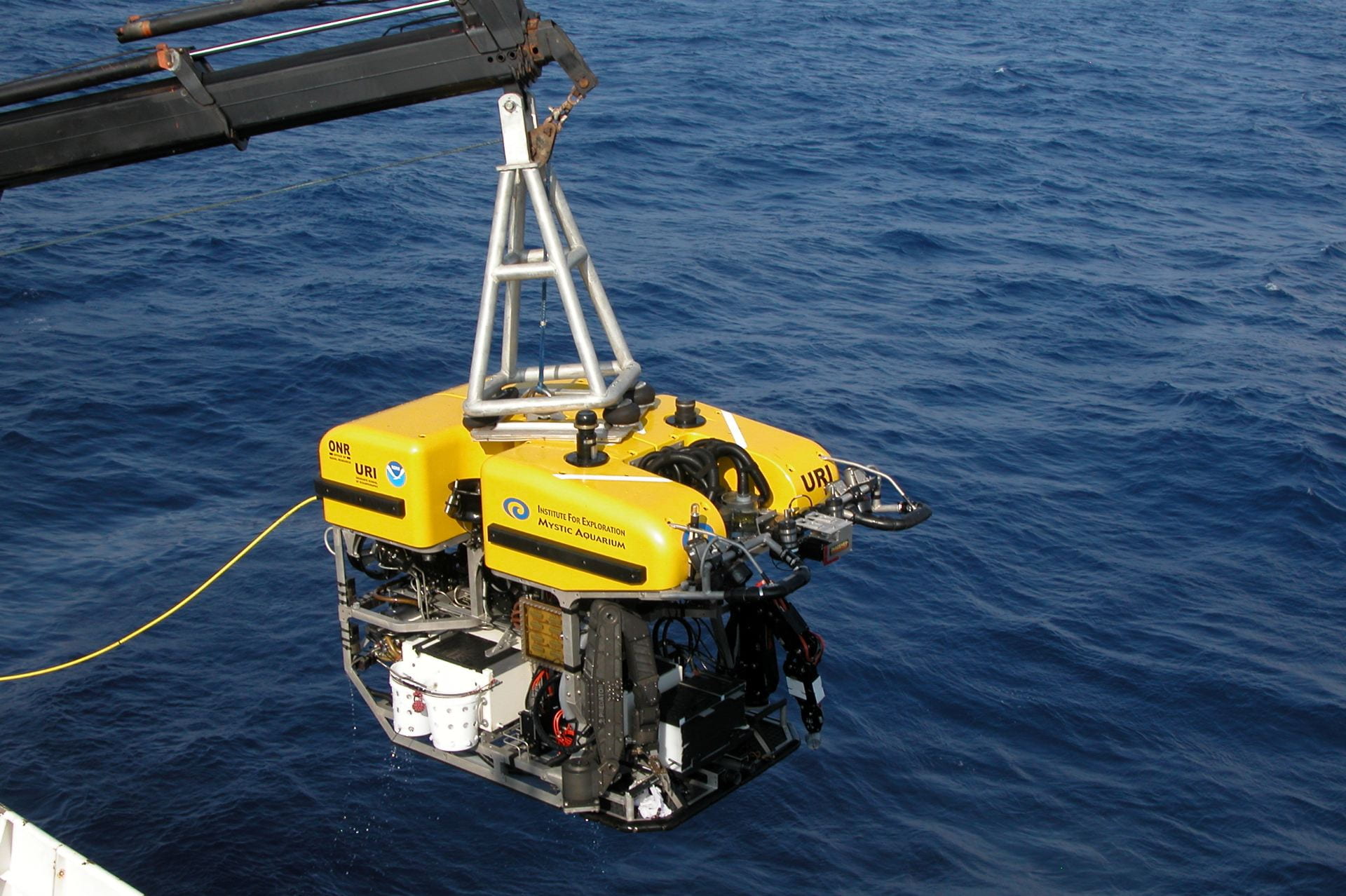
The Hercules remotely operated vehicle (ROV), one of the submersibles used by the EV Nautilus for underwater exploration and research.
Environmental DNA (eDNA) can grow ecosystem knowledge
The application of environmental DNA (eDNA) methods offers a way to monitor the complex interactions between fisheries and the environment by providing a high-level overview of community composition across any given area or depth area.[129–131] DNA is collected from the environment (e.g. filtered seawater) and diagnostic parts of the DNA (barcodes) are sequenced, in an approach known as DNA metabarcoding.
eDNA enables researchers to simply and non-invasively monitor ecosystems through species detection, determining species diversity and further details about ecosystem function, including diet, pathogens and invasive species.[132, 133] The technique can be used to detect rare or elusive species (discussed further in the ‘Genetic technologies’ section and case study on great white sharks). eDNA metabarcoding is cost effective in that it only sequences the ‘barcode’ region of the sample. To cite one example, a nine-litre water sample from the Ningaloo Reef yielded over 60 genera of fish and over 287 families of marine taxa.[134] Similar approaches are being used across Aotearoa New Zealand (see figure below). The wide biotic lens of eDNA is the method’s core strength, and the primary reason why eDNA is quickly becoming a key component of marine surveys across the globe.
eDNA enables researchers to simply and non-invasively monitor ecosystems through species detection, determining species diversity and further details about ecosystem function, including diet, pathogens and invasive species.
The eDNA approach overcomes many limitations when studying more complex biological systems, including time-consuming microscopy, extractive sampling, difficulties identifying different life stages and sexes, and cryptic species identification. In a fisheries management context, a one-year New Jersey study comparing eDNA and monthly trawl estimates were well correlated across fish species richness, composition, seasonality and relative abundance.[135]
A key strength of eDNA is that it is a non-invasive sampling method and can provide a high-level overview of genetic biodiversity, including presence/absence data, and detect ecosystem changes over time. eDNA has also been shown to be effective in detecting anthropogenic disturbances[136] and detecting rare marine taxa from seahorses[137] to sharks.[138] The hope is that in the future eDNA sampling methods will enable further quantitative precision (using absolute and relative abundance), noting that ‘catch’, acoustic and video surveys all have intrinsic strengths and weaknesses when it comes to quantitative analysis.
There are several limitation, comparative and proof-of-principle studies when implementing eDNA in aquatic environments, and these have been at the core of the >1,500 papers on this topic, see [118]. In a fisheries context, there’s potential for contamination from fishing gear, lab contamination and the vectoring of DNA by animals. Moreover, eDNA data provides no direct information on age, weight, life-stage or fecundity.[139] However, the potential for eDNA to move beyond ‘just barcodes’ to measure population-level metrics is being actively explored.[140]
The hope is that in the future eDNA sampling methods will enable further quantitative precision (using absolute and relative abundance), noting that ‘catch’, acoustic and video surveys all have intrinsic strengths and weaknesses when it comes to quantitative analysis.
eDNA methods in the ocean are still relatively new and require ongoing optimisation in many areas of the workflow to ensure sampling and analytical consistency.[133] Being able to apply these techniques also relies on species having a reference barcode in databases to match the sample – reference barcodes are much easier to generate than whole genomes (see section above) and relevant barcodes for key genetic markers (e.g. COI, 16S, 12S) exist for most fish species across Australasia. We expect these databases to continue to grow over time (underpinned by a robust taxonomic framework) making this application more powerful. Increasingly the genetics community is seeking to integrate eDNA data more seamlessly into databases with other biotic data (e.g. the Atlas of Living Australia), one key will be to make eDNA data more accessible to other discipline areas (see commentary in [141]).
Lastly, the true power of eDNA methods will come from structured time-series data that extends across seasons, years and decades – the research community is beginning to assemble just such datasets (see [142]).There is a growing need and willingness to collect eDNA samples now for future use with data, technological and analytical advances. It’s important to ensure samples are collected and stored correctly so that DNA does not degrade.[139, 142, 143]
Other genetic tests, aside from eDNA discussed above, can be applied to individuals and environments for disease surveillance and to understand disease epidemiology. Gene expression assays can be used to rapidly diagnose multiple pathogens. Techniques such as DNA metabarcoding provide an efficient means of tracking the spread of invasive species. Genetic applications can also support protection of vulnerable, protected or taonga species through better understanding of the genetic diversity in small populations, and through the discovery of genetically distinct species or stocks (how different genetic techniques can be applied in fisheries is described in appendix 13).
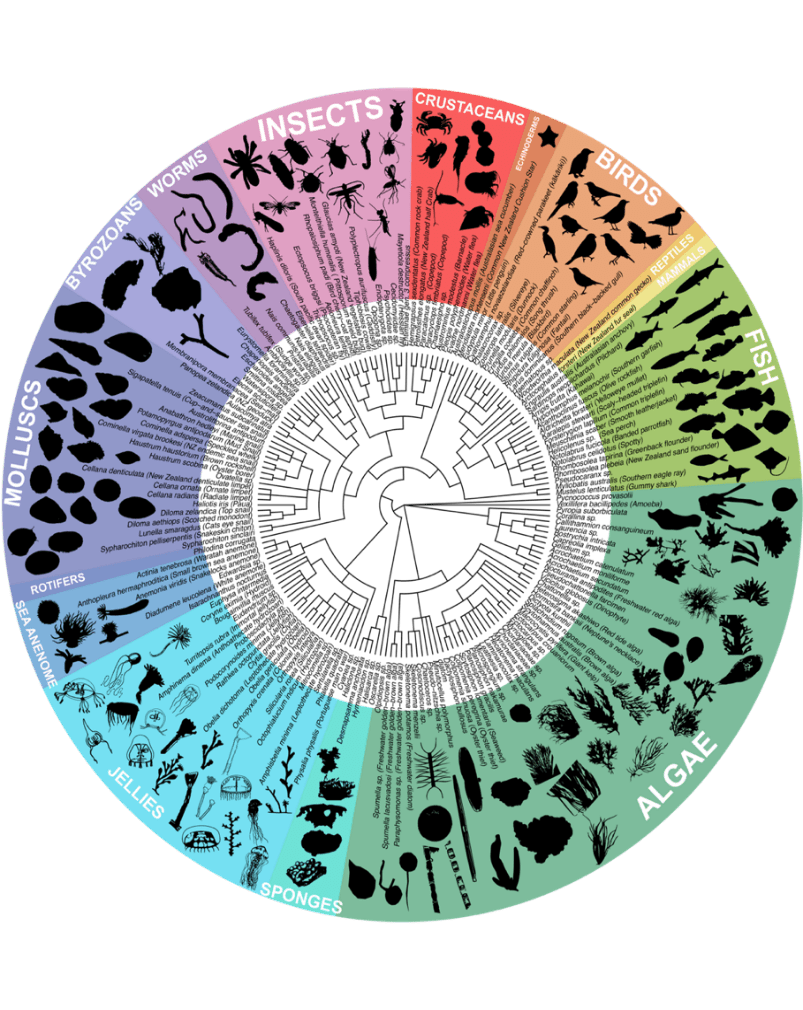
A biotic survey of Wellington harbour using eDNA metabarcoding. Such methods could play a role in baselining a variety of marine biota. Image credit: EPA/Wilderlab.
Models can support ecosystem approaches to fisheries management
Models are key tools that can be used in fisheries management to integrate a wide range of system information in a common framework.[144] Commercial fishing has long relied on modelling to determine how much to fish. These models have largely been single species focused and single-species models remain the dominant tool for informing the management of commercially valuable stocks in practice.[145] This is true for many of Aotearoa New Zealand’s major fisheries where a generalised age- or length-structured fish stock assessment model, CASAL, is used for stock assessments, though not all assessments use it.[146] Most of these models rely on the outputs from current stock assessments and often rely on assumptions that are contested (see section ‘Setting catch limits and allocating catch allowance’).
Innovative ecosystem models can support sustainable fishing by improving system understanding, identifying major processes, drivers and responses of change, highlighting major knowledge gaps, and providing a way to test management strategies before implementation.
The growing recognition of the complexity of ecosystems and need to understand dynamics within them has heralded a new era in modelling that expands beyond a single-species focus to the wider ecosystem, which aims to address the acknowledged limitations of existing models. Along the continuum of complexity for models used in fisheries management, the mechanistic models used for stock assessments sit at one end, with models of intermediate complexity forming the bridge to the full ecosystem models at the other end.[147] Innovative ecosystem models can support sustainable fishing by improving system understanding, identifying major processes, drivers and responses of change, highlighting major knowledge gaps, and providing a way to test management strategies before implementation.[144] However, they are extremely complex and resource intensive, depending on significant datasets for stocks and their environment over time.
There are a number of whole ecosystem modelling approaches of marine ecosystems and these are being increasingly used as a tool for analysis of ecosystem structure and function.[145, 148, 149] However, while full ecosystem models are desirable they are not always practical to be implemented because of data and knowledge gaps.[147] The cost of implementing full ecosystem models can also be prohibitive due to the significant data needs. By 2040, increased data and knowledge regarding our marine ecosystems will support wider application of the more complex models in the stock assessment and broader fisheries management process. Readily available and up-to-date data will also help the development, testing and deployment of more reliable models with fewer assumptions, and reduce the cost burden of running such models.
A high level overview of the different types of modelling approaches in the toolbox that can be applied, depending on available data, and some examples of these are discussed below. Additional examples are briefly described in appendix 14: Further examples of models.
Mechanistic/dynamic models
Mechanistic/dynamic models are used to inform year-to-year management decisions, such as stock assessments. The model is designed based on what we know about the ecosystem, which is simplified to the available, and often limited, knowledge. Structural assumptions and parameters are put into the model and then changes in ecosystem properties can be simulated over time, allowing for the model to predict the future but only for small changes and only if the basis of the model remains valid. The model’s output tends to be highly dependent on the assumptions about how the ecosystem functions so knowledge gaps can be limiting and the more knowledge feeding into it the better.
Casal2 is population modelling software that is used for quantitative assessments of marine populations, including fish stock assessments.[146] It can project population status into the future or simulate observations from a set of given model structures, and can allow for relationships between species, such as predators and prey. Observational data can be input from many different sources, for example removals-at-size or -age from fishing or other human impact, scientific survey, and mark-recapture data. There is flexibility in specifying population dynamics, the parameters used and model outputs – for example, for the first accepted stock assessment using Casal2, completed for New Zealand blue cod in 2020, the model assumed some blue cod could change sex (which they are believed to do, from female to male), and at the same time change their growth rate and longevity.
Mass balance models
Mass balance models such as Ecopath with Ecosim (EwE) are a type of ecosystem model that focus on trophic transfers of material i.e. the feeding of one organism on another. They act as a basis for future ecosystem modelling, for example, the development of models that are seasonally resolved, spatially resolved, and capable of being run dynamically. The model may also allow identification of sub-systems (for example, groups of interconnected species) that should subsequently be modelled in more detail.
Mass balance ecosystem models are beneficial because they force the critical assembly of a large amount of data on all components of the ecosystem in a form where they may be combined and intercompared. The model tests whether our current understanding of the ecosystem structure and function is complete and consistent. In assessing completeness, the model allows us to identify critical gaps in our knowledge, data, or approach. They formalise our conceptual model of ecosystem interconnectedness giving a quantitative model of energy flow through the system. This may be useful for suggesting system-level characteristics or properties of the system. For example, the model is used to identify key species or groups on which the system depends. Models help to identify candidate indicators of ecosystem state, which will be useful in monitoring for major changes in ecosystems over time. Aotearoa New Zealand has developed mass balance models for the Southern Plateau,[148] Chatham Rise,[150] Tīkapa Moana Hauraki Gulf,[151] Te Tapuwae o Rongokako marine reserve,[152] and Ross Sea.[153]
Size-based models
Size-based models are ecosystem models in which key life history traits such as feeding, mortality and reproduction are governed primarily by size, rather than species identity. Size based models range in complexity, from whole community models without resolved species,[154] up to multi-species models in which individual species traits are also captured and resolved.[155] Some models capture the links between pelagic and benthic organisms,[156] and some even consider the role that habitat plays in mediating predation and supporting fisheries productivity.[157]
These models predict ecosystem-level dynamics including, but not limited to: the size-structure of communities, the productivity of fisheries, the average trophic level in an ecosystem, or the maximum size of different species. The benefit of this modelling framework is that it requires significantly less data than mass-balance models, or full ecosystem models, but has the capacity to answer some of the same management questions. The reduced data need is driven by the key assumption that big organisms eat smaller organisms, thus avoiding the need for detailed diet data for all species, which is rarely known, and expensive to generate.
Size-based models have been applied to many pertinent questions in the field of fisheries science, including the impact of climate change on global fisheries productivity,[158] the trade-offs between fisheries targets and marine conservation,[155] and the impacts of habitat loss in tropical fisheries.[157, 159] Size-based models have a great potential as a management tool to support the move towards EAFM. Models are currently being developed for two key Aotearoa New Zealand fisheries, and their predictions are being validated and compared to both mass-balance and full-ecosystem models to understand which tools can answer which questions.
Models of intermediate complexity
Models of intermediate complexity (MICE) are ecosystem models that are question-driven and contain a limited number of components and ecological processes. Intermediate complexity models rely on the most important aspects with the most data. The Chatham Rise MICE model includes two commercially fished species (hoki and hake) and assesses their population dynamics simultaneously, using a functional response formulated by representing predator-prey interactions.
Full ecosystem models
Full ecosystem models are strategic models that are used to support longer-term planning. These models can include some or all of the following aspects – population structures, environmental influences on populations, non-linear species interactions, and human interactions (e.g. beyond harvest).[147] The increasing complexity of these models requires an increasing number of parameters and with that can come heightened uncertainty, until more knowledge is gained.
The increasing complexity of these models requires an increasing number of parameters and with that can come heightened uncertainty, until more knowledge is gained.
Atlantis is a modelling framework to explore ‘what-if’ type questions for marine ecosystems. It can be used to understand possible drivers of ecosystem components and states and to simulate how ecosystem components may respond to various management interventions. The model simulates the ecosystem through time, calculating each new state based on the previous state and events since. It integrates biology, physics, chemistry and human impacts (e.g. the effects of fishing) to provide an overview of marine ecosystem function. The Atlantis modelling framework consists of sub-models that incorporate both the biogeochemical components of the marine ecosystem in question and the human realm into model predictions. Atlantis modelling is a substantial task, with a single application typically taking between six months and two years to develop. Two Atlantis models have recently been constructed, one for Te Tai-o-Aorere Tasman Bay and Mohua Golden Bay and another to represent the Chatham Rise area[4, 160] – see case study: Chatham Rise is a unique fishery with consistent, long-term data. Both models have been tested and validated. Scenarios could include varying levels of climate change impact, or alternative fishing (exploitation level, spatial patterns, target species and gear changes) approaches, and single- versus multi-species maximum sustainable yields. The model does not account for seafloor damage and sensitive benthic habitats and some question whether the model is practical for wide deployment given how much funding would be required to fulfil the data needs.
Size spectrum models
Size spectrum models take body size as the most important variable determining predator-prey interactions, mortality, and growth and reproduction rates.[161–163] They then track the transfer of biomass between species and sizes as a result of these processes. This enable more realistic capturing of the way changes in one species or ecosystem component affect other components.
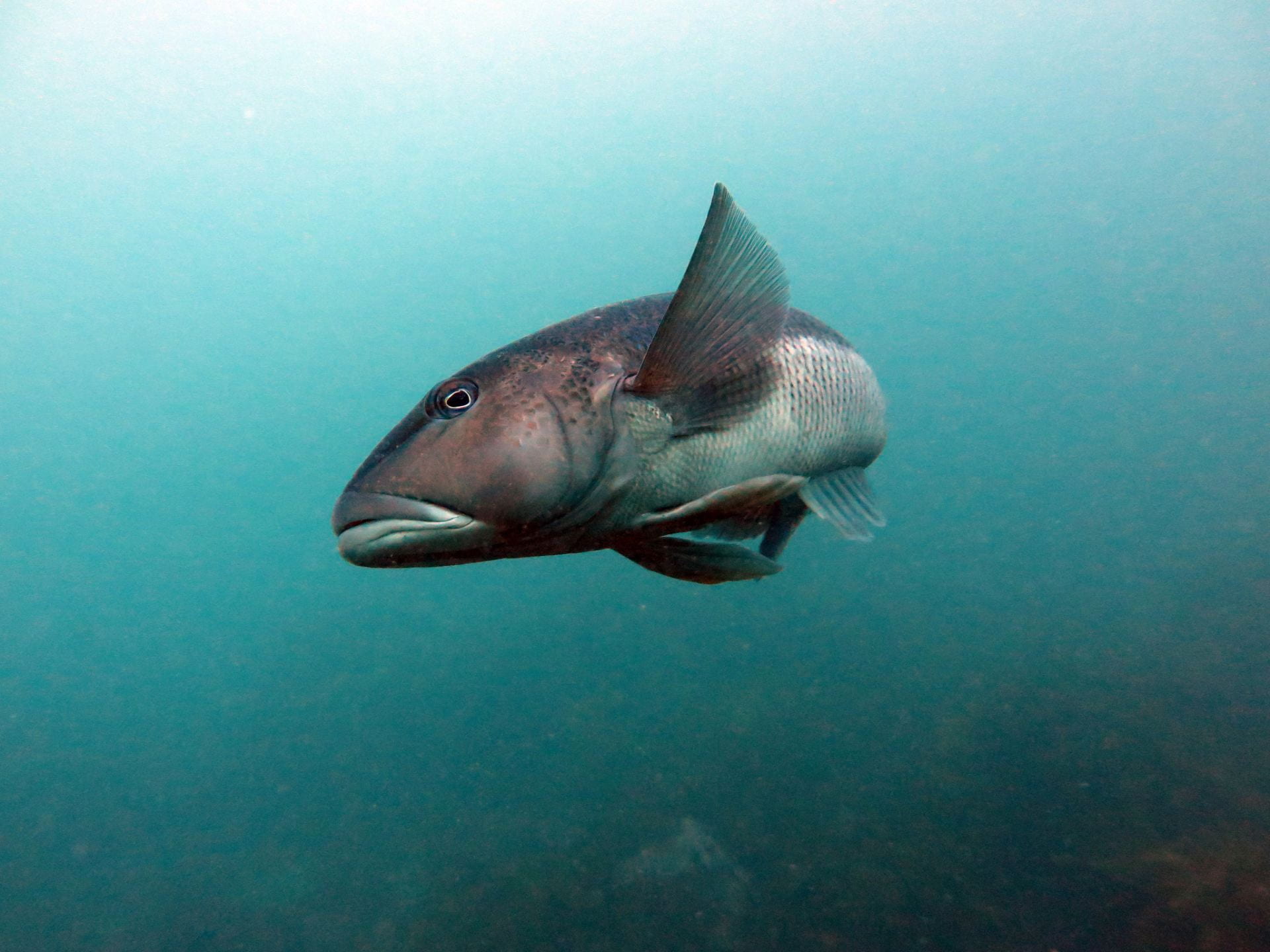
Blue cod (Parapercis colias) are able to change sex (from female to male) and this needs to be accounted for in models. Image credit: Sarah Milicich/iNaturalist (CC BY-NC 4.0).
Need for indicators
Ecosystem indicators are crucial to understand ecosystem dynamics because it is impractical to measure every species to inform management decisions. Ecosystem indicators can be physical, chemical or biological. Physical and chemical indicators are measures of the physical and chemical components of the ecosystem, whereas biological indicators (or bioindicators) refer to organisms, species, or communities whose characteristics show the presence of specific environmental conditions. For example, measuring the most sensitive species can indicate whether the rest of the ecosystem is healthy (discussed in ‘Ecological monitoring’). The opportunities to collect data in new ways (outlined above) could provide valuable indicators for ocean health and ecosystem dynamics.
Filling data gaps is essential to improve models
The reliance on modelling stems in part from many of the data and knowledge gaps discussed in ‘Challenges for the marine environment’ and in ‘Commercial fishing has impacts on target species sustainability’. Consequently, many of the opportunities to improve in these areas will improve the accuracy of modelling. Currently, a smaller number of datasets feature heavily in stock assessment models, particularly time series data on relative abundance. Some of this data is independent (such as research surveys), while other data is fisheries-dependent (e.g. CPUE). The more data we have the less assumptions need to be modelled and the more certain we can make estimates of a species or species group status and how they will respond to changes in fishing pressure or environmental conditions. Having regional models can also lead to more fine-scale knowledge to inform fishing, as is the goal in the case study: The Moana Project – Arming vessels with sensors to help validate ocean models.[164]
Currently, a smaller number of datasets feature heavily in stock assessment models, particularly time series data on relative abundance. Some of this data is independent (such as research surveys), while other data is fisheries dependent (e.g. CPUE). The more data we have the less assumptions need to be modelled and the more certain we can make estimates of a species or species group status and how they will respond to changes in fishing pressure or environmental conditions.
Issues with overreliance on modelling and a lack of validation, particularly in stock assessment, are well-acknowledged and are not limited to Aotearoa New Zealand … At present, the data required to develop robust, dynamic predictive ecosystem models is lacking. Long-term data collection at multiple trophic levels is needed to use these structural food web models to project ecosystem changes into the future in response to climate change and human activities such as fishing.
Validating models
There are opportunities to improve our modelling by strengthening validation and data inputs. Issues with overreliance on modelling and a lack of validation, particularly in stock assessment, are well-acknowledged and are not limited to Aotearoa New Zealand.[165] New research at NIWA is developing methods to use stable isotope analyses of tissue of multiple species to validate and tune food web models to reduce uncertainty and improve reliability. At present, the data required to develop robust, dynamic predictive ecosystem models is lacking. Long-term data collection at multiple trophic levels is needed to use these structural food web models to project ecosystem changes into the future in response to climate change and human activities such as fishing.
Use going forward
- Innovative modelling and spatial analysis tools for identifying vulnerable and sensitive habitats allow for assessment of spatial management configurations and trade-offs to maximise biodiversity protection benefits and minimise costs to bottom trawling fisheries.
- It’s essential that all parties have a clear understanding of the strengths and weaknesses of a model at all stages of development, and the level of uncertainty where the model can be used for fisheries management decisions.
- Models need to be fit-for-purpose, deployed in stages for continuous refinement, and able to be effectively communicated so that people understand how the evidence is generated and the level of uncertainty.
- Models that can be modified and applied to new questions will be most beneficial to support a responsive management system.
- Models can support the basis of multispecies harvest control rules.
- Long computer run times usually preclude more complete exploration of the parameter space but as computing capacity increases this might be possible.
- These models should be fully integrated into the decision-making process and always ground-truthed with real-life fisheries experience.
These issues are pertinent to recommendations in Themes 5, 6 and 7.
Where and when we fish
There are a number of factors that determine where and when fishing can occur, including weather, market opportunities, land-based impacts such as sedimentation, and any regulatory constraints.
With a specific focus on improving the sustainability of fishing operations, here we consider how technology can help refine where and when fishing takes place to reduce the impact on other species and habitats. The target species of commercial fishing operations often overlap with protected species and habitats and other target or non-target species. By knowing where protected or threatened species are, interactions can be better managed. Likewise, knowing which habitats to avoid and when will provide benefits to commercial fisheries stocks and the ecosystem as a whole.
Understanding the interactions of fisheries with species and habitats is important for three reasons:
- We can better estimate the magnitude of the impact of fishing on threatened species, and therefore better assess the conservation status of these species,
- We can understand the nature of the interactions and devise solutions to mitigate these impacts, and
- We can determine which habitats to avoid and when.
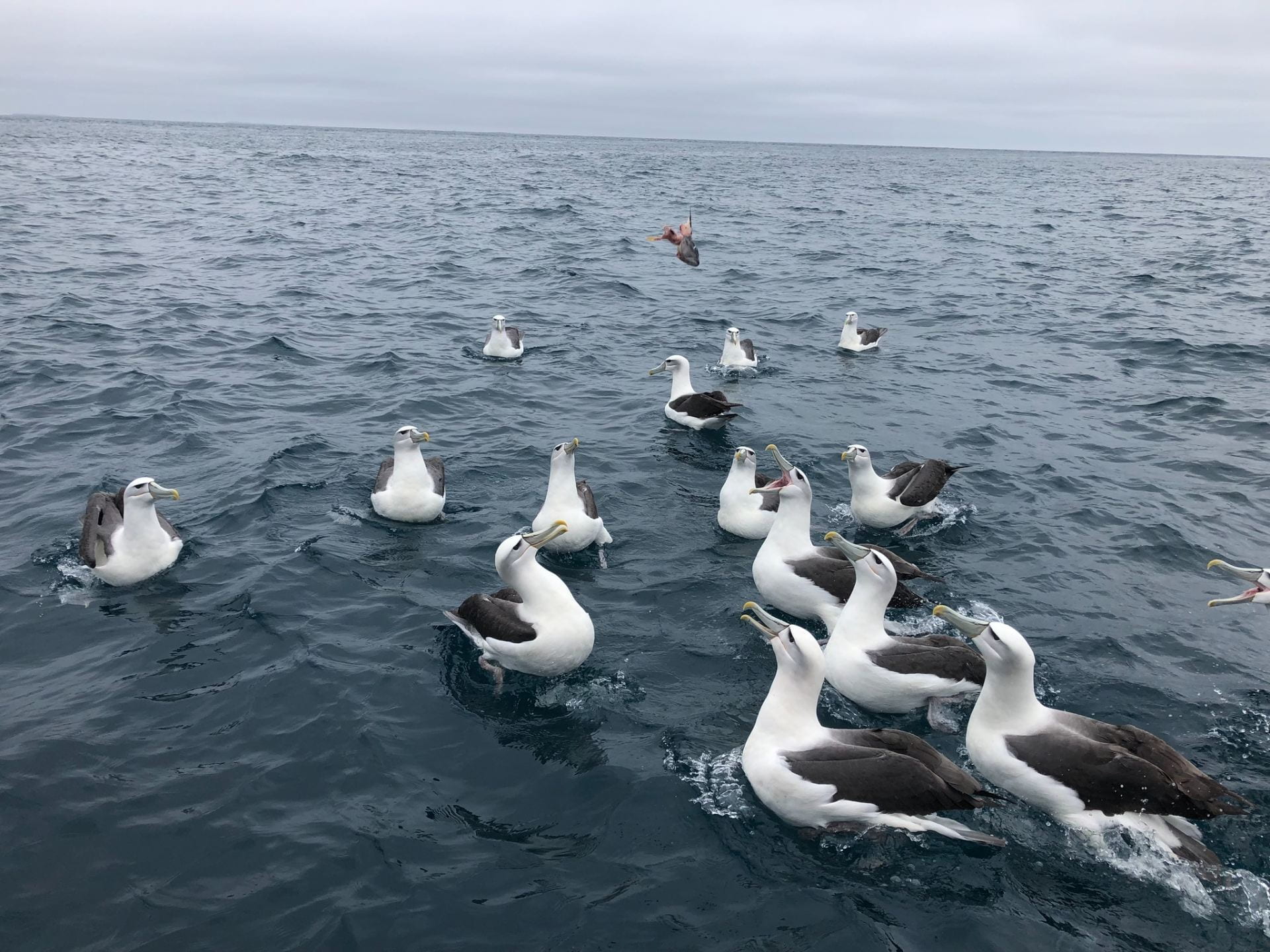
Understanding the interactions of fisheries with species and habitats is important. Image credit: white-capped mollymawks/toroa (Thalassarche steadi), Jody Allair/iNaturalist (CC BY-NC 4.0).
Clear species-specific spatial and seasonal variability in bycatch per unit effort has been identified for seabird bycatch in gillnet fisheries, highlighting high-risk situations occur that could be avoided, while full fishing activity is appropriate at other times.[166] Significant closures to set netting have been regulated in Aotearoa New Zealand because of this.
The timing and location of commercial fisheries operations plays a large role in dictating the impacts of fishing on marine ecosystems. Some of the issues relating to fishing sustainability – catching protected species as bycatch and damaging significant habitats – could be managed by changing where and when fishing occurs. This approach has already been applied in some fisheries where, for example, fishers set their longlines at night to avoid seabird bycatch. The FAO guidelines interpreting UNGA 61/105 on the protection of vulnerable benthic ecosystems include limited spatial extent of bottom fishing as a method to mitigate significant adverse impact.[167]
Some of the issues relating to fishing sustainability – catching protected species as bycatch and damaging significant habitats – could be managed by changing where and when fishing occurs. This approach has already been applied in some fisheries where, for example, fishers set their longlines at night to avoid seabird bycatch.
There is significant opportunity to use innovative tools and techniques to make these changes more specific, dynamic and precise.
First we need to more holistically study the oceans and biota within it. There are knowledge gaps that need to be filled about habitats, lifetime and seasonal movements of marine species, and fisheries interactions with protected species to better understand how to target some species and avoid others.[168] New tools such as drones, autonomous vehicles and satellites are making it possible to gather data from otherwise inaccessible areas. Advances in biochemical techniques can further grow the knowledge base to inform management, and EM can expand data collection efforts and validate observer data.
Next, we can use this information to predict patterns of movement and behaviours. Those predictions can feed into spatial decision support tools that model the risks of coming into contact with protected species. These are already relied on in fisheries management in Aotearoa New Zealand but improving frequency and pace of data collection and analytical processes would allow quicker decision making by fisheries managers. Advances in data science can strengthen the evidence base that feeds into decision making, such as the work by Dragonfly Data Science.[169] The predictions will need to also incorporate projections about how species might respond to changing climates and oceans, as well as evolutionary changes in response to various factors, including fishing.[170]
Ultimately, the goal is for this knowledge and new tools to come together to enable dynamic ocean management, with the goal of near-real-time decision making in the marine space. Being able to dynamically manage the ocean with more precise spatial and temporal management of commercial fishing could support fishing to continue at sustainable levels while minimising impact on non-target species or particular habitats.
In this section, we discuss how innovative tools and new scientific approaches can be used to study, predict and inform in real time where and when fishing should take place, and monitor illegal fishing.
These issues are pertinent to recommendations in Themes 4, 6 and 7.
New tools can refine spatial and temporal knowledge of marine life to inform fisheries management
In order to manage our marine environment better we need to build on our knowledge of ecosystems, habitats and species-specific movements and behaviours, to improve the temporal and spatial resolution. This will allow for fisheries management that is more flexible and adaptable to the changing conditions in the ocean, thus enabling fishing to take place with a far reduced risk of adverse impacts on species and habitats. Data can be collected at a finer scale and captured over time to better understand dynamics so that these can be taken into account for the dynamic management of fisheries. There are new tools that can help grow this knowledge base and generate data to inform predictive and real-time management of protected species and habitats:
- Drones and unmanned aerial vehicles (UAVs) (see below).
- Genetic tools (eDNA and wildlife forensic tools) (see sections above on genetic technologies and eDNA).
- Satellites (see section below).
- EM (see section above).
- Acoustic transmitters (see above).
- Underwater backscatter localisation. A battery-free ‘underwater GPS’ tracking technology has been developed at a proof-of-concept level using piezoelectric materials.[171] The technology provides an alternative to acoustic location technologies that require batteries and can be bulky and limit the tracker’s lifespan – in contrast these systems could be low power, low cost, and scalable. The materials generate their own charge in response to receiving soundwaves and the receiver translates the backscatter into a low-bitrate binary code. However, the technology is in its infancy and requires further development to be able to be applied more widely in ocean exploration applications.
- Biochemical techniques. Chemical signatures derived from biochemical techniques (described in section above on biochemical technologies) can provide insight into species habitats, as shown in the case study ‘How a chemical fingerprint identified Aotearoa’s most significant snapper nursery’. The information from these studies can inform approaches to protect certain areas to sustain healthy fish stocks. As these analytical techniques become more refined and the cost declines over time they could be applied more widely.
Drones and autonomous vehicles ease access to difficult-to-reach parts of the ocean
Technological innovation is opening up new opportunities to map, assess and monitor habitats using UAVs, such as drones. Marine habitats are vast and can be difficult to access. UAVs can allow access to areas of interest from the air, sea surface or underwater. This allows researchers to explore new frontiers in the marine environment because of their ability to access otherwise hard to reach places. Technological advancements and decreasing costs are making unmanned vehicles increasingly accessible and easy to use. These vehicles can combine cameras and/or sensors to record a multitude of data.
UAVs can be used for mapping, assessing and monitoring habitats. After the 2016 Kaikōura earthquake, scientists deployed drones to survey the rocky reef and intertidal habitats affected by uplift.[172] The drones were equipped with visible and infrared cameras to investigate the recovery of kelp and other macroalgae, and the extent of juvenile pāua loss. In Western Australia, an autonomous underwater vehicle has been used to survey benthic habitats with the aim of contributing to ecosystem-based fisheries management.[173]

Multi-spectral drone image of uplifted kelp along the Kaikōura coastline. Image credit: Leigh Tait.
After the 2016 Kaikōura earthquake, scientists deployed drones to survey the rocky reef and intertidal habitats affected by uplift. The drones were equipped with visible and infrared cameras to investigate the recovery of kelp and other macroalgae, and the extent of juvenile pāua loss.
UAVs can also be used to track, count and measure marine mammals (see case study: Māui Drone Project). Unmanned aerial systems have been used to survey Antarctic fur seals[174] and leopard seals[175] in Antarctica, and to count and measure gray and harbour seals[176] in the UK.[177, 178] They have also been deployed to measure and photograph southern right whales/tohorā.[179, 180]
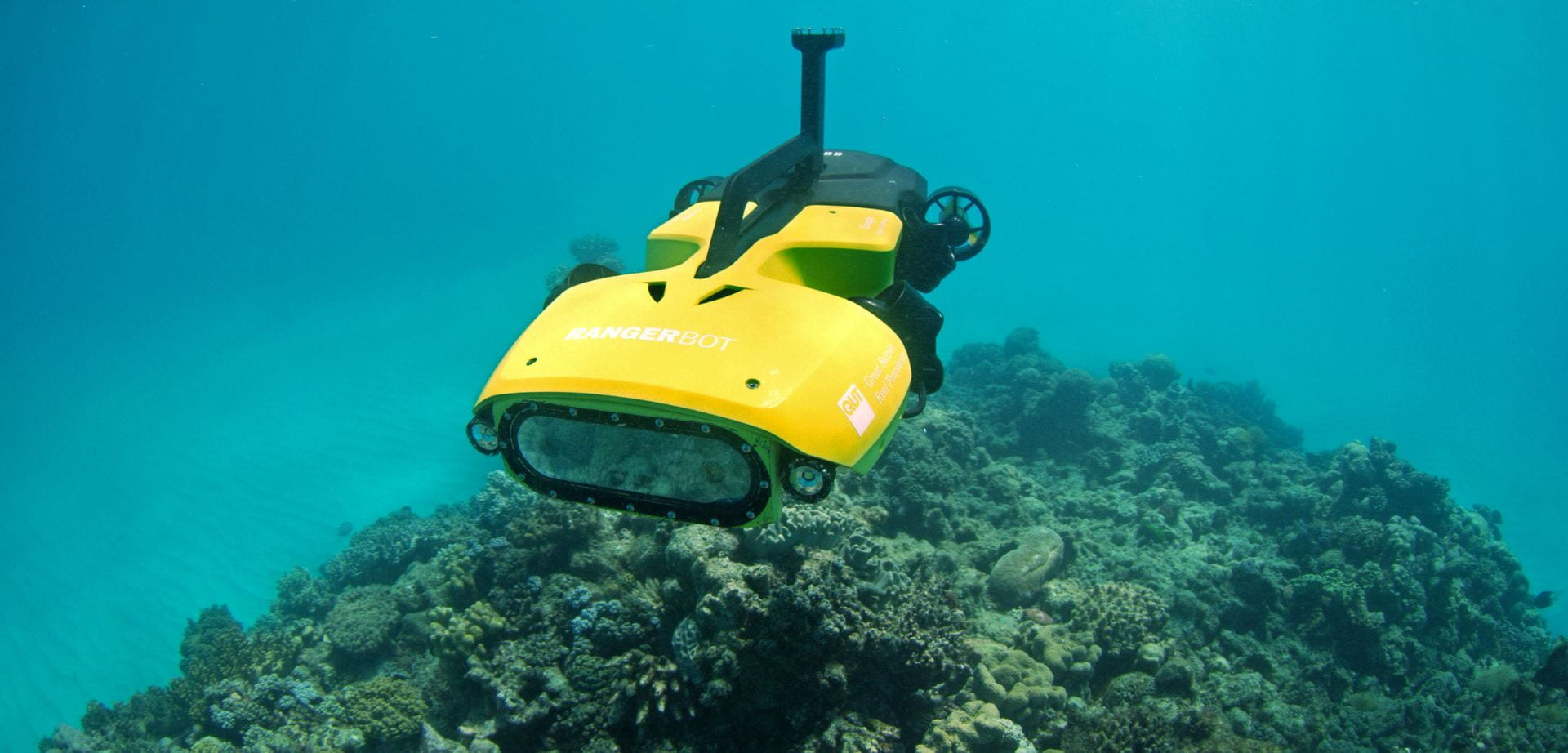
The autonomous RangerBot that can identify invasive crown-of-thorns starfish on the Great Barrier Reef. Image credit: Queensland University of Technology.
There are regulatory and safety hurdles relating to the use of autonomous vehicles. New Zealand’s Civil Aviation Authority regulates the use of Aotearoa New Zealand’s airspace. The Civil Aviation Authority administers specific aviation rules including aircraft weight restrictions and flying height restrictions. They also specify that aircraft must be within visual line of sight – that is, you must be able to see the aircraft at all times with the naked eye. This distance can range from 500 m to about 1.4 km.[181] When operators will not be able to meet the requirements of these rules, they can gain an exemption to operate their craft under different rules.[182] This requires a risk assessment that shows how risks will be mitigated. Two reports have identified potential economic benefits from developing the capability for safe beyond line of sight use of drones – particularly in farming, forestry, transport, construction and utilities contexts.[181, 183] At the time of the report, there were no automated systems that could meet the safety equivalent of manned aircraft so beyond line of sight drone use could not be achieved.
Autonomous vehicles can also be used to track invasive species. Coral-devouring crown-of-thorns starfish[184] are a threat to the Great Barrier Reef. Researchers have designed an unmanned robotic system known as ‘RangerBot’ to automatically identify crown-of-thorns starfish, and administer a lethal injection of bile salts.[185]
Satellite technology allows us to track marine species in detail
Using satellite sensors allows wide-ranging observations of movements around the ocean over various spatial and temporal scales.[186] Satellite tagging and tracking in the ocean has emerged through technological advances in batteries, hardware and software, which have facilitated the development of smaller devices, enabling organisms across a vast range of habitats in the ocean to be monitored.[187]
Improving and emerging satellite technologies have promising applications in fisheries management. Species can be tagged with satellite tags and their movements monitored. Pop-up satellite archival tags have been used for nearly 20 years to track species movements and behaviours.[188] Developments in satellite technology are enabling more refined characterisation of the horizontal and vertical movements of individuals, populations and entire communities over wide-ranging spatial and temporal scares – from metres to tens of thousands of kilometres and from hours to years, and in some cases the lifetime of individuals.[187] This can be coupled with oceanographic data to understand what drives movement and behaviour. Some examples of innovative applications of satellite technology are outlined below.
- Satellite technology has been integrated with other research tools to determine the spatial patterns of humpback whales[189] and southern right whales and other baleen whales.[190–193] Satellite tags have been used in conjunction with genomic and chemical analyses to follow the migration and feeding grounds of southern right whales around the subantarctic Maungahuka Auckland Islands.[193] Chemical analyses of stable isotopes in the skin provide information on foraging, along with DNA, RNA and epigenetic analyses which show the whale’s sex, age and identify individuals. The researchers also use drones to measure the whales’ size and fatness and use integrative statistics to combine insights from these different methods to estimate (a) how many whales there were before whaling and (b) how many whales there are now. The information garnered from these studies is useful for projecting future management of potential entanglement issues and proactive fisheries management based on this evidence.
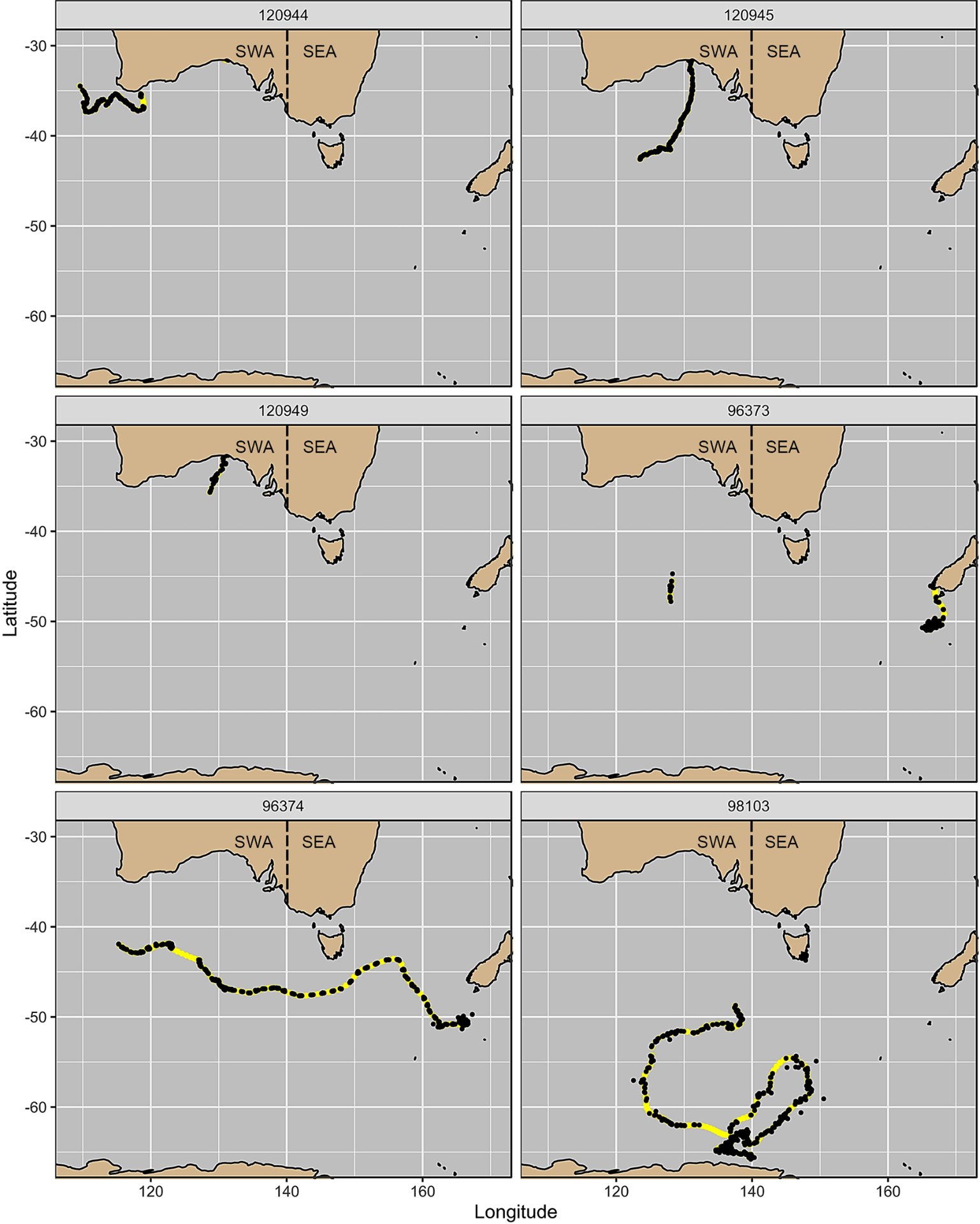
Satellite tracking routes of southern right whales in the Southern Ocean. Image credit: PLOS One.
- Data from satellite remote sensing technologies was integrated to understand environmental factors, habitat use and movement patterns of sharks and rays to support conservation and management.[194]
- Satellite tags were used to remotely sense and monitor cases of illegal fishing in a shark sanctuary in the Republic of the Marshall Islands.[195] Illegal fishing is discussed further below.
- Information gathered from satellite tracking has been used to identify areas suitable for a fishery closure.[196]
- A study using satellite tags on killer whales[197] in Norway found that the whales were attracted to herring[198] fishing vessels which suggests deterrent approaches may be the most effective fisheries management solution.[199]
Limitations and opportunities
- Satellite tagging is labour intensive and tags are expensive.[168]
- Currently there is limited spatial resolution of satellite remote sensors[194], but this is likely to be able to be improved over time.
- Similarly, there can be temporal limitations depending on how the study or system is designed. Short studies may miss capture events or dynamics and things like cloud cover can interrupt data collection.
- Satellite tagging requires species to be caught once to deploy the tags but doesn’t require recapture, which is an advantage over mark recapture method.
- Developments with batteries and hardware will continue to reduce the size of tags.
Electronic monitoring can be used to collect data on interactions between fishing and protected species
The oceans around Aotearoa New Zealand are biodiverse. With this rich natural heritage, EM trials in Aotearoa New Zealand have often explicitly aimed to assess interactions with threatened, endangered or protected species – a focus not as central in EM trials elsewhere.
Selected EM trials in New Zealand that detected threatened, endangered or protected species interactions.
Fishing method(s) | Protected species detected using EM | Reference |
---|---|---|
Demersal longline Pelagic longline | Seabirds, turtles, fish including sharks and rays | [200] |
Trawl | Seabirds, cetaceans, sharks and rays | [201] |
Set net/gillnet | Seabirds, cetaceans, fish including sharks and rays | [202] |
Set net/gillnet | Seabirds, cetaceans | [203] |
In Australia, EM for threatened, endangered or protected species has progressed beyond the trial stage and is part of at least two full-scale, operational programmes: seabird captures are monitored in pelagic longline fisheries, and pinniped and cetacean captures are monitored in gillnets.[68]
A 2018 review of EM for monitoring interactions with threatened, endangered or protected species found that EM is effective for detecting a range of events across different fishing methods, including deployment of mitigation devices, bycatch events and unusual behaviour. It is possible to identify species from EM imagery, even with the challenging conditions that can include wet or obscured specimens.[74]
EM can change the behaviour of fishers. For example, fishers in an electronic-monitoring trial increased their reporting of seabird captures (see case study: Using cameras to protect threatened seabirds).[204] Digital monitoring is expected to greatly improve the information on seabird capture events across a broad range of fisheries.[205]
The 2018 review made recommendations for next steps to improve threatened, endangered or protected species monitoring via EM in Aotearoa New Zealand.[74] Most of these centred on the review component of EM: developing training materials and programmes, as well as developing quality assurance and data standards. Key among these was the creation of bespoke ‘fishionaries’ that catalogue and store photos taken by fisheries observers to use in EM training.
Comprehensive models can inform predictions about populations and protected species
Fisheries threats to marine mammals can be reduced through effective spatial management. This requires knowledge about where the animals are and how they are interacting with fishing operations. Data and knowledge generated from the tools discussed above can be integrated into models and risk assessments that can predict where bycatch events may occur and use that to inform fisheries management. Our ability to predict organism and community responses to changes in our oceans will be dependent on knowledge of animal movements, interactions, and how the physiological and environmental processes underlying them shape species distributions,[187] which is why filling the knowledge gaps is an important first step.
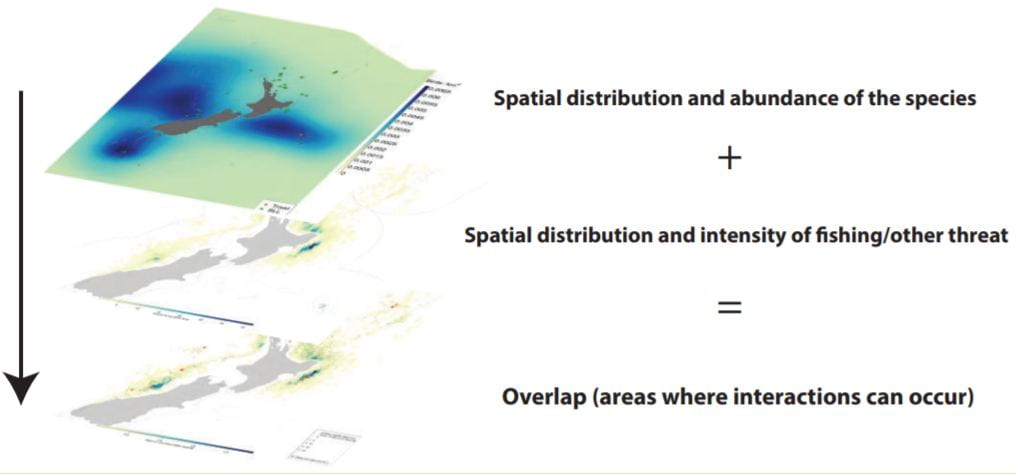
Schematic of spatially explicit fisheries risk assessment (SEFRA).
There are a range of tools that model data to estimate the probability of bycatch, to inform conservation measures and fisheries management:
- Bycatch prediction tools. A tool that combines species distribution models with oceanographic data to predict bycatch of pilot whales[206] in a longline fishery was strongly and significantly correlated with observed rates of bycatch in space and time, demonstrating that such tools could be accurately predict times and places with a high risk of bycatch.[207] Models to study bycatch of seals identified water turbidity was a major driver of seasonal trends in bycatch which could inform bycatch mitigation efforts.[208]
- Spatial decision support tools weigh up different spatial management scenarios and optimise spatial plans for maintaining ecosystem health and biodiversity. There are frameworks that can be used to assess the risk of encountering protected species during fishing to determine whether fishing can take place in a region. These tools are likely to become more refined over time as further data from the methods described above feed into them. Examples include:
- The SEFRA is an assessment that has been developed to estimate the risk to protected species posed by fishing activities.[209] The assessment can be used when there is little data available on mortality (for instance, where the species is rare, or fisheries observer coverage is very low). The assessment uses the spatial distribution and abundance of a species and combines it with the distribution and intensity of fisheries activities (or other threats) to estimate their overlap. It has been used to inform fisheries closures to protect Māui and Hector’s dolphins.[210]
- The Risk Atlas tool enables querying of fisheries risk to protected species and what the risk reduction benefits and costs are of different spatial management configurations.
- Freely available decision-support tool software such as Marxan[211] and Zonation[212] are also used for conservation planning. These tools help to define a system of protected areas using ecological, social and economic criteria. Both of these tools have been used in Aotearoa New Zealand to support marine management.
Other tools can be used to understand population dynamics.
- Models of spatial population dynamics and species distribution/habitat suitability. Models have been used for spatial distribution of cetaceans in Aotearoa New Zealand waters[213] and habitat suitability for corals and vulnerable marine ecosystems.[214] Integrating tagging and fisheries data into a spatial population dynamics model can improve its predictive skills.[215] These models have the same caveats outlined in the section above on models.
- Spatial Population Model (SPM) is a modification of the CASAL software (see section above), which models fish and fisheries distribution at a higher level of spatial resolution. SPM simplifies spatial distribution of fish over time and space using ‘preference layers’ to determine the probability that fish occur in each area at each time, and can be, for example, sea surface temperature, depth, or the distance from one cell to another. SPM is valuable because this simplified and unique way of modelling spatial distributions and movements allows it to operate as an estimation model. Therefore, rather than being just a tool for investigating the potential effect of space on fish stocks and fisheries (which most spatially resolved models are), it can estimate model parameters, including those controlling spatial movement, and be directly used in stock assessment. SPM has been used as part of the stock assessment of Antarctic toothfish,[216] and more recently for investigating potential distributional changes in tuna associated with climate change.
Ideally these tools will be adapted to inform management in real time, as discussed below in the section ‘Dynamic ocean management will help protect non-target species in real time’. Their use will grow once we have more data to refine the models.

Spatial distribution of biomass (in thousands of tonnes) for immature, mature, and spawning Antarctic toothfish as estimated by SPM. From [217].
Dynamic ocean management will help protect non-target species in real time
The ocean is not a static environment so effective fisheries management needs to be fluid in space and time to respond to the changing locations of marine species and its users. A number of tools can help to monitor the patterns of movement of protected species to first predict, but ideally inform in real time, the areas to avoid while fishing. Methods and tools previously described for other applications in fisheries can also be drawn on to inform dynamic ocean management to protect non-target and threatened species. The challenge lies in rapidly collecting and pulling relevant data together to inform decisions.
- Modelling. The Ecocast app (see case study: EcoCast – an app that can help fishers decide where to fish) highlights how a model can be applied to inform where fishers choose to fish to avoid bycatch in real time. FaCeT (Fisheries and Climate Toolkit) is another dynamic ocean management tool under development in the US that aims to provide real-time and forecasting information for fishers, bringing together fisheries and climate science.
- UAVs. Real-time communication from drones or autonomous vehicles to fishing vessels could enable responsive management. This is one aim of the Māui drone project (see case study: Māui Drone Project).
- Genetic technologies. eDNA tools can support protection of vulnerable, protected or taonga species by recognising where these species are and changing management practices accordingly (see case study: Managing great white shark conservation through eDNA).
- Acoustic technologies. Acoustic tags can be applied for real-time monitoring for direct conservation. Canada is using near-real-time acoustic technology, in addition to aircraft and vessel surveillance, to detect North Atlantic right whales.[218] Once a whale is detected, fishery closures are put in place to help protect them from entanglements.
- EM, including use of cameras. EM data can inform fishers of bycatch hotspots that should be avoided. In Alaska, pollock[219] fishers have banded together under the Pollock Conservation Cooperative, agreeing to stop ‘the race for fish’. Since opting for “a more rational, deliberate pace”, the pollock fishers have produced about 50% more products per pound of fish harvested. To reduce their bycatch of non-pollock species, the Pollock Conservation Cooperative have contracted private company Sea State. Cooperative members share their data with Sea State, who analyse this data and advise vessel operators of bycatch hotspots to avoid, in real time.
- AI. Automating video review could also be applied to detecting interactions with non-fish species, such as seabirds or marine mammals. In Aotearoa New Zealand, automated detection of seabird catches in video footage from longline fisheries has been trialled. By understanding how often bycatch occurs, we can better estimate the effect on threatened seabird populations, which in turn informs their conservation. However, this approach had a ‘rare event’ issue: out of thousands of fishing events captured in footage, only tens were seabird captures. This lack of data made it tricky to train an algorithm, and the difficulties were further compounded by changing operating conditions over time e.g. frame rate and resolution improvements. Combining AI technology with UAVs such as drones is another approach to monitoring fisheries interactions with threatened species.
Innovative tools can also be used to detect illegal fishing
Nearly two-thirds of the world’s oceans lie outside of any country’s jurisdiction or control. It is difficult to observe activity across these vast regions using conventional methods. A number of tools described previously in this chapter can also be used to monitor fishing vessel movements to detect illegal, unreported and unregulated (IUU) fishing and poaching.
- UAVs. Drones have been used to monitor fishing activities by countries including Belize, Jamaica and Costa Rica, and NGOs such as Sea Shepherd.[220] Recently an unmanned vehicle completed circumnavigation of Antarctica, surviving extreme conditions, and highlighting the potential for such vehicles to be used for other purposes. A strength of this technology is that it doesn’t require expensive boat time or crews.
- Acoustics can also be used for detecting IUU, such as incursions of vessels within MPA areas. In New Caledonia, they have deployed triangulated acoustic devices that detect vessels within an MPA area that then cues further activity, such as deployment of a patrol boat to investigate the incursion.
- Satellite monitoring of boats. The application of satellite-related tracking systems is widespread in fishery management, exemplified by the massive and intensive use of vessel monitoring systems.[220] As part of the automatic ship identification system (AIS), some ships are fitted with a transponder to broadcast the ship’s identity, position and course.[221] The worldwide AIS for monitoring solutions on the high seas is ostensibly aiming to prevent ship collisions, but satellites have enabled the detection of AIS data en masse. Satellite monitoring of vessels has been used to monitor fishing activity, observing compliance with regard to MPAs in particular.[222] In another study, four satellite technologies, combining AIS data, optical imagery, infrared imagery, and satellite radar were integrated to create the most comprehensive picture of fishing activities in North Korean waters to date and identified illegal fishing activities from Chinese fishing boats.[223]
Screengrab of the Starboard interface. Image credit: Starboard.
- Data science, machine learning and AI.
- Aotearoa New Zealand company Xerra Earth Observation Institute (Xerra) have developed a cost-effective way to monitor fishing activities and detect potential IUU fishing. The platform, known as Starboard™ Maritime Intelligence combines datasets from multiple sources to derive insights about the vessels fishing in an area (see screengrab, above). The visual presence of a vessel (through synthetic aperture radar and optical imagery data) and emissions from a vessel’s marine navigation radar (through radio frequency data) are compared to AIS data to uncover which boats are reporting their location and those that aren’t. To help determine which species vessels are targeting, Starboard incorporates sea surface temperature data. The integrated data can also be used to detect unusual and noteworthy vessel behaviour. A proof-of-concept pilot operation to detect IUU fishing of southern bluefin tuna in the Tasman Sea was successful at detecting a dark fleet of vessels. The cost of monitoring the area using satellites over a two week period was roughly equal to a typical eight-hour, maritime surveillance flight.
- Researchers used machine learning to verify whether FADs were used in purse seine fishing, which could have useful applications where such use is banned.[224]
- Non-profit organisation Global Fishing Watch uses AIS data to increase the transparency and sustainability of fishing on the high seas. Algorithms can separate fishing activity from non-fishing behaviours such as transiting from AIS data. In this way, Global Fishing Watch can track fishing activity and intensity across areas of the ocean that may be otherwise inaccessible. This approach revealed fishing activity before and after the establishment of an MPA near Kiribati, where all fishing activity ceased upon closure bar one vessel, which was fined by Kiribati. In 2016, Indonesia partnered with Global Fishing Watch, making data from their vessel monitoring system available publicly. This allows Global Fishing Watch to track Indonesian vessels over a certain size that do not use AIS. Other countries such as Peru, Costa Rica and Chile have subsequently followed suit. The Global Fishing Watch algorithms have also been used to identify when fishing vessels are setting longlines, with the aim of assessing how many vessels adopt best-practice night-setting to mitigate albatross bycatch.
- Innovative New Zealand company, X-craft have developed an alternative approach to patrolling the high seas: the Proteus, an unmanned sea vessel powered by solar panels and a mini wind turbine. The Proteus is equipped with a range of sensors, an on-board drone, and an AI system that allows the vessel to make decisions – about whether to follow or track a vessel of interest, for example.
Starboard™ Maritime Intelligence combines datasets from multiple sources to derive insights about the vessels fishing in an area. The visual presence of a vessel (through synthetic aperture radar and optical imagery data) and emissions from a vessel’s marine navigation radar (through radio frequency data) are compared to AIS data to uncover which boats are reporting their location and those that aren’t.
How we ensure a healthy ocean
Sustainable fisheries depend on a healthy ocean. As discussed in the section ‘Fishing is one of many stressors on our oceans’, there are a range of stressors beyond fishing that impact the health of the ocean. An understanding of the wider ecosystem is critical to understand how these stressors will drive oceanographic changes, including changes in temperature, salinity, circulation, primary production and acidification.
Monitoring changes within the ocean is crucial to understand and respond to the downstream impacts on the commercial fishing sector. We can use new techniques and technological advances to build on and improve current datasets. Monitoring our ocean systems and ensuring a healthy ocean will in turn promote healthy fish stocks and ecosystems.
New technology can make it easier to monitor the marine environment
Long-term monitoring of our oceans is very important, particularly with the inevitable changes caused by a changing climate. Several important indicators can be measured to track of the health of our oceans. Prior to a range of technological developments, ocean measurements had to be made by research vessels which was painstaking and expensive. The result was that the global ocean was poorly sampled.
The southern hemisphere ocean region was particularly poorly measured because of the large sizes and remoteness of the South Pacific, Indian and Southern Oceans, together with the relative lack of local wealthy nations funding oceanography.
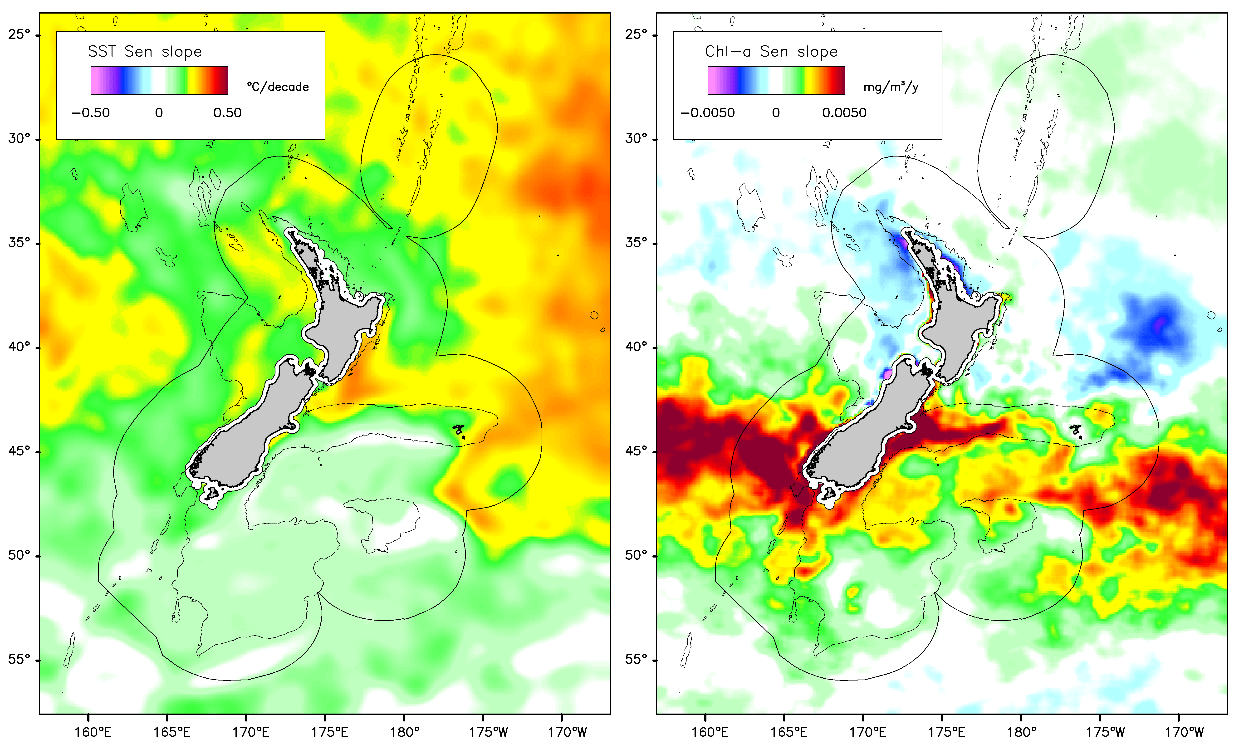
Long term trends in (a) sea-surface temperature (1981-2018) and (b) phytoplankton abundance (1997-2018).[225]
Technological developments have opened up new opportunities to monitor our vast oceans. We can draw on these developments to build on Aotearoa New Zealand’s existing monitoring efforts. In the boxes below, we highlight the data types of importance for monitoring the marine environment, any current initiatives that contribute to monitoring in Aotearoa New Zealand and opportunities to improve monitoring. Data collected from this range of tools could be coordinated and collated into an ocean observing system, as discussed in the following section. Monitoring of all ocean measures could be improved through a nationwide series of inshore surveys that collect a range of environmental and fisheries data and there are also opportunities to utilise existing efforts (e.g. tagged animals) to gather further information, which are not explored further here.
Temperature (on surface and at depth)
Significance
Changing temperature can lead to species movement and can also have indirect effects on fish recruitment, fish productivity, ecosystem services (biodiversity) and water quality (e.g. nutrient supply for plankton productivity and source of food for pelagic fish).
Higher temperatures may also result in stratification – with eutrophic conditions inshore and oligotrophic conditions offshore; harmful algal blooms and higher rates of disease; and species invasion and biosecurity risks.
Extreme events such as marine heatwaves may have greater impact than long-term trends.
Current Aotearoa New Zealand initiatives
- Satellite surface temperature (SST) observation is the longest time-series for Aotearoa New Zealand’s ocean monitoring; it started in around 1981 and will continue for the foreseeable future.
- Two stations measuring SST and five coastal SST stations.
- Argo (see case study).
- EXpendable Bathy Thermographs (XBTs).
- Temperature sensors deployed on boats through the Moana Project (see case study).
- Autonomous underwater vehicles (Slocum gliders) that carry sensors to measure temperature, salinity, light, oxygen, turbidity and fluorescence below the surface of the ocean.
- Part of GOOS (see section below).
Opportunities to improve monitoring
- UAVs can collect data on weather conditions and surface temperature.[220]
- Reinstate buoyed moorings.
Ocean acidification (changes in saturation horizon, aragonite, calcite)
Significance
Ocean acidification can cause harm to marine ecosystems, having direct and indirect impacts like changes to the behaviours and physiology of creatures.
Ocean acidification can: cause dissolution of organisms with calcareous shells or exoskeletons (e.g. plankton, shellfish –particularly juveniles, crustaceans, algae, deep-sea corals); and change the behaviour responses of invertebrate and fish species.
Shallowing of saturation heights and dissolution of deep-sea coral fisheries habitats.
Data on ocean acidification is important for improving our climate modelling (see section ‘Climate change is a huge threat to our oceans’).
Current Aotearoa New Zealand initiatives
- NZ has the longest running ocean acidification records in the southern hemisphere taken off Otago, along a transect that samples surface waters of the coast, the subtropical front and subantarctic water.
- Limited monitoring sites with long-term data.[226]
- Other coastal monitoring, but not long enough to determine longer-term trends.
- New Zealand Ocean Acidification Observing Network established (see appendix 15).
- 14 sites now measuring ocean acidification.[227]
- A Munida transect (established in 1998).
- Argo (see case study).
Circulation
Current Aotearoa New Zealand initiatives
- Sensors deployed on boats for the Moana Project (see case study) also monitor circulation.
- Sea surface height, sea surface temperature, (and other satellite data), drifter studies, current metres, acoustic Doppler current profilers all provide circulation data.
Opportunities to improve monitoring
Satellites can be used to monitor circulation in the upper ocean.
Primary production
Significance
Plankton are a critical component of ecosystems with high importance to food web functioning.[228] The production rates of plankton underpin the productivity of the ocean including providing the energy or food source for fish. They also ultimately determine the biological carrying capacity of the ocean in a given area. Primary production rates are a function of many combined factors such as circulation, wind, upwelling, bathymetry, topography, latitude, geographic location, oxygen, nutrient and iron availability, and temperatures.
Characterising plankton communities in space and time and monitoring for change is challenging because of the ecological complexity and spatial heterogeneity of communities, short intergenerational periods and the current analytical inability to identify genus or species out of the lab, though recent developments are promising.[229, 230] Satellite measurements of phytoplankton abundance is usually species specific.
Monitoring changes in magnitude and patterns in Aotearoa New Zealand’s EEZ is likely to provide valuable context for understanding change in fisheries, changes to Aotearoa New Zealand’s marine biogeochemistry and climate-related changes to ocean productivity.
Current Aotearoa New Zealand initiatives
- The current time-series of satellite measurements of phytoplankton abundance started in 1997 and is ongoing (see figure above).
- Continuous Plankton Recorder (CPR) transects have been run regularly between Lyttelton and the Ross Sea since 2008, but only occasional CPR transects have been carried out elsewhere in the Aotearoa New Zealand EEZ. The CPR can provide wide-area, long-term and cost-effective information on zooplankton communities. This plankton-sampling instrument is towed from the back of ships and samples water at about 10 metre depth (which represents plankton assembly at other depths).[231] Water is filtered through silk, then the silk and plankton are preserved for later counting and identification of species in different regions in the lab. Collection takes place at normal cruising speeds so the recorders can deployed from any vessel and opportunistic collection is possible.
- CPR data can then be related to environmental data such as water temperature, ocean mixing and primary productivity,[228] enabling trend-analysis to investigate patterns of long-term change.
Opportunities to improve monitoring
- A project with the Antarctic Science Platform aims to develop a high-throughput genetic and optical (size-based) analysis method for CPR data to reduce the cost and time of laboratory analysis and improve data quality.
- Establishing a regular CPR transect through across the Chatham Rise would be extremely valuable for characterising zooplankton communities in this key area and understanding variability and change in this crucial ecosystem component in a major fisheries area.
- It would be useful to expand regular CPR data collection from other parts of Aotearoa New Zealand, not just the Chatham Rise. E.g. going north from Lyttelton, and also from Wellington to Sydney.
- It is a cost effective way of ground truthing remote collected satellite data on primary productivity.
Invasive species
Significance
Algal blooms, toxic algal blooms, disease, nuisance species can all have a detrimental impact on marine ecosystems.
Current Aotearoa New Zealand initiatives
Port surveys, satellite data as existing indicators; monitoring of harmful algal blooms by Cawthron.
Opportunities to improve monitoring
- eDNA tools are able to detect invasive species and early biofouling communities and screen for pest taxa and support biosecurity management[232, 233] and targeted detection of high-profile marine pests around shipping hubs. It can be coupled with eRNA to reduce false positives.
- Acoustic techniques have the potential to detect invasive species. The research relating to this is in its infancy, but acoustic technology may be able to be applied for early detection of invasive species for biosecurity purposes.[234]
Pollution
Significance
Marine litter and plastic pollution in the ocean have been identified as a burgeoning problem worldwide and Aotearoa New Zealand is not exempt.
Current Aotearoa New Zealand initiatives
- Ocean Plastics Simulator
- Sustainable Coastlines Litter Intelligence
- FNZ project to identify density of seabed litter from DTIS camera surveys
- CPR series analysed for microplastics in surface waters between Lyttelton and the Ross Sea.
- DOC monitors sightings of plastics in seabird nests, seabird stomachs.
Opportunities to improve monitoring
An ocean observing system can address the challenge of managing multiple stressors
The data and tools described in the previous section can form the basis of an ocean observing system (OOS). An ocean observing system collects and coordinates ocean-related observation data like temperature and salinity. It is an ongoing and collaborative system. At a high level, ocean observing systems collect data on climate, provide data for services like weather forecasting, and monitor marine ecosystem health.
On a global scale, the United Nations established a Global Ocean Observing System (GOOS) in 1991, which Aotearoa New Zealand participates in through the Pacific Islands Ocean Observing System. The system is co-sponsored by the Intergovernmental Oceanographic Commission of UNESCO, the World Meteorological Organization, the United Nations Environment Programme, and the International Science Council. There is also a framework for ocean observing (last updated in 2017) and most recently they have published a 2030 strategy for the GOOS.[236] There are many other systems feeding into GOOS, including OceanSITES (sustained interdisciplinary time series environment) observation system, which measures variables from sea-air interactions down to 5,000 m depth. NIWA is a member of OceanSITES.
At the global level, many of the benefits of an ocean observing system cannot be directly applied to fisheries management because data isn’t localised enough, though there are wider benefits – for example, improving early warnings of severe weather events like floods, droughts and storms.
Fisheries management and ecosystem services are stated to have been significant drivers for the requirements of GOOS over the last decade.[237] However, commentary detailing the trend towards EBM and the need for GOOS to service this need goes back 20 years in the literature.[238, 239] The OceanObs’19 conference canvassed the aspirations of what an ocean observing system can provide – including addressing the needs of fisheries and EBM practitioners.[240] At the global level, many of the benefits of an ocean observing system cannot be directly applied to fisheries management because data isn’t localised enough, though there are wider benefits – for example, improving early warnings of severe weather events like floods, droughts and storms.[236]
There are many other forms of OOS – regional, coastal, and national systems – which collect detail at a finer scale. Some of this data feeds into GOOS while other data is collected for other purposes. Australia has the Integrated Marine Observing System and the US has the Integrated Ocean Observing System.
Aotearoa New Zealand does not currently have an OOS but would benefit from one as it would assist prediction, mitigation and management of the effects of multiple stressors, including climate change, sea-level rise, ocean acidification and impacts from changing terrestrial fluxes.[241] Current ocean monitoring efforts in Aotearoa New Zealand could be built on to establish this.[241] The local research community has work underway to plan for this. In 2018, there was a planning workshop for a New Zealand OOS involving research institutes, government, and businesses. Outcomes from the workshop are presented in a paper by O’Callaghan et al.[241] The paper describes how an integrated OOS for Aotearoa New Zealand can be developed in Aotearoa New Zealand that integrates mātauranga Māori with western science. The NZ-OOS would be “bottom-up community-driven” and success requires inclusiveness and cross-institutional engagement. The biggest hurdle would likely be sufficient and sustained prioritisation of the system, with government data streams being the backbone of the system. Details of the plan and overarching structure are included in the figure below and appendix 16.
An OOS adds a more sophisticated dimension (in space and time) to enable a 3D view which is dynamic instead of spatial tools such as MPAs.
An OOS adds a more sophisticated dimension (in space and time) to enable a 3D view which is dynamic instead of spatial tools such as MPAs.
Framework for the NZ-OOS building on existing efforts in Aotearoa New Zealand, from [241].
Using the whole fish to develop high-value by-products
Because there is limited scope for harvesting more fish, adding value to the existing harvest is an attractive path to increasing revenue for the commercial fishing industry. One way to achieve this is through developing high-value by-products.
Over the past 30 years there has been significant research and development activity in Aotearoa New Zealand directed at development of high-value marine products. Despite successful research and product/process development, progress to market has been slow and much raw material is still sent to low-value fish meal and oil. Recently the situation has started to change as the larger fishing companies (particularly Talley’s and Sanford) seek to generate more value from the same or decreasing catch volumes. The industry recognises that significant potential remains, but full realisation needs a change in approach. A path to transformation of the Aotearoa New Zealand industry and making full utilisation and maximised value the norm are to be investigated within the 2020 Cyber-Marine programme funded by the Ministry of Business, Innovation and Employment, a collaboration between industry, research organisations (Aotearoa New Zealand, Norway) and universities (Aotearoa New Zealand, Australia). It takes a very similar approach to that discussed earlier, with a focus on using real-time data, AI, automation and new technology to provide flexibility and rapid response to the diverse raw materials that are characteristic of the Aotearoa New Zealand fishing industry.
Iceland arguably lead the world in their use of fish by-products and we can look to them as an exemplar for how to unlock this potential in a national commercial fisheries industry. Most fisheries nations use around 50% of the fish but Iceland’s fisheries use over 80%, with many of the applications being high value. Leading fisheries companies in the country have publicly stated that their goal is to use 100% of the fish.[242]
The seafood industry is one of the pillars of the Icelandic economy. It is a dynamic ‘base industry’ which now forms the foundation for a diverse range of other industries.[242] Over the past few decades, the volume of catch in Iceland has decreased but this has not translated to a loss in revenue across the industry. Instead, export value has increased thanks to efforts to maximise use of fisheries resources by using more of the fish. Taking cod as an example, between 1981 and 2011 landings decreased from 460,000 tonnes to 180,000 tonnes, but the total export value of cod products increased from US$340 million to $680 million.[242] This increase in revenue despite a reduction in landings is due to progression from only selling fillets at around $12 per fish to using by-products from the whole fish. There is an ambitious estimate that full utilisation of the fish into high value products could attract up to $3,500 per fish. A proprietary scientific process to develop wound care products from cod skin is responsible for the significant increase, increasing the value to over $2,500 per skin.[242] Taking a more conservative estimate would still see a significantly increased value per fish than what is generated without high-value by-products. In the Aotearoa New Zealand context, even production of relatively simple products as part of a 100% utilisation cascade could make a significant improvement to value recovery. Using predicted values from the Cyber-Marine research programme, the value of jack mackerel, currently sold whole into very low-value markets, could easily move from <$1.95/kg to $8.50/kg (figure below).
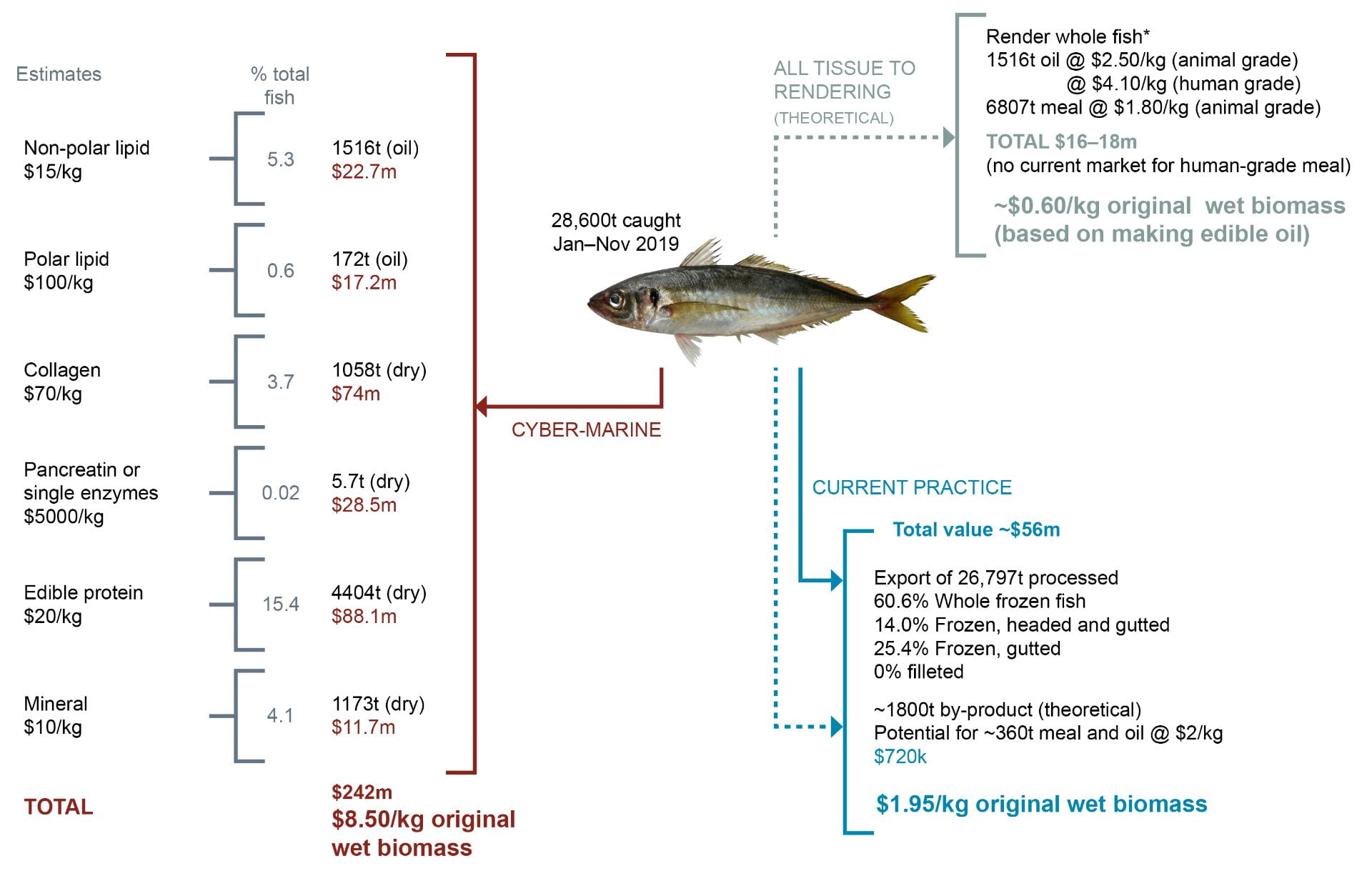
Potential to increase the value of jack mackerel through the refined use of by-products to be of higher value. Image credit: Plant & Food Research.
Iceland’s roughly 3000% increase in by-product use has generated around US$500 million per year and around 700 direct jobs – many of which are in rural coastal towns. For a population of around 360,000 people, there are more than sixty companies in Iceland working with by-products and processing of seafood.[242] Further growth of the industry will mean more jobs and even greater economic gains.
This success is in part due to the Iceland Ocean Cluster organisation and their 100% Fish Project, whose mission is “to inspire the seafood industry and seafood communities to utilise more of each fish, increase the value of each fish landed, support new business opportunities, increase employment and decrease waste.”
Strengths of this approach that could be drawn on in Aotearoa New Zealand’s efforts to use more of the fish include:
- Taking a bottom-up approach to accelerate innovation. The private-sector initiative operates an accelerator to support start-ups in the seafood industry. There are over 70 companies in the programme and a large group of entrepreneurs starting companies to capitalise on fish waste. The Iceland Ocean Cluster invests in these companies and also brings other investors in.
- Welcoming ideas from within and outside the fisheries industry. Though the network is mostly focused on start-ups, the cluster also supports innovation from incumbent companies in the fishing industry. This is important because although many of the innovative products have come from newcomers to the industry, it is difficult to realise some of the ideas without existing fisheries expertise and industry connections.[243]
- Incubating good ideas and offering a physical meeting space. Bringing people together to support their initiatives provides networking and learning opportunities, knowledge spill over and economies of scale to reduce the risk of failure.[243] The benefit of sharing space is shown by 70% of the companies who are part of the cluster having collaborated together. It is especially helpful to connect the entrepreneurs new to the industry with the experienced fisheries companies to share insights and overcome hurdles.
- Focusing on local value add in fishing communities. To restore opportunities to smaller fishing towns, some of the new innovations are in technology development for fish utilisation that would allow processors to operate on smaller scales, e.g. through modular systems, which could help to revive more remote fishing communities.
- Expanding networks beyond borders. The team developed an Ocean Cluster Network, which includes several clusters in the US, Canada and Norway, to strengthen innovation and utilisation of fish by-products so that products can be developed through collaboration within the network. There is plenty of opportunity to share lessons and collaborate, all while preserving the unique value propositions and commercially sensitive information inherent to each company, and several such projects are already underway.
Although some companies in Aotearoa New Zealand are taking steps in this direction, the wider seafood industry would benefit from transforming thinking around by-products, moving away from seeing by-products as waste to seeing them as a rich resource from which we can draw value. We could learn from programmes such as the Bioresource Processing Alliance. This could make the seafood industry become even more productive to the broader economy and aligns with the Government’s vision in its economic plan of moving from volume to value.[244] Using the whole fish requires product innovation in the versatile use of the fish by-products and process innovation in harvesting raw materials. Technological developments related to by-products from wild fisheries are relevant to aquaculture and vice versa.
Recognising that using more of our catch is not only positive for fisheries sustainability but also has significant economic potential, Aotearoa New Zealand should take a focused approach to accelerating opportunities in this sector. A local, context-specific approach will be necessary, but we can look to Iceland for inspiration and experience. The Iceland Ocean Cluster is part of a broader suite of related initiatives that contribute to a thriving by-products industry in the country. These include an annual international one-day conference, Fish Waste For Profit, aimed at companies involved in the commercial fishing, aquaculture and processing sector, and Ocean Excellence – a one-stop-shop consulting firm to help fishing companies develop solutions to use 100% of the fish.
Moving up the value chain
The approach that leads to the highest value will depend on the species of fish and the established market. Efficacy and profitability need to be balanced. A few species are sold as whole fish as this generates the highest value. For other species, the highest value product may be the primary product (e.g. fillets or collagen) with the rest of the fish processed into secondary products. Currently, there is general under-valorisation more than under-utilisation. Ultimately, the goal is for companies to develop a cascade of products for each species that can generate more value than they otherwise get by selling the fish whole, or generating low-value by-products.
Demonstration of the relationship between value add and quantity available for different categories of fish by-products. Click to enlarge.
Pharmaceutical, nutraceutical and cosmetic applications of fish by-products
Pharmaceutical uses of fish by-products include biotechnology, nutraceuticals and cosmetics. Several projects funded by Seafood Innovations Ltd relate to development of such products. There has also been considerable investment by the Ministry of Business, Innovation and Employment and its predecessors in projects through the Bioresource Processing Alliance and in projects funded by industry. These types of products require a high level of sorting and processing to pharmaceutical quality standards, but this leads to a high level of value-add.[245]
There are numerous medical and surgical applications from fish by-products in development. For example, squalene from shark liver is used as an adjuvant in vaccine delivery. One example in development by is the use of a crystallin protein found in the fish eye lens in ophthalmic surgeries (e.g. as a glue rather than sutures) or treatments (e.g. to treat corneal disease). Researchers at Otago University have used the sugar chitosan from squid pens to develop a gel to prevent surgical adhesions.
The nutraceutical market is also ripe for applications from fish by-products and Aotearoa New Zealand companies are already selling and developing nutraceutical and cosmetic products. It is important that these are scientifically validated.
Nutritional supplements and value-added food products from fish by-products
Nutritional supplements and food products require a high level of sorting and quality control to meet food-grade quality standards. Some products require mass transformation while others need to be sorted into the individual product. The value-add can range from moderate to high, and there is a moderate market capacity for these products. The potential for use of fish by-products in food fortification is influenced by nutritional value, bioavailability of nutrients, texture and smell, religious restrictions and regulatory requirements, but there are still challenges regarding safety and interaction with other ingredients and some technical concerns remain.[246]
Using fish by-products in agriculture, animal feed and energy generation
For fish by-products that cannot be processed into higher-value products in the pharmaceutical and nutritional markets, there are lower value but larger market capacity options that include animal feed (agriculture and aquaculture), biofuels, bio-stimulants and fertilisers. The quality standards for these applications are less strict and products generated require less sorting.

Fish offcuts at Lee Fish are saved and used as fishmeal in a variety of products.
Examples of applications for fish by-products
A number of companies in Aotearoa New Zealand are already manufacturing high value products from fish. Products include squalene from shark livers; fish oil for health supplements; marine collagen from fish skin for cosmetics and heath supplements; and shark cartilage as a health food supplement. It is important that such product development is validated by scientific studies on efficacy and focused on secondary product streams in existing harvests.
Body part | Composition and products of potential | Examples of application |
---|---|---|
Skin | Collagen and gelatin |
|
Bone | Cartilage calcium Collagen and gelatin Minerals | Calcium/bone supplement e.g. United Fisheries’ Nutri Zing (NZ). |
Muscle/red fish meat | Protein isolates – bioactive compounds e.g. carotenoids | Supplements e.g. BioBalance Astaxanthin from algae (NZ). |
Liver | Omega-3 oils |
|
Viscera | Enzymes e.g. trypsin High oil content (salmon) | Spray to protect against common cold e.g. ColdZyme (Iceland). |
Crustacea shell | Chitosan |
|
Swim bladder/maw | Collagen |
|
Scales | Collagen and gelatin | Bioplastic (UK). |
Fins | Collagen and gelatin | |
Blood | Nutrients, small protein molecules and iron | |
Heads | High oil content (salmon) | Oils, capsules, supplements. |
Mixed (i.e. offal) |
|
|
Shark cartilage | Chondroitin sulfate | Powders for health supplements e.g. Waitaki Biosciences (NZ). |
Mussels |
|
Challenges and opportunities
There is huge potential for large generators of fish processing by-products to extract more value. However, there are numerous challenges to overcome in order to process the whole fish into marketable products, with even greater challenges for developing high-value products. Some barriers are logistical, while others technical or social.
“We are sitting on a goldmine, but we don’t know how to tackle it.”
A significant challenge for Aotearoa New Zealand’s industry is having over 100 commercial species with different potentially valuable components that cannot be processed in the same manner as each other due to variable tissue mixtures depending on the market for other uses (e.g. skins for collagen, or heads for rock lobster bait or food; fish that may not be filleted so there is no economic means to separate tissues; potential species and quality variability in response to water temperature changes). In addition, our current marine products processing infrastructure designed for manufacture of single products has no flexibility and often destroys one component when recovering another. Making our challenges into unique opportunities requires knowing exactly what is in any raw material in real time, then using this information to direct processing, choosing from a suite of integrated technologies to maximise raw material use and product value.
A culture change is also needed to shift thinking from volume to value, which has been a long-term strategy across primary industries and a long-stated government goal. Further analysis of this is outside the scope of this report but analysis to understand why we are not moving faster up the value chain could be beneficial in the context of commercial fisheries. Policy could encourage innovation and reduce these barriers so Aotearoa New Zealand’s industry as a whole can lead in this space, while ensuring that higher value by-products do not impact food security.
One industry representative articulated the opportunities and barriers clearly when they said, “We are sitting on a goldmine, but we don’t know how to tackle it.”
Key ways to streamline commercialisation of fish by-products include:
- Improving knowledge of demand and opportunities for supply. The industry’s understanding of the consumer could be improved via better access to market data to inform investment, entrepreneurship and innovation. Connecting this market knowledge with what we know about the species, location and volume of by-products generated by our fisheries companies could help to identify opportunities to extract high-value products for which there is consumer demand. At the same time, developing species-specific understanding of potential proteins of interest via chemical compositional analysis is important to develop novel products which can also be high value.
- Addressing issues in processing systems and supply chains. Streamlining processes for higher value products could help to overcome some of the cumbersome aspects of by-product processing or remove logistical inefficiencies that make it unprofitable. This may mean improving aspects related to storage on vessels, split processing operations, or gaps in supply and processing chains, though these are generally thought to be of a high standard in our industry. Vertical integration of fishing and processing in Iceland has helped streamlined processes there and the WaSeaBi project in the EU aims to address these issues.
- Planning and support to establish infrastructure. Most commercial fisheries companies are capital constrained and the cost of establishing infrastructure and manufacturing processes can hinder product development. Many by-product project proposals have stalled due to high capital costs. Collaboration between companies or processors to establish economies of scale would be one step to help address this problem. Alternatively, different companies each specialising in key products or different parts of the supply chain and offering contract manufacturing to the others could address this challenge.
- Improving access to technical expertise and applied science. Many of the higher value products such as those with pharmaceutical applications require technical expertise. This is an area where great potential may remain untapped unless people within the industry become better connected to researchers with technical expertise to fulfil this development (see case study: High quality marine collagen from Aotearoa New Zealand). Some applications may call for the business to lead the research themselves, which requires more flexibility in contracts and research funding models as well as a research industry that is able to provide applied research as a service.
- Making it easier to do clinical trials: Clinical regulatory requirements can be a challenge to meet, both financially and logistically, but developing a clinically proven ingredient means a higher and more guaranteed income from the product. Funded or subsidised clinical research would remove the barrier that many companies face in making a high-value medical product from a fish by-product.
- Supporting networks and connection. As discussed in the section ‘How we fish’, facilitating connections between fisheries stakeholders is important in innovating for a more sustainable future. The Iceland Ocean Cluster and Canada’s Ocean Assets stakeholder database are examples of ways to do this with a specific focus on using the whole fish. The Bioresource Processing Alliance could be drawn on to support this.
Some challenges for by-product processing that are unique to wild fisheries (as opposed to aquaculture) include having to manage supply issues where there are short fishing seasons with large landings, rather than a constant supply year-round.
The Food and Beverage Manufacturing Industry Transformation Plan, currently in early development, may help to address some of these issues.
Improving traceability to add a premium to products
Traceability allows food to be tracked through all stages of its production, including processing and distribution. It is primarily used to facilitate food safety and quality assurance and is a cost of doing business internationally, but traceability can also increase transparency and build confidence in the sustainability of the product. This adds a premium, which customers will pay for (see case study: How a commitment to transparency and traceability has generated a premium product).
Widespread use of traceability systems that demonstrate social and environmental sustainability of commercial catch could help to reinforce best practice in the industry and send a message to consumers about the sustainability of that fishery, adding value in a commercial market. Companies that have fished legally and sustainably can prove this via traceability systems and set a precedent for making this information open and accessible while shining a light on illegal fishing and fraudulent labelling of seafood products. However, findings that significant illegal trade is ongoing in the Atlantic bluefin tuna fishery despite mandated catch documentation suggest that current systems need to become more watertight.[247]
There are different ways of measuring and reporting seafood production, processing and distribution, and additional methods to verify claims and identify false information. Catch documentation schemes are used to record and certify details of fish capture and processing, with the documentation accompanying the product through the supply chain. Records, where kept, were traditionally paper-based but traceability software now supports electronic data transmission from point of harvest to point of sale (or part of supply chain) via interoperable software e.g. barcodes, RFID tags and QR codes. Analytical methods and instruments to support traceability can be used to verify or falsify claims in a traceability system (but alone do not provide traceability). Systems can also be set up to alert users when data anomalies suggest irregularities or if companies are known to have expired certifications or are blacklisted on IUU database. Technological advances are likely to change these processes (see case study: Blockchain supply chain traceability project). As technologies improve, such as in EM, these can be combined to facilitate traceability systems that allow retailers to present a complete and transparent origin story for each fish.[11]
Government requirements for traceability, private sector sustainability commitments, and a greater interest in supply chain transparency because of legal and social risks are some of the factors driving seafood traceability systems to expand beyond food safety and inventory management.[248] Traceability systems used for compliance purposes could be built on for traceability that supports sustainable fisheries management.[249] These may need to be adapted when the objective of the system is to share information with the consumer in order to add a market premium, to ensure the information provided meets consumers’ needs.[250] How these systems impact the fishery depend on their legal or voluntary framework. For example, restrictions from an individual country or region around what fish can be imported only control what enters the end market, not what comes out of the fishery (i.e. the fish could be sold elsewhere). In contrast, a multilateral catch documentation scheme, backed by international law via RFMO, controls what is fished and monitors it throughout the supply chain.
In Aotearoa New Zealand, it is already a legislated requirement for seafood producers to track information[251] and import markets have their own requirements that our seafood industry needs to meet. For example, fish and fishery products caught and processed outside the EU have to comply with the EU’s eCERT framework in order for their import to be authorised.
The first global standards were established by the Global Dialogue on Seafood Traceability in 2017 and these could be implemented by the Aotearoa New Zealand seafood industry. There are also calls for a global, secure, interoperable support system for seafood traceability, but that faces many challenges such as technological and financial constraints, language barriers and regulatory differences.[252] In the meantime, voluntary approaches, country specific approaches and government-to-government agreements are likely to be where progress is made.
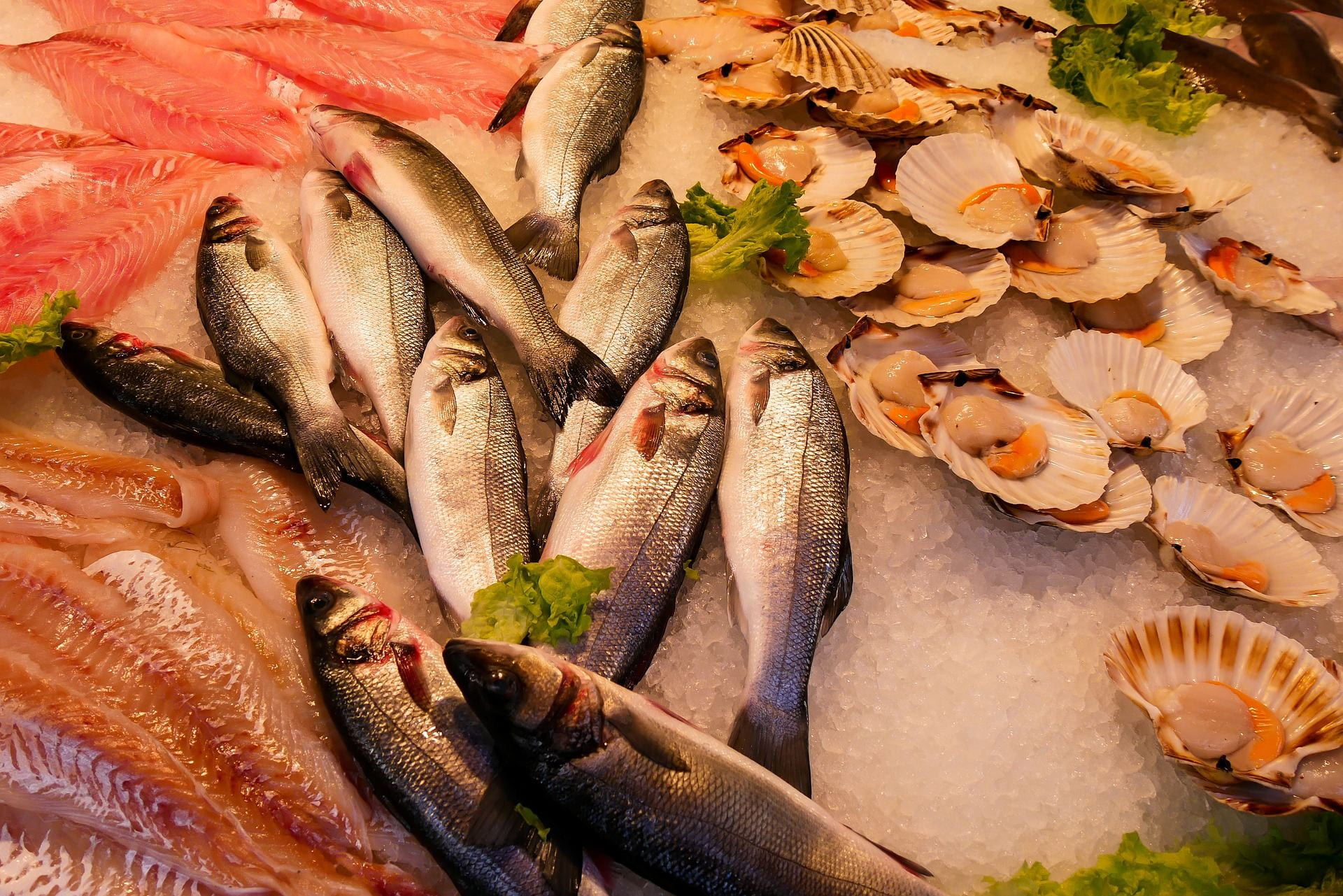
Accurate seafood labelling is important to protect threatened species and improve consumer trust.
Detailed labelling of seafood products has the potential to protect threatened marine species. A recent Australian study highlighted that over 90 endangered marine species can be legally caught in commercial fisheries across the world, many of which are importers into Australia. By the time the fish has been processed, exported and sold (with minimal details on the label), the consumer can be unaware of the plight of the catch.[253]
The researchers suggest four key elements that should be included to accurately label seafood:
- the species – bigeye tuna, not “tuna”,
- where it was caught – Queensland, not just “wild caught”,
- how it was caught – pole-and-line, not just “dolphin-safe”, and
- the company responsible for the fishing.
There also need to be mechanisms to monitor compliance and traceability of seafood. Using genetic sequencing to match a fish or fish product to a species, population or individual could support sustainable fishing by preventing food fraud and protecting vulnerable species.[254, 255] It could be used throughout the supply chain to stop practices such as product mislabelling. So far this is only used sporadically in fisheries (e.g. through FishTrace, FISH-BOL, FishPopTrace), despite suggestion that costs are lower than values of confiscated catches and fines.[255]
Implementing regulations and improving transparency across the global seafood supply chain will require national and international policy action. Awareness from seafood consumers, which has led to positive industry change in the past, will be critical to further improvements.[253]
Sustainability schemes provide a way to benchmark and improve fishing practices
One way to encourage improvements in fisheries sustainability is to set a benchmark for sustainable practices through accreditation or certification schemes, many of which come with an associated eco-label. With rising community expectations around the transparency and accountability of fisheries management, these types of schemes can help to assure people that products are sustainable. These rising expectations will also mean that certification needs to be credible with respect to protection, bycatch mitigation and gear impacts.
Some retailers may limit the products they will stock to only include those that have met sustainability standards. This means achieving certification gives that particular fishery market access. Where retailers don’t restrict the products they will stock, individuals may still choose to buy a particular product because it has an eco-label. The idea is that the positive economic impacts that come with being certified will encourage more fisheries to improve their practices to meet certification standards, which will in turn lift sustainability practices throughout the industry. Price premiums are reported in studies are around 10-15%, for example, 11% price premium in Baltic Sea cod fishery.[256] Additional benefits include greater social acceptance of the industry and improved governance processes.[257]
Private standards may be set internally by the individual organisation (first-party scheme), by an industry association for their members (second party), or by an independent organisation (third party). Over 30 third-party schemes already exist globally and many Aotearoa New Zealand fisheries companies engage with such programmes. Large numbers of schemes can sometimes create market confusion and can risk accusations of falsely representing a product to be more environmentally sound than it is. On the public side, some governments have supported the establishment of regional or national schemes, including France and Iceland, who have both based their eco-label on FAO guidelines.[258]
There are several important points regarding the application of sustainability schemes in fisheries management.
- Sustainability schemes are a way to set best-practice expectations. These schemes provide a way to make clear the expectations for sustainable fisheries management and support the industry to not only meet these but to continually improve their practices. Open and transparent certification processes and eco-labelling can maintain social license for fisheries, influence community perceptions of fisheries management, enhance public confidence in the sector and address concerns about the sustainability of fishing. Measuring the proportion of Aotearoa New Zealand’s fisheries that are accredited to a sustainability scheme could be a useful indicator of progress towards sustainability of our fisheries over time.[259] However, sustainability schemes may not be comprehensive in setting best practice – standards can be potentially set too low or may not address impacts of fishing methods such as trawling and dredging.
- Measuring sustainability requires a solid evidence base. Data and knowledge about fisheries stock status and wider ecosystem impacts are needed to assess whether a fishery is sustainable. The majority of fisheries will have enough data to inform the process but some, in particular small-scale fisheries, may be limited by this. Continued improvements in data collection and innovations to make fishing practices more sustainable will be key to making sure all fisheries can be assessed and pass certification (as discussed in the section above, ‘Data-driven knowledge is the cornerstone of effective and sustainable fisheries management’).
- The voluntary nature of schemes may limit engagement. Many local fisheries companies are already engaged with sustainability schemes, but there are barriers which prevent others getting involved while it is not considered essential for market access or is not government-mandated. For smaller-scale fisheries, financial or organisational barriers may be the reason for not engaging.
- Government assistance can make these schemes more accessible. To address the financial barrier that may prevent some fishers being assessed, the government can provide financial assistance to companies to get their products certified. In Aotearoa New Zealand, this already occurs for the MSC scheme via the Environmental Certification Fund.[240] The government can also fund baseline studies that benefit many fishers, for example in Western Australia the state government funded independent third-party assessment taking a bioregional approach to assess a number of different fisheries in a region at once.[260]
- Mandatory accreditation could even the playing field. As suggested by Telesetsky[64], a government scheme that indicates basic compliance with sustainability practices (akin to a ‘warrant of sustainability’) could set a benchmark and shape norms in the industry. Consistency among Aotearoa New Zealand’s seafood industry could strengthen the credibility of the whole industry, particularly on the international market. Iceland have taken a similar approach, developing a ‘country of origin’ eco-label based on the fact they believe they are viewed as a responsible fisheries country and wanted a way to verify this. A ‘statement of responsible fisheries in Iceland’ was co-developed by government and industry and all products have to undergo independent certification based on this statement to use the label. Maintaining independence in the assessment is important and having certification against a trusted, independent and transparent standard may also reduce the burden of responding to stock assessment information requests. Unless a mandatory programme is supported by the government, similar barriers to uptake from smaller players may occur.
- Demand for certified sustainable products is likely to increase. For now, it’s important for the Aotearoa New Zealand fisheries brand to be seen as sustainable and Fisheries New Zealand could play an important role in assisting the local industry to achieve certification across the board.[261] In the future, not meeting sustainability criteria may prohibit overseas market access, so it’s important for our fisheries exporters to stay ahead of these demands by achieving certification for globally recognised schemes.
- It’s important to strike a balance between consistency and monopolisation: Having similar standards is beneficial to know that the same stringency is applied across different products and may be helpful for people to be able to compare products. However, if one scheme takes over there is a chance that what constitutes ‘sustainability’ will be monopolised. This means any limitations, disadvantages or perverse incentives associated with that scheme will become widespread.[262]
Sustainability schemes are likely to play an important role in continuing to challenge Aotearoa New Zealand’s fisheries industry to improve their management practices. Achieving and maintaining certification of established but evolving standards can be used alongside government-driven management initiatives to achieve sustainable fisheries management.
Sustainability schemes must be credible to achieve these goals. Some fisheries that have been certified to schemes have received criticism for not adequately addressing fisheries impacts.[263, 264] These concerns can include bycatch and fisheries being certified that have not been scientifically assessed in decades, particularly in fisheries where fish are reportedly increasingly difficult to catch and fishers have not been reaching the TAC limits. For some fisheries, the level of investment needed can be very large. This issue is particularly pertinent for small, inshore fisheries which have significant data gaps that would need to be filled as a result of limited research spending allocation due to their small size.
References and footnotes
[1] Francis, R. I. C. C. et al. (2006) Links between climate and recruitment of New Zealand hoki (Macruronus novaezelandiae) now unclear, New Zealand Journal of Marine and Freshwater Research, 40(4), pp. 547–560.
[2] Bradford-Grieve, J. M. and Livingston, M. E. (2011) Spawning fisheries and the productivity of the marine environment off the west coast of the South Island, New Zealand, New Zealand aquatic environment and biodiversity Report No. 84.
[3] Input from Tā Tipene O’Regan.
[4] McGregor, V. L. et al. (2019) From data compilation to model validation: A comprehensive analysis of a full deep-sea ecosystem model of the Chatham Rise, PeerJ, 2019(2), pp. 1–42.
[5] Wiggins, A. et al. (2011) Mechanisms for data quality and validation in citizen science, in IEEE 7th International Conference on E-Science, e-Science 2011, Workshop Proceedings, Stockholm, Sweden.
[6] Bonter, D. N. and Cooper, C. B. (2012) Data validation in citizen science: A case study from Project FeederWatch, Frontiers in Ecology and the Environment, 10(6), pp. 305–307.
[7] Inhabiting the intermediate depths of the sea around 200-1,000 m.
[8] Flores, P. C. E. et al. (2020) Estimates of density of mesopelagic fish in the Southern Ocean derived from bulk acoustic data collected by ships of opportunity, Polar Biology, 43(1), pp. 43–61.
[9] Schmidt, J. O. et al. (2019) Future ocean observations to connect climate, fisheries and marine ecosystems, Frontiers in Marine Science, 6, pp. 1–18.
[10] Bradley, D. et al. (2019) Opportunities to improve fisheries management through innovative technology and advanced data systems, Fish and Fisheries, 20(3), pp. 564–583.
[11] van Helmond, A. T. M. et al. (2020) Electronic monitoring in fisheries: Lessons from global experiences and future opportunities, Fish and Fisheries, 21(1), pp. 162–189.
[12] NIWA is developing Fishecoviz in conjunction with Catalyst.
[13] DOC2.0 is being developed by NIWA.
[14] Malde, K. et al. (2019) Machine intelligence and the data-driven future of marine science, ICES Journal of Marine Science, 77(4), pp. 1274–1285.
[15] AI Forum of New Zealand (2018) Artificial intelligence: Shaping a future New Zealand.
[16] Wang, D. et al. (2020) Artificial intelligence-based multi-objective optimization protocol for protein structure refinement, Bioinformatics, 36(2), pp. 437–448.
[17] Berruti, F. et al. (2020) An executive primer on artificial general intelligence, McKinsey & Company.
[18] Hao, K. (2018) What is machine learning?, MIT Technology Review.
[19] Budek, K. and Osiński, B. (2018) What is reinforcement learning? The complete guide, deepsense.ai: Big Data Science.
[20] Hardesty, L. (2017) Explained: Neural networks, MIT News.
[21] LeCun, Y., Bengio, Y. and Hinton, G. (2015) Deep learning, Nature, pp. 436–444.
[22] Allken, V. et al. (2019) Machine learning to improve marine science for the sustainability of living ocean resources: Report from the 2019 Norway – US Workshop.
[23] Moen, E. et al. (2018) Automatic interpretation of otoliths using deep learning, PLoS One, 13(12), p. e0204713.
[24] Moore, B. R. et al. (2019) Feasibility of automating otolith ageing using CT scanning and machine learning.
[25] Michelin, M. et al. (2018) Catalyzing the growth of electronic monitoring in fisheries.
[26] Garcia, S. M. and Rosenberg, A. A. (2010) Food security and marine capture fisheries: Characteristics, trends, drivers and future perspectives, Philosophical Transactions of the Royal Society of London. Series B: Biological Sciences, 365(1554), pp. 2869–2880.
[27] Zhou, S. et al. (2019) Balanced harvest: Concept, policies, evidence, and management implications, Reviews in Fish Biology and Fisheries, 29(3), pp. 711–733.
[28] Hemmings, C. C. (1973) Direct observation of the behaviour of fish in relation to fishing gear, Helgoländer Wissenschaftliche Meeresuntersuchungen, 24(1–4), pp. 348–360.
[29] Pitcher, T. J. (1986) The behaviour of teleost fishes, Croom Helm London & Sydney.
[30] Takahashi, Y. and Komeyama, K. (2020) Simulation of the capture process in set net fishing using a fish-schooling behavior model, Fisheries Science, 86(6), 971-983.
[31] Putland, R. L. et al. (2017) Exploring spatial and temporal trends in the soundscape of an ecologically significant embayment, Scientific Reports, 7(1), pp. 1–12.
[32] Putland, R. L. et al. (2018) Vessel noise cuts down communication space for vocalizing fish and marine mammals, Global Change Biology, 24(4), pp. 1708–1721.
[33] Major, R. N. and Jeffs, A. G. (2018) Laboratory comparison of potential natural baits for potting New Zealand scampi (Metanephrops challenger), Bulletin of Marine Science, 94(3), pp. 635–655.
[34] van der Reis, A. L. et al. (2018) Preliminary analysis of New Zealand scampi (Metanephrops challengeri) diet using metabarcoding, PeerJ, 2018(9), pp. 1–25.
[35] Major, R. N. et al. (2017) Laboratory investigations of the foraging behaviour of New Zealand scampi, Journal of Experimental Marine Biology and Ecology, 497, pp. 99–106.
[36] Eayrs, S. et al. (2020) Mitigation techniques to reduce benthic impacts of trawling: MIT2019-02 A review for the Department of Conservation by Terra Moana Limited, pp. 1–59. Terra Moana.
[37] Tingley, G. (2014) An assessment of the potential for near-seabed midwater trawling to contact the seabed and to impact benthic habitat and vulnerable marine ecosystems (VMEs), SPRFMO 2nd Meeting of the Scientific Committee.
[38] Suuronen, P. (2005) Mortality of fish escaping trawl gears, FAO Fisheries Technical Paper.
[39] Wade, O. (2013) Trials of net modifications aboard the FV Nancy Glen II. Unpublished report prepared for Te Ohu Kaimoana.
[40] Hamilton, S. and Baker, G. B. (2015) Review of research and assessments on the efficacy of sea lion exclusion devices in reducing the incidental mortality of New Zealand sea lions (Phocarctos hookeri) in the Auckland Islands squid trawl fishery: Reply to Robertson (2015), Fisheries Research, pp. 130–132.
[41] Meyer, S. et al. (2017) Marine mammal population decline linked to obscured by-catch, Proceedings of the National Academy of Sciences of the United States of America, 114(44), pp. 11781–11786.
[42] McConnaughey, R. A. et al. (2020) Choosing best practices for managing impacts of trawl fishing on seabed habitats and biota, Fish and Fisheries, (21), pp. 319–337.
[43] Jones, E. G. (2015) Voyage Report (NAN1402): Trials if semi-pelagic trawl doors. Unpublished Report held in NIWA library.
[44] Herrmann et al. (2007) Understanding the size selectivity of redfish (Sebastes spp.) in North Atlantic trawl codends, Journal of Northwest Atlantic Fishery Science 44, p. 1-13.
[45] Peltorhamphus novaezeelandiae.
[46] Rhombosolea leporina.
[47] Rhombosolea plebeia.
[48] The use of net sonde cables, also known as ‘third wires’ (i.e. where a cable is hard wired to a trawl sonar attached to the net head rope to allow monitoring of the nest position and catch entering the net) have been prohibited in Aotearoa New Zealand waters by regulation since 2008 to prevent seabird mortalities because of observations that the third wire increased the risk of ‘warp strikes’, where seabirds run into the wire and are injured or killed (Acoura Marine, 2018). FNZ can (and has) grant special permits to trial gear with a third wire, with a requirement for observer coverage during trial.
[49] Þórðarson, G. and Viðarsson, J. R. (2014) Coastal fisheries in Iceland.
[50] See Desert Star Systems; Fiomarine; Edgetech; Smelts; Ashored.
[51] See ‘New ‘Pop-Up’ Fishing Gear Could Reduce Whale Entanglements’, SeaSense; ‘Shellfish sector tests innovative fishing gear to address concerns about mounting marine mammal deaths’.
[52] Dawson, S. M. et al. (2013) To ping or not to ping: The use of active acoustic devices in mitigating interactions between small cetaceans and gillnet fisheries, Endangered Species Research, 19, pp. 201–221.
[53] Omeyer, L. C. M. et al. (2020) Assessing the effects of banana pingers as a bycatch mitigation device for harbour porpoises (Phocoena phocoena), Frontiers in Marine Science, 7, pp. 1–10.
[54] Childerhouse, S. et al. (2020) Mit2019-01 review of dolphin acoustic deterrent device mitigation in inshore fisheries, Report No. 3507, Cawthron.
[55] Wang, J. H. et al. (2010) Developing visual deterrents to reduce sea turtle bycatch in gill net fisheries, Marine Ecology Progress Series, 408, pp. 241–250.
[56] Bielli, A. et al. (2020) An illuminating idea to reduce bycatch in the Peruvian small-scale gillnet fishery, Biological Conservation, 241, p. 108277.
[57] Field, R. et al. (2019) High contrast panels and lights do not reduce bird bycatch in Baltic Sea gillnet fisheries, Global Ecology and Conservation, 18, e00602.
[58] Pierre, J. P. and Norden, W. S. (2006) Reducing seabird bycatch in longline fisheries using a natural olfactory deterrent, Biological Conservation, 130(3), pp. 406–415.
[59] Wagner, C. M. et al. (2006) A field test verifies that pheromones can be useful for sea lamprey (Petromyzon marinus) control in the Great Lakes, Canadian Journal of Fisheries and Aquatic Sciences, 63(3), pp. 475–479.
[60] Johnson, N. S. et al. (2013) A synthesized mating pheromone component increases adult sea lamprey (Petromyzon marinus) trap capture in management scenarios, Canadian Journal of Fisheries and Aquatic Sciences, 70(7), pp. 1101–1108.
[61] Christiani, P. et al. (2019) Precision fisheries: Navigating a sea of troubles with advanced analytics.
[62] An Interview With Matts Johansen, Chief Executive Officer Of Aker BioMarine – Tech Company News.
[63] Chang, J. B. and Lee, Y. (2019) The effects of technological development on fisheries production, Fisheries Science, 85(1), pp. 259–269.
[64] Telesetsky, A. (2016) Fishing for the future: Addressing fisheries discards and increasing export value for New Zealand’s sustainable fisheries.
[65] Hilborn, R. et al. (2009) Can the data from at-sea observer surveys be used to make general inferences about catch composition and discards?, Canadian Journal of Fisheries and Aquatic Sciences, 66(12), pp. 2025–2039.
[66] Metacarcinus magister.
[67] Stanley et al. (2015) Design and implementation of electronic monitoring in the British Columbia groundfish hook and line fishery: A retrospective view of the ingredients of success. ICES Journal of Marine Science, 72(4), pp. 1230-1236.
[68] Australian Fisheries Management Authority (2020) Australian Fisheries Management Authority Electronic Monitoring Program.
[69] National Oceanic and Atmospheric Administration, US Department of Commerce and National Marine Fisheries Service (2016) Second National Electronic Monitoring Workshop, Seattle, WA.
[70] Sylvia, G. et al. (2016) Challenges, opportunities and costs of electronic fisheries monitoring. San Francisco.
[71] Mangi, S. C. et al. (2015) Approaches to fully documented fisheries: Practical issues and stakeholder perceptions, Fish and Fisheries, 16(3), pp. 426–452.
[72] Plet-Hansen, K. S. et al. (2017) Remote electronic monitoring and the landing obligation – some insights into fishers and fishery inspectors’ opinions, Marine Policy, 76, pp. 98–106.
[73] Nowlin, M. B. et al. (2019) Applying unoccupied aircraft systems to study human behavior in marine science and conservation programs, Frontiers in Marine Science, 6, p. 11.
[74] Pierre, J. P. (2018) Using electronic monitoring imagery to characterise protected species interactions with commercial fisheries: A primer and review.
[75] Awalludin, E. A. et al. (2020) A review on image processing techniques for fisheries application, Journal of Physics: Conference Series, 1529(5), p. 052031.
[76] Schoening, T. (2019) SHiPCC—A sea-going high-performance compute cluster for image analysis, Frontiers in Marine Science, 6, pp. 1–6.
[77] Wilcox, M. (2018) The future of fishing Is big data and artificial intelligence, Civil Eats.
[78] Ministry for Primary Industries (2020) Questions and answers: Further rollout of cameras on commercial fishing vessels.
[79] The Nature Conservancy and Gulf of Maine Research Institute (2017) Competition: N+1 fish, N+2 fish.
[80] Dawkins, M. et al. (2017) An open-source platform for underwater image and video analytics, in 2017 IEEE Winter Conference on Applications of Computer Vision (WACV), Santa Rosa, CA, pp. 898–906.
[81] Ovenden, J. (1990) Mitochondrial DNA and marine stock assessment: A review, Marine and Freshwater Research, 41(6), p. 835.
[82] Bernatchez, L. et al. (2017) Harnessing the power of genomics to secure the future of seafood, Trends in Ecology and Evolution, 32(9), pp. 665–680.
[83] Casey, J. et al. (2016) The role of genetics in fisheries management under the E.U. common fisheries policy, Journal of Fish Biology, 89(6), pp. 2755–2767.
[84] Mace, P. et al. (2020) Report of the workshop on the utility of genetic analyses for addressing New Zealand fisheries questions, New Zealand Fisheries Science Review 2020/01.
[85] Tuck, I. D. et al. (2014) Ecosystem and Environmental Indicators for Deepwater Fisheries, New Zealand Aquatic Environment and Biodiversity Report No. 127.
[86] Symonds, J. et al. (2018) New Zealand aquaculture selective breeding: From theory to industry application for three flagship species, Proceedings of the World Congress on Genetics applied to Livestock Production, 11(11), p. 1035.
[87] Verry, A. J. F. et al. (2020) Genetic structure and recent population expansion in the commercially harvested deep-sea decapod, Metanephrops challengeri (Crustacea: Decapoda), New Zealand Journal of Marine and Freshwater Research, 54:2, 251-270.
[88] Bernatchez, L. and Wellenreuther, M. (2018) Synergistic integration of genomics and ecoevolutionary dynamics for sustainable fisheries: A Reply to Kuparinen and Uusi-Heikkilä, Trends in Ecology and Evolution, 33(5), pp. 308–310.
[89] Heino, M. et al. (2015) Fisheries-induced evolution, Annual Review of Ecology, Evolution, and Systematics, 46, pp. 461–480.
[90] Dahle, G. et al. (2018) Genetic management of mixed-stock fisheries “real-time”: The case of the largest remaining cod fishery operating in the Atlantic in 2007–2017, Fisheries Research, 205, pp. 77–85.
[91] Rhincodon typus.
[92] Mobula mobular.
[93] Carcharhinus longimanus.
[94] Cetorhinus maximus.
[95] Tursiops truncatus.
[96] Monteiro, N. M. et al. (2014) Validating the use of colouration patterns for individual recognition in the worm pipefish using a novel set of microsatellite markers, Molecular Ecology Resources, 14(1), pp. 150–156.
[97] Le Vin, A. L. et al. (2011) Validation of swabs as a non-destructive and relatively non-invasive DNA sampling method in fish, Molecular Ecology Resources, 11(1), pp. 107–109.
[98] Tzadik, O. E. et al. (2017) Chemical archives in fishes beyond otoliths: A review on the use of other body parts as chronological recorders of microchemical constituents for expanding interpretations of environmental, ecological, and life-history changes, Limnology and Oceanography: Methods, 15(3), pp. 238–263.
[99] Kalish, J. M. (1989) Otolith microchemistry: Validation of the effects of physiology, age and environment on otolith composition, Journal of Experimental Marine Biology and Ecology, 132(3), pp. 151–178.
[100] Rowell, K. et al. (2010) Nitrogen isotopes in otoliths reconstruct ancient trophic position, Environmental Biology of Fishes, 89(3), pp. 415–425.
[101] Tanner, S. E. et al. (2016) Otolith chemistry in stock delineation: A brief overview, current challenges and future prospects, Fisheries Research, 173, pp. 206–213.
[102] Salmo salar.
[103] Kennedy, B. P. et al. (2002) Reconstructing the lives of fish using Sr isotopes in otoliths, Canadian Journal of Fisheries and Aquatic Sciences, 59(6), pp. 925–929.
[104] Norrie, C. et al. (2020) Spill-over from aquaculture may provide a larval subsidy for the restoration of mussel reefs, Aquaculture Environment Interactions, 12, pp. 231–249.
[105] Fenton, G. E. et al. (1991) Age determination of orange roughy, Hoplostethus atlanticus (Pisces: Trachichthyidae) using 210Pb:226Ra disequilibria, Marine Biology, 109(2), pp. 197–202.
[106] McMahon, K. W. et al. (2011) A new method to reconstruct fish diet and movement patterns from δ13C values in otolith amino acids, Canadian Journal of Fisheries and Aquatic Sciences, 68(8), pp. 1330–1340.
[107] Walther, B. D. (2019) The art of otolith chemistry: Interpreting patterns by integrating perspectives, Marine and Freshwater Research, 70(12).
[108] Mace, P. M. et al. (2014) The evolution of New Zealand’s fisheries science and management systems under ITQs, ICES Journal of Marine Science, 71(2), pp. 204–215.
[109] Ryan, T. E. and Kloser, R. J. (2016) Improved estimates of orange roughy biomass using an acoustic-optical system in commercial trawlnets, ICES Journal of Marine Science, 73(8), pp. 2112–2124.
[110] Luczkovich, J. J. et al. (2008) Passive acoustics as a tool in fisheries science, Transactions of the American Fisheries Society, 137(2), pp. 533–541.
[111] Fisheries New Zealand (2020) Medium term research plan for Deepwater Fisheries, Fisheries New Zealand Information Paper No: 2020/01.
[112] Rowell, T. J. et al. (2019) Advanced acoustic technologies for the monitoring and management of sustainable fisheries: A practice manual.
[113] Ryan, T. E. et al. (2009) Measurement and visual verification of fish target strength using an acoustic-optical system attached to a trawlnet, ICES Journal of Marine Science, 66(6), pp. 1238–1244.
[114] O’Driscoll, R. L. et al. (2016) Industry acoustic surveys of spawning southern blue whiting on the Bounty Platform, New Zealand, Fisheries Research, 178, pp. 61–70.
[115] Rountree, R. A. et al. (2006) Listening to Fish, Fisheries, 31(9), pp. 433–446.
[116] Escobar-Flores, P. et al. (2019) Acoustic assessment of the micronekton community on the Chatham Rise, New Zealand, using a semi-automated approach, Frontiers in Marine Science, 6(507).
[117] Acoustic target strength is the amount of sound scattered by an individual fish and is the denominator in the equation used to estimate fish density (i.e. the total amount of sound scattering attributed to the species is divided by the target strength to calculate density).
[118] Compson, Z. G. et al. (2020) Metabarcoding from microbes to mammals: Comprehensive bioassessment on a global scale, Frontiers in Ecology and Evolution, 8, pp. 1–19.
[119] Bicknell, A. W. J. et al. (2016) Camera technology for monitoring marine biodiversity and human impact, Frontiers in Ecology and the Environment, 14(8), pp. 424–432.
[120] Lambert, G. I. et al. (2014) Quantifying recovery rates and resilience of seabed habitats impacted by bottom fishing, Journal of Applied Ecology, 51(5), pp. 1326–1336.
[121] Williams, K. et al. (2014) An underwater stereo-camera trap, Methods in Oceanography, 11, pp. 1–12.
[122] Whitmarsh, S. K. et al. (2017) What is Big BRUVver up to? Methods and uses of baited underwater video, Reviews in Fish Biology and Fisheries, pp. 53–73.
[123] Thomson, J. A. et al. (2015) Extreme temperatures, foundation species, and abrupt ecosystem change: an example from an iconic seagrass ecosystem, Global Change Biology, 21(4), pp. 1463–1474.
[124] Rosen, S. and Holst, J. C. (2013) DeepVision in-trawl imaging: Sampling the water column in four dimensions, Fisheries Research, 148, pp. 64–73.
[125] Jaiteh, V. F. et al. (2014) Combining in-trawl video with observer coverage improves understanding of protected and vulnerable species by-catch in trawl fisheries, Marine and Freshwater Research, 65(9), pp. 830–837.
[126] Bryan, D. R. et al. (2014) Quantitative video analysis of flatfish herding behavior and impact on effective area swept of a survey trawl, Fisheries Research, 154, pp. 120–126.
[127] Liggins, L. et al. (2020) Natural history footage provides new reef fish biodiversity information for a pristine but rarely visited archipelago, Scientific Reports, 10(1), p. 3159.
[128] Nyegaard, M. (2018) There be giants! The importance of taxonomic clarity of the large ocean sunfishes (genus Mola, Family Molidae) for assessing sunfish vulnerability to anthropogenic pressures. A thesis submitted for the degree of Doctor of Philosophy of Veterinary and Life Sciences, Murdoch University, Perth, Australia.
[129] Salter, I. et al. (2019) Environmental DNA concentrations are correlated with regional biomass of Atlantic cod in oceanic waters, Communications Biology, 2(1), pp. 1–9.
[130] Lacoursière‐Roussel, A. et al. (2016) Quantifying relative fish abundance with eDNA a promising tool for fisheries management, Journal of Applied Ecology, 53, pp. 1148–1157.
[131] Knudsen, S. W. et al. (2019) Species-specific detection and quantification of environmental DNA from marine fishes in the Baltic Sea, Journal of Experimental Marine Biology and Ecology, 510, pp. 31–45.
[132] Ficetola, G. F. et al. (2008) Species detection using environmental DNA from water samples, Biology Letters, 4(4), pp. 423–425.
[133] Zaiko, A. et al. (2018) Advantages and limitations of environmental DNA/RNA tools for marine biosecurity: Management and surveillance of non-indigenous species, Frontiers in Marine Science, 5.
[134] Stat, M. et al. (2017) Ecosystem biomonitoring with eDNA: Metabarcoding across the tree of life in a tropical marine environment, Scientific Reports, 7(1), pp. 1–11.
[135] Stoeckle, M. Y. et al. (2020) Trawl and eDNA assessment of marine fish diversity, seasonality, and relative abundance in coastal New Jersey, ICES Journal of Marine Science.
[136] DiBattista, J. D. et al. (2020) Environmental DNA can act as a biodiversity barometer of anthropogenic pressures in coastal ecosystems, Scientific Reports, 10(1), pp. 1–15.
[137] Nester, G. M. et al. (2020) Development and evaluation of fish eDNA metabarcoding assays facilitate the detection of cryptic seahorse taxa (family: Syngnathidae), Environmental DNA, 2(4), pp. 614–626.
[138] Bakker, J. et al. (2017) Environmental DNA reveals tropical shark diversity in contrasting levels of anthropogenic impact, Scientific Reports, 7(1), pp. 1–11.
[139] Hansen, B. K. et al. (2018) The sceptical optimist: Challenges and perspectives for the application of environmental DNA in marine fisheries, Fish and Fisheries, 19(5), pp. 751–768.
[140] Andres, K. J. et al. (2021) Nuclear eDNA estimates population allele frequencies and abundance in experimental mesocosms and field samples, Molecular Ecology, pp. 1–13.
[141] Berry, O. et al. (2020) Making environmental DNA (eDNA) biodiversity records globally accessible, Environmental DNA, pp. 1–7.
[142] Berry, T. E. et al. (2019) Marine environmental DNA biomonitoring reveals seasonal patterns in biodiversity and identifies ecosystem responses to anomalous climatic events, PLoS Genetics, 15(2), pp. 1–19.
[143] Jarman, S. N. et al. (2018) The value of environmental DNA biobanking for long-term biomonitoring, Nature Ecology and Evolution, 2(8), pp. 1192–1193.
[144] Fulton, E. A. et al. (2011) Lessons in modelling and management of marine ecosystems: The Atlantis experience, Fish and Fisheries, 12(2), pp. 171–188.
[145] Plaganyi, E. E. (2007) Models for an ecosystem approach to fisheries, FAO Fisheries Technical Paper 477.
[146] Doonan, I. et al. (2016) Casal2: New Zealand’s integrated population modelling tool, Fisheries Research, 183, pp. 498–505.
[147] Collie, J. S. et al. (2016) Ecosystem models for fisheries management: Finding the sweet spot, Fish and Fisheries, 17(1), pp. 101–125.
[148] Bradford-Grieve, J. M. et al. (2003) Pilot trophic model for subantarctic water over the Southern Plateau, New Zealand: A low biomass, high transfer efficiency system, Journal of Experimental Marine Biology and Ecology, pp. 223–262.
[149] Pauly, D. et al. (2000) Ecopath, Ecosim, and Ecospace as tools for evaluating ecosystem impact of fisheries, ICES Journal of Marine Science, 57(3), pp. 697–706.
[150] Pinkerton, M. H. (2011) A balanced trophic model of the Chatham Rise, New Zealand. NIWA. Wellington, New Zealand.
[151] Pinkerton, M. H. et al. (2015) Changes to the food-web of the Hauraki Gulf during the period of human occupation: A mass-balance model approach, New Zealand Aquatic Environment and Biodiversity Report No. 160.
[152] Pinkerton, M. H. et al. (2008) Trophic modelling of a New Zealand rocky reef ecosystem using simultaneous adjustment of diet, biomass and energetic parameters, Journal of Experimental Marine Biology and Ecology, pp. 189–203.
[153] Pinkerton, M. H. (2010) A balanced model of the food web of the Ross Sea, Antarctica, Commission for the Conservation of Antarctic Marine Living Resources Science, 17, pp. 1–31.
[154] Law, R. et al. (2009) Size-spectra dynamics from stochastic predation and growth of individuals, Ecology, 90(3), pp. 802–811.
[155] Blanchard, J. L. et al. (2014) Evaluating targets and trade-offs among fisheries and conservation objectives using a multispecies size spectrum model, Journal of Applied Ecology, 51, pp. 612–622.
[156] Blanchard, J. L. et al. (2009) How does abundance scale with body size in coupled size-structured food webs?, Journal of Animal Ecology, 78(1), pp. 270–280.
[157] Rogers, A. et al. (2014) Vulnerability of coral reef fisheries to a loss of structural complexity, Current Biology, 24(9), pp. 1000–1005.
[158] Blanchard, J. L. et al. (2012) Potential consequences of climate change for primary production and fish production in large marine ecosystems, Philosophical Transactions of the Royal Society B: Biological Sciences, 367(1605), pp. 2979–2989.
[159] Rogers, A. et al. (2018) Fisheries productivity under progressive coral reef degradation, Journal of Applied Ecology, 55(3), pp. 1041–1049.
[160] McGregor, V. L. et al. (2019) Spawning stock recruitment creates misleading dynamics under predation release in ecosystem and multi-species models, PeerJ, 2019(7).
[161] Andersen, K. H. (2020) Size-based theory for fisheries advice, ICES Journal of Marine Science, 77(7-8), 2445-2455.
[162] Jacobsen, N. S. et al. (2016) Comparing model predictions for ecosystem-based management, Canadian Journal of Fisheries and Aquatic Sciences, 73(4), pp. 666–676.
[163] Petrik, C. M. et al. (2019) Bottom-up drivers of global patterns of demersal, forage, and pelagic fishes, Progress in Oceanography, 176, p. 102124.
[164] Azevedo C. et al. (2020) Evaluation of four global ocean reanalysis products for New Zealand waters – A guide for regional ocean modelling, New Zealand Journal of Marine and Freshwater Research, pp. 1–24.
[165] Maunder, M. N. and Piner, K. R. (2015) Contemporary fisheries stock assessment: Many issues still remain, ICES Journal of Marine Science, 71(1), pp. 7-18.
[166] Glemarec, G. et al. (2020) Assessing seabird bycatch in gillnet fisheries using electronic monitoring, Biological Conservation, 243, p. 108461.
[167] FAO (2009) International guidelines for the management of Deep-Sea fisheries in the high seas.
[168] Letessier, T. B. et al. (2017) Sampling mobile oceanic fishes and sharks: Implications for fisheries and conservation planning, Biological Reviews, 92(2), pp. 627–646.
[169] Abraham, E. R. and Thompson, F. N. (2011) Estimated capture of seabirds in New Zealand trawl and longline fisheries, 2002–03 to 2008–09, New Zealand Aquatic Environment and Biodiversity Report No. 79.
[170] Guerra, A. S. et al. (2020) Fisheries-induced selection against schooling behaviour in marine fishes: FIE and schooling behaviour, Proceedings of the Royal Society B: Biological Sciences, 287(1935).
[171] Ghaffarivardavagh, R. et al. (2020) Underwater backscatter localization: Toward a battery-free underwater GPS, HotNets ’20, November 4–6, 2020, Virtual Event, USA.
[172] Schiel, D. R. et al. (2019) The Kaikōura earthquake in southern New Zealand: Loss of connectivity of marine communities and the necessity of a cross-ecosystem perspective, Aquatic Conservation: Marine and Freshwater Ecosystems, 29(9), pp. 1520–1534.
[173] Smale, D. A. et al. (2012) Regional-scale benthic monitoring for ecosystem-based fisheries management (EBFM) using an autonomous underwater vehicle (AUV), ICES Journal of Marine Science, pp. 1108–1118.
[174] Arctocephalus gazelle.
[175] Hydrurga leptonyx.
[176] Halichoerus grypus and Phoca vitulina.
[177] Goebel, M. E. et al. (2015) A small unmanned aerial system for estimating abundance and size of Antarctic predators, Polar Biology, 38(5), pp. 619–630.
[178] Pomeroy, P. et al. (2015) Assessing use of and reaction to unmanned aerial systems in gray and harbor seals during breeding and molt in the UK, Journal of Unmanned Vehicle Systems, 3(3), pp. 102–113.
[179] Eubalaena australis.
[180] Christiansen, F. et al. (2018) Maternal body size and condition determine calf growth rates in southern right whales, Marine Ecology Progress Series, 592, pp. 267–281.
[181] Shelley, A. and Andrews, H. (2015) Economic benefits to New Zealand from beyond-line-of-sight operation of UAVs, p. 69.
[182] Civil Aviation Authority New Zealand (2015) Part 102: CAA consolidation. Unmanned aircraft operator certification, Civil Aviation Rules.
[183] M.E. Consulting (2019) Drones: Benefits study, High level findings.
[184] Acanthaster planci.
[185] Dayoub, F. et al. (2015) Robotic detection and tracking of Crown-of-Thorns starfish, in IEEE/RSJ International Conference on Intelligent Robots and Systems (IROS), pp. 1921–1928.
[186] Yang, J. et al. (2013) The role of satellite remote sensing in climate change studies, Nature Climate Change, 3(10), pp. 875–883.
[187] Hussey, N. E. et al. (2015) Aquatic animal telemetry: A panoramic window into the underwater world, Science, 348(6240), p. 1255642.
[188] Wilson, S. G. et al. (2005) Movements of bluefin tuna (Thunnus thynnus) in the northwestern Atlantic Ocean recorded by pop-up satellite archival tags, Marine Biology, 146(2), pp. 409–423.
[189] Megaptera novaeangliae.
[190] Mysticeti.
[191] Riekkola, L. et al. (2018) Application of a multi-disciplinary approach to reveal population structure and Southern Ocean feeding grounds of humpback whales, Ecological Indicators, 89, pp. 455–465.
[192] Riekkola, L. et al. (2019) Environmental drivers of humpback whale foraging behavior in the remote Southern Ocean, Journal of Experimental Marine Biology and Ecology, 517, pp. 1–12.
[193] Carroll, E. (2020) Genome and satellite technology reveal recovery rates and impacts of climate change on southern right whales, The Conversation.
[194] Williamson, M. J. et al. (2019) Satellite remote sensing in shark and ray ecology, conservation and management, Frontiers in Marine Science, 6, pp. 1–23.
[195] Bradley, D. et al. (2019) Leveraging satellite technology to create true shark sanctuaries, Conservation Letters, 12(2), pp. 1–8.
[196] Block, B. A. et al. (2011) Tracking apex marine predator movements in a dynamic ocean, Nature, 475(7354), pp. 86–90.
[197] Orcinus orca.
[198] Forage fish of many different species from the Clupeidae family.
[199] Mul, E. et al. (2020) Killer whales are attracted to herring fishing vessels, Marine Ecology Progress Series, 652, pp. 1–13.
[200] McElderry, H. et al. (2008) Electronic monitoring to assess protected species interactions in New Zealand longline fisheries: A pilot study.
[201] McElderry, H. et al. (2011) Electronic monitoring in the New Zealand inshore trawl fishery: A pilot study.
[202] McElderry, H. et al. (2007) Pilot study to test the effectiveness of electronic monitoring in Canterbury fisheries, Wellington, New Zealand.
[203] Pria, M. J. et al. (2014) Using electronic monitoring to document inshore set net captures of Hector’s dolphins.
[204] Tremblay-Boyer, L. and Abraham, E. R. (2020) Increased fisher-reporting of seabird captures during an electronic-monitoring trial, New Zealand Aquatic Environment and Biodiversity Report No. 238.
[205] Department of Conservation and Fisheries New Zealand (2019) National plan of action – Seabirds 2020: Supporting document.
[206] Globicephala macrorhynchus and Globicephala melas.
[207] Thorne, L. H. et al. (2019) Predicting fisheries bycatch: A case study and field test for pilot whales in a pelagic longline fishery, Diversity and Distributions, 25(6), pp. 909–923.
[208] Luck, C. et al. (2020) Drivers of spatiotemporal variability in bycatch of a top marine predator: First evidence for the role of water turbidity in protected species bycatch, Journal of Applied Ecology, pp. 219–228.
[209] Ministry for Primary Industries (2020) Aquatic environment and biodiversity annual review 2019-20, p. 724.
[210] Roberts, J. O. et al. (2019) Spatial risk assessment of threats to Hector’s and Māui dolphins (Cephalorhynchus hectori), New Zealand Aquatic Environment and Biodiversity Report No. 214.
[211] Ball, I. et al. (2009) Marxan and relatives: Software for spatial conservation prioritization, In: Spatial conservation prioritization: Quantitative methods and computational tools, Oxford University Press, pp. 185–195.
[212] Lehtomäki, J. and Moilanen, A. (2013) Methods and workflow for spatial conservation prioritization using Zonation, Environmental Modelling and Software, 47, pp. 128–137.
[213] Stephenson, F. et al. (2020) Modelling the spatial distribution of cetaceans in New Zealand waters, Diversity and Distributions, 26(4), pp. 495–516.
[214] Georgian, S. E. et al. (2019) Ensemble habitat suitability modeling of vulnerable marine ecosystem indicator taxa to inform deep-sea fisheries management in the South Pacific Ocean, Fisheries Research, pp. 256–274.
[215] Senina, I. et al. (2020) Integrating tagging and fisheries data into a spatial population dynamics model to improve its predictive skills, Canadian Journal of Fisheries and Aquatic Sciences, 77(3), pp. 576–593.
[216] Dissostichus mawsoni.
[217] Mormede, S. et al. (2017) Using spatial population models to investigate the potential effects of the Ross Sea region Marine Protected Area on the Antarctic toothfish population, Fisheries Research, 190 pp. 164-174.
[218] Eubalaena glacialis.
[219] Pollachius spp.
[220] Toonen, H. M. and Bush, S. R. (2018) The digital frontiers of fisheries governance: Fish attraction devices, drones and satellites, Journal of Environmental Policy and Planning, pp. 1–13.
[221] McCauley, D. J. et al. (2016) Ending hide and seek at sea, Science, 351(6278), pp. 114 – 1150.
[222] Rowlands, G. et al. (2019) Satellite surveillance of fishing vessel activity in the Ascension Island Exclusive Economic Zone and Marine Protected Area, Marine Policy, 101, pp. 39–50.
[223] Park, J. et al. (2020) Illuminating dark fishing fleets in North Korea, Science Advances, 6(30), pp. 1–8.
[224] Hare, S. R. et al. (2015) Verifying FAD-association in purse seine catches on the basis of catch sampling, Fisheries Research, 172, pp. 361–372.
[225] Pinkerton, M. H. et al. (2019) Satellite indicators of phytoplankton and ocean surface temperature for New Zealand, NIWA Client Report No: 2018180WN.
[226] Ministry for the Environment and Stats NZ (2019) New Zealand’s Environmental Reporting Series: Our Marine Environment 2019.
[227] Vance, J. M. et al. (2020) NZOA-ON: The New Zealand Ocean Acidification Observing Network, Marine and Freshwater Research, 71(3), pp. 281–299.
[228] Pinkerton, M. H. et al. (2020) Zooplankton in the Southern Ocean from the continuous plankton recorder: Distributions and long-term change, Deep-Sea Research Part I: Oceanographic Research Papers, 162.
[229] Ohman, M. D. et al. (2019) Zooglider: An autonomous vehicle for optical and acoustic sensing of zooplankton, Limnology and Oceanography: Methods, 17(1), pp. 69–86.
[230] Lombard, F. et al. (2019) Globally consistent quantitative observations of planktonic ecosystems, Frontiers in Marine Science, 6, pp. 1-1.
[231] Hosie, G. W. et al. (2003) Development of the Southern Ocean continuous plankton recorder survey, Progress in Oceanography, 57(2–4), pp. 263–283.
[232] Zaiko, A. et al. (2016) Metabarcoding improves detection of eukaryotes from early biofouling communities: Implications for pest monitoring and pathway management, Biofouling, 32(6), pp. 671-684.
[233] Zaiko, A. et al. (2020) Elucidating biodiversity shifts in ballast water tanks during a cross-latitudinal transfer: Complementary insights from molecular analyses, Environmental Science and Technology, 54(13), pp. 8443-8454.
[234] Juanes, F. (2018) Visual and acoustic sensors for early detection of biological invasions: Current uses and future potential, Journal for Nature Conservation, 42, pp. 7-11.
[235] Brekke, C. and Solberg, A. (2005) Oil spill detection by satellite remote sensing, Remote Sensing of Environment, 95(1), pp. 1-13.
[236] IOC (2019) The global ocean observing system 2030 strategy, IOC Brochure, 2019.
[237] Moltmann, T. et al. (2019) A Global Ocean Observing System (GOOS), delivered through enhanced collaboration across regions, communities, and new technologies, Frontiers in Marine Science, 6, pp. 1–21.
[238] Gislason, H. et al. (2000) Symposium overview: Incorporating ecosystem objectives within fisheries management, ICES Journal of Marine Science, 57(3), pp. 468–475.
[239] Summerhayes, C. (2002) Technical tools for regional seas management: The role of the global ocean observing system (GOOS), Ocean and Coastal Management, 45(11–12), pp. 777–796.
[240] Lee, W. C. and Viswanathan, K. K. (2019) Trade and sustainability of ASEAN fisheries resources, Journal of Economics and Sustainability, 1(1), pp. 33–45.
[241] O’Callaghan, J. et al. (2019) Developing an integrated ocean observing system for New Zealand, Frontiers in Marine Science, 6, pp. 1–7.
[242] Sigfusson, T. (2019) The New Fish Wave, Leete’s Island Books.
[243] den Hollander, N. and Thorsteinsson, T. (2020) Driving innovation in the Icelandic seafood industry: A case study of the Iceland Ocean Cluster, KTH Royal Institute of Technology, Stockholm, Sweden.
[244] New Zealand Government (2019) Economic Plan for a productive, sustainable and inclusive economy, p. 28.
[245] Secretariat of the Pacific Community (2014) Adding value to fish processing by-products – SPC Policy Brief 21/2014.
[246] Nawaz, A. et al. (2020) Valorization of fisheries by-products: Challenges and technical concerns to food industry, Trends in Food Science and Technology, 99, pp. 34–43.
[247] Hosch, G. (2019) The 2018 Atlantic Bluefin Tuna Trade Scandal: The catch & trade control framework of ICCAT and how to fix it, Europe, pp. 65.
[248] Lewis, S. G. and Boyle, M. (2017) The expanding role of traceability in seafood: Tools and key initiatives, Journal of Food Science, 82, pp. A13–A21.
[249] Hosch, G. and Blaha, F. (2017) Seafood traceability for fisheries compliance: Country-level support for catch documentation schemes, FAO Fisheries and Aquaculture Technical Paper 619.
[250] Rodriguez-Salvador, B. and Dopico, D. C. (2020) Understanding the value of traceability of fishery products from a consumer perspective, Food Control, 112, p. 107142.
[251] OpenSeas (2019) Traceability section detail report, p. 8.
[252] Hardt, M. J. et al. (2017) Current barriers to large-scale interoperability of traceability technology in the seafood sector, Journal of Food Science, 82, pp. A3–A12.
[253] Roberson, L. A. et al. (2020) Over 90 endangered fish and invertebrates are caught in industrial fisheries, Nature Communications, 11(1), pp. 1–8.
[254] Ogden, R. (2008) Fisheries forensics: The use of DNA tools for improving compliance, traceability and enforcement in the fishing industry, Fish and Fisheries, 9(4), pp. 462–472.
[255] Martinsohn, J. T. et al. (2018) DNA-analysis to monitor fisheries and aquaculture: Too costly?, Fish and Fisheries, pp. 391–401.
[256] Blomquist, J. et al. (2020) Price premiums for eco-labelled seafood: Effects of the MSC certification suspension in the Baltic Sea cod fishery, European Review of Agricultural Economics, 47(1), pp. 50–70.
[257] Van Putten, I. et al. (2020) Shifting focus: The impacts of sustainable seafood certification, PLoS One, 15(5), pp. 1–24.
[258] Washington, S. and Ababouch, L. (2011) Private standards and certification in fisheries and aquaculture: Current practice and emerging issues, FAO Fisheries and Aquaculture Technical Paper.
[259] Pinkerton, M.H. (2010) Headline indicators for the New Zealand Ocean, NIWA, Wellington, New Zealand.
[260] Bellchambers, L. M. et al. (2016) Adopting Marine Stewardship Council certification of Western Australian fisheries at a jurisdictional level: The benefits and challenges, Fisheries Research, 183, pp. 609–616.
[261] Fisheries New Zealand (2018) Aquatic environment and biodiversity annual review 2018: A summary of environmental interactions between the seafood sector and the aquatic environment.
[262] Hadjimichael, M. and Hegland, T. J. (2016) Really sustainable? Inherent risks of eco-labeling in fisheries, Fisheries Research, 174, pp. 129–135.
[263] Jacquet, J. et al. (2010) Seafood stewardship in crisis, Nature, 467(7311), pp. 28–29.
[264] Christian, C. et al. (2013) A review of formal objections to Marine Stewardship Council fisheries certifications, Biological Conservation, 161, pp. 10–17.